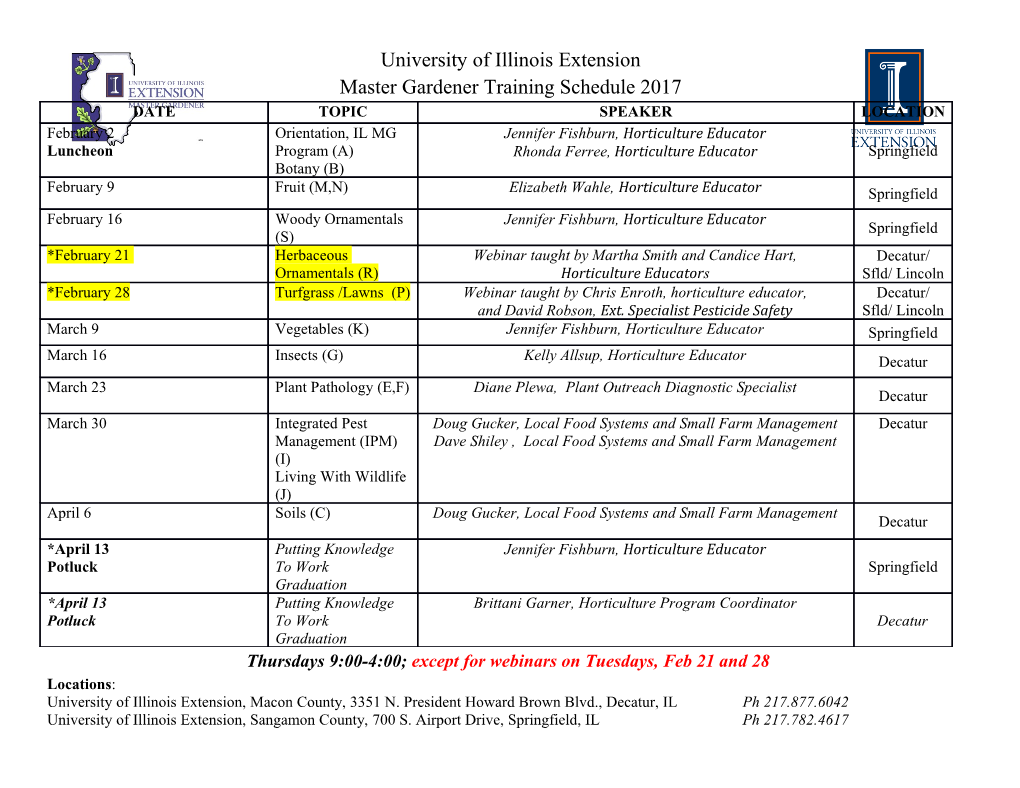
Randomized Algorithms 1. Concepts of randomized algorithms 2. Randomize algorithm analysis 3. Randomized complexity classes 4. Pseudorandom number generation 5. Global min-cut problem 6. Maximum 3-SAT 7. Primality test CP412 ADAII Introduction # 1 1. Concepts of randomized algorithms • A randomized algorithm is an algorithm whose working not only depends on the input but also on certain random choices made by the algorithm during the execution – A randomized algorithm depends on random numbers for its operation – Assume that we have a random number generator Random(a, b) that generates for two integers a, b with a < b an integer r with a <=r <= b uniformly at random. In other words. We assume that Random(a, b) runs in O(1) time. Examples randomized quick sort: randomly choose a pivoting element CP412 ADAII Introduction # 2 Deterministic vs Randomized algorithms Boolean equivalence problem: given two boolean functions f1(x1, .., xn) and f2(x1, …, xn), question: f1=f2 ? Deterministic algorithm: for each X in the enumeration of {0, 1}n if f1(X) != f2(X) return false; return true Randomized algorithm (probabilistic algorithms): for k from 1 to N (or while true), randomly choose X from {0, 1}n if f1(X) != f2(X) return false; return true Note that “true” output of the above probabilistic algorithm may not be correct. But the “false” output is an correct answer. CP412 ADAII Introduction # 3 Two types of randomized algorithms • Las Vegas algorithms – use the randomness to reduce the expected running time or memory usage of computing process, but always terminate with a correct result in a bounded amount of time. e.g. randomized quicksort • Monte Carlo algorithms (probabilistic algorithms) – depend on the random input, have a chance of producing an incorrect result or fail to produce a result either by signalling a failure or failing to terminate e.g. Randomized Boolean equivalence algorithm CP412 ADAII Introduction # 4 Why randomized algorithms? • Use randomized algorithm to find a solution • Using randomness to improve algorithm performance • Using randomness to improve a solution to a problem • Generating random data for testing • Simulation CP412 ADAII Introduction # 5 3. Analysis • Resource usage for randomized algorithms – expected worst-case performance, which is the average amount of time it takes on the worst input of a given size. This average is computed over all the possible outcomes of the random numbers during the execution of the algorithm. – Generally for Las Vegas algorithms For randomized algorithm A, when input x and random bits r are given, it becomes deteminstic algorithm. Let TA (x, r) denote the computing time of the algorithem. TA (x, r) is a random function of random variable r. The expected running time is E[TAA (x, r) ] = T (x, r) P[r] r 6 Randomized QuickSort • Pick a pivot p uniformly at random from the elements of the array Let T(n) be the expected number of comparisons done on an array of n elements. We have T(0) = T(1) = 0 and for larger n. Because there are n equally-likely choices for our pivot (hence the 1/n), and for each choice the expected cost is T(k) + T(n − 1 − k), where k is the number of elements less than the pivot. 