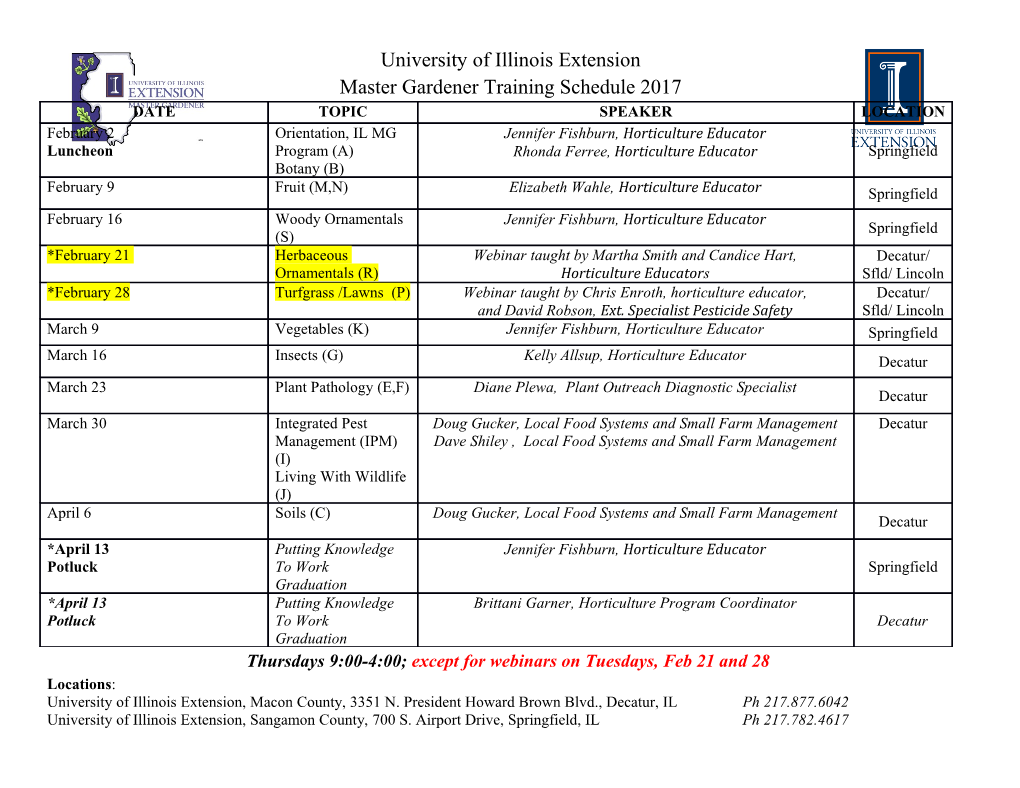
BÐack¹b ÓÜ ÅÓ deÐ× fÖÓÑ ÁÒÔÙØ¹ÓÙØÔÙØ Åea×ÙÖeÑeÒØ× ÄeÒÒaÖØ ÄjÙÒg DeÔaÖØÑeÒØ Óf EÐecØÖicaÐ EÒgiÒeeÖiÒg ÄiÒkÓÔiÒg ÍÒiÚeÖ×iØÝ¸ ËE¹58½ 8¿ ÄiÒkÓÔiÒg¸ ËÛedeÒ ÏÏÏ: hØØÔ:»»ÛÛÛºcÓÒØÖÓкi×ݺРiÙº ×e EÑaiÐ: ÐjÙÒg@i×ݺÐiÙº×e ÇcØÓb eÖ ¾¸ ¾¼¼½ ERTEK REGL NIK A UT OL OMATIC CONTR LINKÖPING ÊeÔ ÓÖØ ÒÓº: ÄiÌÀ¹ÁËY¹Ê¹¾¿6¾ FÓÖ Øhe ½8Øh ÁEEE ÁÒרÖÙÑeÒØaØiÓÒ aÒd Åea×ÙÖeÑeÒØ ÌechÒÓÐÓgÝ CÓÒfeÖeÒce¸ BÙdaÔ eר¸ ¾¼¼½ ÌechÒicaÐ ÖeÔ ÓÖØ× fÖÓÑ Øhe AÙØÓÑaØic CÓÒØÖÓÐ gÖÓÙÔ iÒ ÄiÒkÓÔiÒg aÖe aÚaiÐabÐe bÝ aÒÓÒÝÑÓÙ× fØÔ aØ Øhe addÖe×× fØÔºcÓÒØÖÓкi×ݺÐiÙº×eº Ìhi× ÖeÔ ÓÖØ i× cÓÒØaiÒed iÒ Øhe ¬Ðe ¾¿6¾ºÔdfº IEEE Instrumentation and Measurement Technology Conference Budapest, Hungary, May 21–23, 2001 Black-box Models from Input-output Measurements Lennart Ljung Div. of Automatic Control Link¨oping University SE-58183 Link¨oping, Sweden email: [email protected] Abstract – A black-box model of a system is one that does not use any par- “successful in the past”. ticular prior knowledge of the character or physics of the relationships in- volved. It is therefore more a question of ”curve- fitting” than ”modeling”. This paper deals with Black-box models for dynamical sys- In this presentation several examples of such black-box model structures will be given. Both linear and non-linear structures are treated. Relation- tems, for which inputs and outputs can be measured. We shall ships between linear models, fuzzy models, neural networks and classical both deal with linear and non-linear systems. non-parametric models are discussed. Some reasons for the usefulness of these model types will also be given. First, in Section II we list the basic features of black-box mod- eling in terms of a simple example. In Section III we outline Ways to fit black box structures to measured input-output data are described, as well as the more fundamental (statistical) properties of the resulting mod- the basic estimation techniques used. Section IV deals with els. the basic trade-offs to decide the size of the structure used (es- sentially how many parameters are to be used: the “fineness of I. INTRODUCTION: MODELS OF DIFFERENT COLORS approximation”). So far the discussion is quite independent of the particular structure used. In Section V we turn to particu- At the heart of any estimation problem is to select a suitable lar examples of linear black-box models and to issues how to model structure. A model structure is a parameterized family choose between the possibilities, while VI similarly deals with of candidate models of some sort, within which the search for non-linear black-box models for dynamical systems. a model is conducted. II. BLACK-BOX MODELS: BASIC FEATURES A basic rule in estimation is not to estimate what you already know. In other words, one should utilize prior knowledge and To bring out the basic features of a black-box estimation prob- physical insight about the system when selecting the model lem, let us study a simple example. Suppose the problem is g ´Üµ; ½ Ü ½ structure. It is customary to color-code – in shades of grey – to estimate an