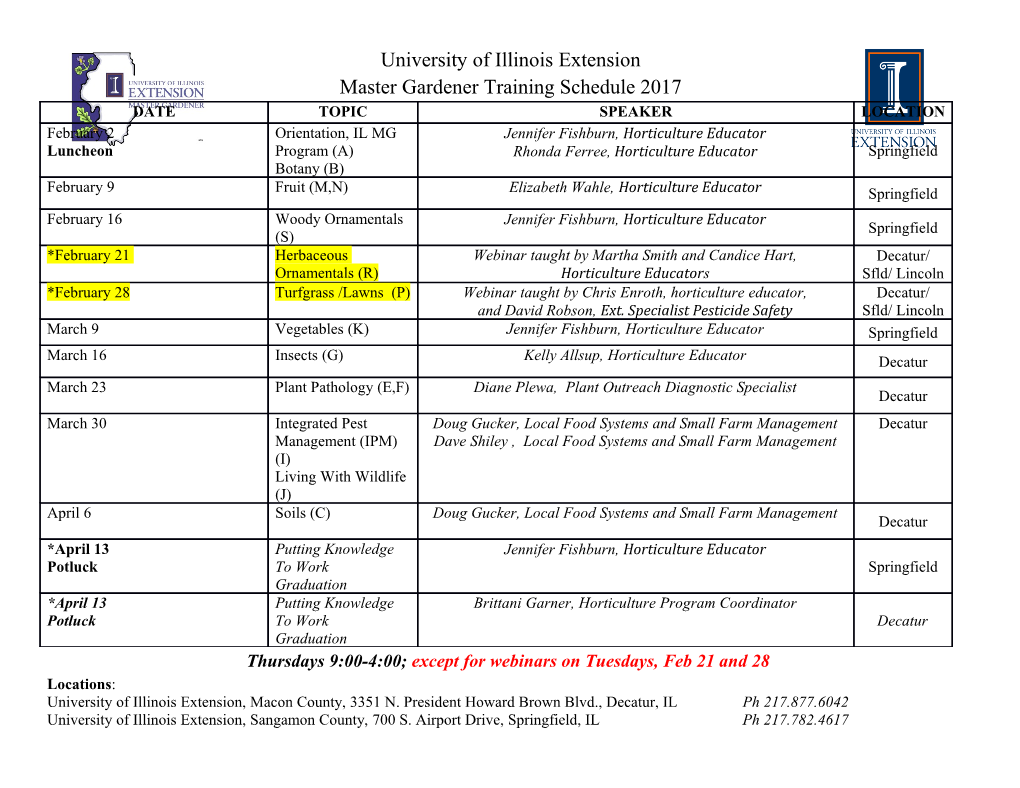
Università degli Studi di Milano-Bicocca Dipartimento di Statistica e Metodi Quantitativi Dottorato di ricerca in matematica per l’analisi dei mercati finanziari XXVI Ciclo MULTIVARIATE LÉVY MODELS: ESTIMATION AND ASSET ALLOCATION Tesi di Dottorato di ANGELA LOREGIAN matricola 072377 Relatori: Proff. Gianluca Fusai e Laura Ballotta Abstract Multidimensional asset models based on Lévy processes have been introduced to meet the necessity of capturing market shocks using more refined distribution assumptions com- pared to the standard Gaussian framework. In particular, along with accurately modeling marginal distributions of asset returns, capturing the dependence structure among them is of paramount importance, for example, to correctly price derivatives written on more than one underlying asset. Most of the literature on multivariate Lévy models focuses in fact on pricing multi-asset products, which is also the case of the model introduced in Ballotta and Bonfiglioli(2014). Believing that risk and portfolio management applications may benefit from a better description of the joint distribution of the returns as well, we choose to adopt Ballotta and Bonfiglioli(2014) model for asset allocation purposes and we empirically test its performances. We choose this model since, besides its flexibility and the ability to properly capture the dependence among assets, it is simple, relatively par- simonious and it has an immediate and intuitive interpretation, retaining a high degree of mathematical tractability. In particular we test two specifications of the general model, assuming respectively a pure jump process, more precisely the normal inverse Gaussian process, or a jump-diffusion process, precisely Merton’s jump-diffusion process, for all the components involved in the model construction. To estimate the model we propose a simple and easy-to-implement three-step proce- dure, which we assess via simulations, comparing the results with those obtained through a more computationally intensive one-step maximum likelihood estimation. We empirically test portfolio construction based on multivariate Lévy models assum- ing a standard utility maximization framework; for the exponential utility function we get a closed form expression for the expected utility, while for other utility functions (we choose to test the power one) we resort to numerical approximations. Among the benchmark strategies, we consider in our study what we call a ‘non-parametric optimization approach’, based on Gaussian kernel estimation of the portfolio return dis- tribution, which to our knowledge has never been used. A different approach to allocation decisions aims at minimizing portfolio riskiness re- quiring a minimum expected return. Following Rockafellar and Uryasev(2000), we de- scribe how to solve this optimization problem in our multivariate Lévy framework, when risk is measured by CVaR. Moreover we present formulas and methods to compute, as ef- ficiently as possible, some downside risk measures for portfolios made of assets following the multivariate Lévy model by Ballotta and Bonfiglioli(2014). More precisely, we con- sider traditional risk measures (VaR and CVaR), the corresponding marginal measures, which evaluate their sensibility to portfolio weights alterations, and intra-horizon risk measures, which take into account the magnitude of losses that can incur before the end of the investment horizon. Formulas for CVaR in monetary terms and marginal mea- sures, together with our approach to evaluate intra-horizon risk, are among the original contributions of this work. Contents Introduction 10 Literature review..................................... 12 1 Essential notions 17 1.1 Stochastic processes and filtrations........................ 17 1.2 Characteristic function, moments and cumulants............... 18 1.3 Lévy processes in finance.............................. 20 1.3.1 The normal inverse Gaussian process (NIG).............. 23 1.3.2 The Merton’s jump-diffusion model (MJD)................ 25 1.4 Estimation methods................................. 26 1.4.1 Method of moments (MoM)........................ 27 1.4.2 Maximum likelihood (ML)......................... 27 1.4.3 ML via Expectation-Maximization algorithm (EM)........... 27 1.4.4 Spectral generalized method of moments (sGMM)........... 28 1.5 Evaluating estimators............................... 29 2 Multivariate Lévy models via linear transformation 31 2.1 Model construction................................. 32 2.2 Strengths of the model............................... 33 2.3 Model estimation.................................. 35 2.3.1 A three-step estimation procedure.................... 36 2.3.1.1 Simulation study: loadings................... 39 2.3.1.2 Simulation study: the ‘all NIG’ model............. 44 2.3.1.3 Simulation study: the ‘all MJD’ model............. 53 2.3.2 One-step approach: maximum likelihood................ 60 2.3.2.1 Simulation study: the ‘all-NIG’ model............. 60 2.3.2.2 Simulation study: the ‘all-MJD’ model............. 67 2.3.3 Estimation on real data.......................... 74 3 Application to portfolio allocation 77 3.1 The investor’s problem............................... 77 3.2 Asset allocation with the multivariate Lévy model............... 79 3.3 Benchmarks..................................... 80 3.3.1 Equally weighted portfolio [EQ]...................... 80 3.3.2 Mean-variance portfolio [MV]....................... 81 3.3.3 Four-moments based allocation (Single Factor approach) [SF].... 82 3.3.4 Non-parametric optimization [NP].................... 85 3.4 Performance measures............................... 86 3.5 Data.......................................... 88 3.6 Methodology and results.............................. 95 3.6.1 Daily horizon................................ 95 3.6.1.1 CARA utility function...................... 98 3.6.1.2 CRRA utility function...................... 102 3.6.2 Weekly horizon............................... 106 3.6.2.1 CARA utility function...................... 107 3.6.2.2 CRRA utility function...................... 111 3.6.3 Summarizing allocation results...................... 115 4 Downside risk measures for Lévy portfolios 119 4.1 Value at risk, expected shortfall and marginal measures........... 120 4.1.1 Value at risk (VaR)............................. 120 4.1.2 Expected shortfall (ES/CVaR/TCE).................... 121 4.1.3 Marginal value at risk (M-VaR)...................... 122 4.1.4 Marginal expected shortfall (M-ES)................... 124 4.2 Intra-horizon risk.................................. 124 4.2.1 Definition of intra-horizon risk measures................ 125 4.2.2 Estimating the first-passage probability................. 127 4.3 Portfolio optimization with CVaR......................... 130 4.3.1 Framework.................................. 131 4.3.2 CVaR optimization: general theory.................... 131 4.3.3 CVaR optimization: multivariate Lévy model.............. 134 4.4 Application...................................... 135 Conclusions and future work 141 Appendices 143 A Proof of statements in Chapter2 143 A.1 Proof of Proposition 1................................ 143 A.2 Proof of Corollary 1................................. 144 A.3 Proof of Corollary 2................................. 145 B EM algorithm for Merton’s JD model 146 C CARA utility and normal returns 149 D Testing the equality of Sharpe ratios 150 D.1 Pairwise Sharpe ratio test............................. 150 D.2 Multivariate Sharpe ratio test........................... 151 E Formula for ES in monetary terms 153 List of Figures 2.1 Three step procedure assessment: first loading.................. 41 2.2 Three step procedure assessment: loadings.................... 43 2.3 Three step procedure assessment: ’all-NIG’ model, common factor (ML)... 47 2.4 Three step procedure assessment: ’all-NIG’ model, common factor (sGMM). 49 2.5 Three step procedure assessment: ’all-NIG’ model, first idiosyncratic com- ponent (ML)...................................... 52 2.6 Three step procedure assessment: ’all-MJD’ model, common factor (EM)... 55 2.7 Three step procedure assessment: ’all-MJD’ model, first idiosyncratic com- ponent (EM)..................................... 59 2.8 One-step approach assessment: ’all-NIG’ model, common factor....... 62 2.9 One-step approach assessment: ’all-NIG’ model, first idiosyncratic component 63 2.10 One-step approach assessment: ’all-NIG’ model, first loading......... 64 2.11 Likelihood comparison (‘all-NIG’ model)..................... 66 2.12 One-step approach assessment: ’all-MJD’ model, common factor....... 69 2.13 One-step approach assessment: ’all-MJD’ model, first idiosyncratic component 71 2.14 One-step approach assessment: ’all-MJD’ model, first loading........ 72 2.15 Likelihood comparison (‘all-MJD’ model)..................... 73 2.16 Estimation on real data: Apple Inc. returns distribution........... 75 2.17 Estimation on real data: covariance matrix................... 76 3.1 Data: time series and histograms (1)....................... 90 3.2 Data: time series and histograms (2)....................... 91 3.3 Data: time series and histograms (3)....................... 92 3.4 Data: time series and histograms (4)....................... 93 3.5 Data: time series and histograms (5)....................... 94 3.6 Rolling window strategy (daily horizon)..................... 96 3.7 Weights: daily horizon; CARA utility (λ = 10); n = 10.............. 98 3.8 Weights: daily horizon; CARA utility (λ = 15); n = 10.............. 99 3.9 Weights: daily
Details
-
File Typepdf
-
Upload Time-
-
Content LanguagesEnglish
-
Upload UserAnonymous/Not logged-in
-
File Pages163 Page
-
File Size-