7 1 n1 Tn() (() Tk Tn ( 1 k )) n k0 222nnn111 Tk() Tk () ak log k nnnkkk011 22 22log1aannnn kkdklog ( ) nn1 244 On(log) n more precise: Tn( ) 2 n log n 8 Expectation Expectation. Given a discrete random variables X, its expectation E[X] is defined by: Waiting for a first success. Coin is heads with probability p and tails with probability 1– p. How many independent flips X until first heads? Linearity of expectation. Given two random variables X and Y defined over the same probability space, E[X + Y] = E[X] + E[Y]. CP412 ADAII Introduction # 9 Guessing cards Game. Shuffle a deck of n cards; turn them over one at a time; try to guess each card. Guessing with memory. Guess a card uniformly at random from cards not yet seen. Claim. The expected number of correct guesses is Θ(log n). Coupon collector Coupon collector. Each box of cereal contains a coupon. There are n different types of coupons. Assuming all boxes are equally likely to contain each coupon, how many boxes before you have ≥ 1 coupon of each type? Claim. The expected number of steps is Θ(n log n). Monte Carlo algorithm analysis For Monte Carlo algorithms, when an random input is given, the execution is deterministic, but may get incorrect answer. We are interested in the probability of getting correct answer. To get high probability of correctness, we can do many runs. The overall running time is the number of runs times the time of each run. E.g. Boolean equivalence problem, we are interested in probability of getting a correct answer of the probabilistic algorithm. • For decision problems, these algorithms are generally classified as either false-biased or true-biased. A false-biased Monte Carlo algorithm is always correct when it returns false; . a true-biased algorithm is always correct when it returns true 12 Randomized complexity classes The class R or RP (Randomized Polynomial time) consists of languages L for which a polynomial-time Turing machine M exists such that P[M(x, r) = 1] ≥ 1/2 if x is in L P[M(x, r) = 1] = 0 if x is not in L In other words, we can find a witness that x is in L with constant probability. With n runs: P[Get yes answer] ≥ 1 − 2−n . The class co-R (co-RP): P[M(x, r) = 1] ≥ 1/2 if x is not L P[M(x, r) = 1] = 0 if x is in L CP412 ADAII Introduction # 13 Randomized complexity classes The class ZPP (Zero-error Probabilistic Polynomial time) is defined as RP union co-RP. The class BPP (Bounded-error Probabilistic Polynomial time): P[M(x, r) = 1] ≥ 2/3 if x is in L P[M(x, r) = 1] ≤ 1/3 if x is not in L The class PP (Probabilistic Polynomial time): P[M(x, r) = 1] > 1/2 if x is in L P[M(x, r) = 1] ≤ 1/2 if x is not in L CP412 ADAII Introduction # 14 4. Random number generation “Anyone who attempts to generate random numbers by deterministic means is, of course, living in a state of sin.” —John von Neuman • The computer is not capable of generating truly random numbers – The computer can only generate pseudorandom numbers-- numbers that are generated by a formula – Pseudorandom numbers look random, but are perfectly predictable if you know the formula • Pseudorandom numbers are good enough for most purposes, but not all--for example, not for serious security applications – Devices for generating truly random numbers do exist • They are based on physics properties CP412 ADAII Properties of good pseudorandom number generator 1. The numbers must have a correct distribution. In simulations, it is important that the sequence of random numbers is uncorrelated (i.e. numbers in the sequence are not related in any way). In numerical integration, it is important that the distribution is flat. 2. The sequence of random numbers must have a long period. All random number generators will repeat the same sequence of numbers eventually, but it is important that the sequence is sufficiently long. 3. The sequences should be reproducible. Often it is necessary to test the effect of certain simulation parameters and the exact same sequence of random numbers should be used to run many different simulation runs. It must also be possible to stop a simulation run and then re-continue the same run which means that the state of the RNG must be stored in memory. 