unknown function ¼ . The ob- Ý ´k µ Ü the model structure according to what type of prior knowledge servations we have are noise measurements at points k has been used: which we may or may not choose ourselves: Ý ´k µ=g ´Ü µ·e´k µ ¼ k ¯ White-box models: This is the case when a model is per- (1) fectly known; it has been possible to construct it entirely from prior knowledge and physical insight. How to approach this problem? One way or another we must decide “where to look for” g . We could, e.g., have the infor- ¯ Grey-box models: This is the case when some physical insight is available, but several parameters remain to be mation that g is a third order polynomial. This would lead to determined from observed data. It is useful to consider the – in this case – grey box model structure ¾ ½ two sub-cases: Ò g ´Ü; µ= · Ü · Ü · ::: · Ü ½ ¾ ¿ Ò (2) – Physical Modeling: A model structure can be built on physical grounds, which has a certain number of pa- =4 with Ò , and we would estimate the parameter vector rameters to be estimated from data. This could, e.g., be from the observations Ý , using e.g. the classical least squares a state space model of given order and structure. method. – Semi-physical modeling: Physical insight is used to sug- gest certain nonlinear combinations of measured data Now suppose that we have no structural information at all signal. These new signals are then subjected to model about g .8/01/$10.00 We would then©2001 still IEEE have to assume something about structures of black box character. it, e.g. it is an analytical function, or that it is piecewise con- ¯ Black-box models: No physical insight is available or stant or something like that. In this situation, we could still use used, but the chosen model structure belongs to fami- (2), but now as black-box model: if we assume g to be ana- lies that are known to have good flexibility and have been lytic we know that it can be approximated arbitrarily well by a 0-7803-6646- polynomial. The necessary order Ò would not be known, and We shall also generally denote all measurements available up we would have to find a good value of it using some suitable to time Æ by scheme. Æ Z (6) Note that there are several alternatives in this black-box situa- tion: We could use rational approximations: III. ESTIMATION TECHNIQUES AND BASIC ¾ ½ Ò PROPERTIES · Ü · Ü · ::: · Ü ½ ¾ ¿ Ò ´Ü; µ= g (3) ¾ Ñ ½ ½· Ü · Ü · ::: · Ü ·½ Ò·¾ Ò·Ñ ½ Ò In this section we shall deal with issues that are independent or Fourier series expansions of model structure. Principles and algorithms for fitting mod- els to data, as well as the general properties of the estimated Ò X models are all model-structure independent and equally well g ´Ü; µ= · cÓ×´` ܵ· ×iÒ´` ܵ ¼ ` ½ ¾` ¾ (4) applicable to, say, linear ARMAX models and Neural Network =½ ` models, to be discussed later in this paper. Alternatively, we could approximate the function by piece- A. Criterion of Fit wise constant functions, as illustrated in Figure 1. We shall It suggests itself that the basic least-squares like approach is 1.5 Ý ´Øj µ a natural approach, even when the predictor ^ is a more general function of : 1.4 Æ ^ = aÖg ÑiÒ Î ´; Z µ Æ 1.3 Æ (7) 1.2 where 1.1 Æ X ½ Æ ¾ Î ´; Z µ= kÝ ´Øµ Ý^´Øj µk Æ (8) 1 Æ Ø=½ 0.9 We shall also use the following notation for the discrepancy 0.8 between measurement and predicted value 0.7 ´Ø; µ=Ý ´Øµ Ý^´Ø; j µ 1 2 3 4 5 6 7 8 9 10 " (9) Fig. 1. A piece-wise constant approximation. This procedure is natural and pragmatic – we can think of it ´Øµ Ý^´Øj µ as “curve-fitting” between Ý and . It also has several in Section VI return to a formal model description of the pa- statistical and information theoretic interpretations. Most im- rameterization in this figure. portantly, if the noise source in the system is supposed to be e´Øµg It is now clear that the basic steps of black-box modeling are a Gaussian sequence of independent random variables f as follows: then (7) becomes the Maximum Likelihood estimate (MLE). Ý ´k j µ 1. Choose the “type” of model structure class. (For exam- If ^ is a linear function of the minimization problem ple, in the case above, Fourier transform, rational func- (7) is easily solved. In more general cases the minimization tion, or piecewise constant.) will have to be carried out by iterative (local) search for the 2. Determine the “size” of this model (i.e. the number of minimum: ´i·½µ ´iµ ´iµ Ò parameters, ). This will correspond to how “fine” the Æ ^ ^ ^ = · f ´Z ; µ (10) Æ Æ approximation is. Æ Î 3. Use observed data both to estimate the numerical values f where typically is related to the gradient of Æ , like the of the parameters and to select a suitable value of Ò. Gauss-Newton direction. See, e.g. [1], Chapter 10. We shall generally denote a model structure for the observa- It is also quite useful work with a modified criterion tions by Æ Æ ¾ Ï ´; Z µ=Î ´Z µ·Æ k k Æ Æ (11) Ý^´k j µ=g ´Ü ;µ k (5) Î with Æ defined by (8). This is known as regularization.It Ý ´k j µ ´iµ It is to interpreted so that ^ is the predicted value of the may be noted that stopping the iterations in (10) before the ´k µ observation Ý assuming the function can be described by minimum has been reached has the same effect as regulariza- the parameter vector . tion. See, e.g., [2]. !½ B. Convergence as Æ covariance matrix of this sensitivity derivative. This is a quite natural result. An essential question is, of course, what will be the properties of the estimate resulting from (8). These will naturally depend The result (14) - (15) is general and holds for all model struc- Æ on the properties of the data record Z . It is in general a dif- tures, both linear and non-linear ones, subject only to some ^ ficult problem to characterize the quality of Æ exactly. One regularity and smoothness conditions. They are also fairly nat- normally has to be content with the asymptotic properties of ural, and will give the guidelines for all user choices involved ^ Æ Æ as the number of data, , tends to infinity.
Details
-
File Typepdf
-
Upload Time-
-
Content LanguagesEnglish
-
Upload UserAnonymous/Not logged-in
-
File Pages11 Page
-
File Size-