4. The RNG should be easy to export from one computing environment to another. 5. The RNG must be fast. Large amounts of random numbers are needed in simulations. 6. The algorithm must be parallelizable. 16 Random Bit/Number Generator RBG: a device or algorithm which outputs a sequence of statistically independent and unbiased binary digits • Hardware-based – elapsed time between emission of particle during radioactive decay – thermal noise from a semiconductor diode or resistor; – the frequency instability of a free running oscillator; – air turbulence within disk drive which causes random fluctuations – drive sector read latency times – sound from a microphone or video input from a camera. • Software-based – the system clock – elapsed time between keystrokes or mouse movement – content of input/output buffers – user input – operating system values such as system load and network statistics Adopted from slides of Introduction # 17 Pseudo Random Bit/Number Generator PRBG – Input: a seed i.e. a truly random input sequence of length k (the seed) – Output: a deterministic sequence of length l >> k that “seems random” An adversary cannot efficiently distinguish between sequences of PRBG and truly RBG of length l. Introduction # 18 CP412 ADAII Introduction # 19 CP412 ADAII Introduction # 20 CP412 ADAII Introduction # 21 PRBG test CP412 ADAII Introduction # 22 CP412 ADAII Introduction # 23 CP412 ADAII Introduction # 24 CP412 ADAII Introduction # 25 CP412 ADAII Linear congruential generator • The simple way of generating pseudo-random numbers is by the linear congruential generator (LCG): xi+1 = (a * xi + b) % m, i=0, 1, 2, …., ; where a, b an m are constant numbers, say a, b are large prime numbers m is 232 or 264 – The initial value of x0 is called the seed LCG(a, b, m) or with mixed b>0, MLCG(a, m) GGL: 31 xi+1 = (16807xi) mod (2 −1) RAND: 32 32 xi+1 = ((69069xi+1) mod (2 ) or LCG(69069, 1, 2 ) 27 RAND implementation #include <stdio.h> double lcg(long int *xi) /* m=2**32 */ #include <stdlib.h> { long int getseed(); static long int a=69069, double lcg(long int *xi); b=1, m=4294967296; main(int argc, char **argv) { static double rm=4294967296.0; long int seed; *xi = (*xi*a+b)%m; int i; return (double)*xi/rm; double r1, r2; } /* Seed calculated from time */ seed = getseed(); #include <sys/time.h> /* Generate random number pairs */ long int getseed() { for(i=1; i<=20000; i++) { long int i; r1 = lcg(&seed); r2 = lcg(&seed); struct timeval tp; fprintf(stdout,"%g %g\n",r1,r2); if (gettimeofday(&tp,(struct timezone *) NULL)==0) { } i=tp.tv_sec+tp.tv_usec; return(0); i=i%1000000|1; } return i; } else { return -1; } } Introduction # 28 Visual test • 20000 random number pairs in two dimensions on a unit square CP412 ADAII Introduction # 29 Lagged Fibonacci generators • A lagged Fibonacci generator requires an initial set of elements x1, x2, ….
Details
-
File Typepdf
-
Upload Time-
-
Content LanguagesEnglish
-
Upload UserAnonymous/Not logged-in
-
File Pages45 Page
-
File Size-