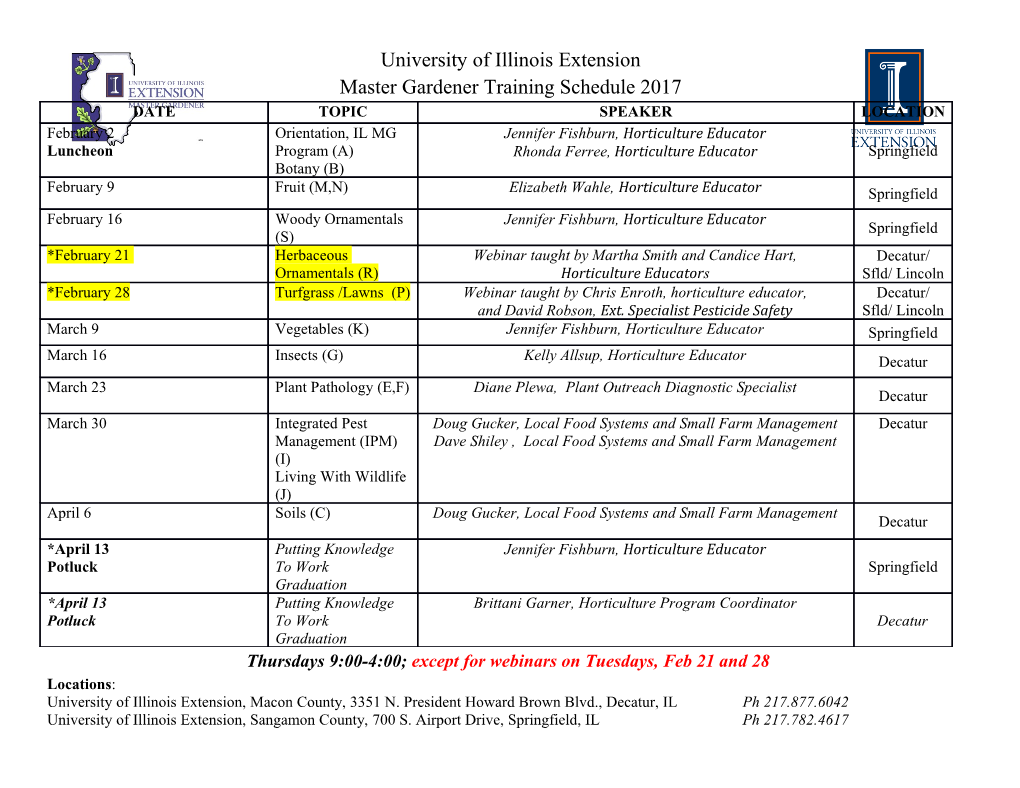
New algorithms for the analysis of live-cell images acquired in phase contrast microscopy Thèse Pierre-Marc Juneau Doctorat en génie chimique Philosophiæ doctor (Ph.D.) Québec, Canada © Pierre-Marc Juneau, 2015 ii Résumé La détection et la caractérisation automatisée des cellules constituent un enjeu important dans de nombreux domaines de recherche tels que la cicatrisation, le développement de l'embryon et des cellules souches, l’immunologie, l’oncologie, l'ingénierie tissulaire et la découverte de nouveaux médicaments. Étudier le comportement cellulaire in vitro par imagerie des cellules vivantes et par le criblage à haut débit implique des milliers d'images et de vastes quantités de données. Des outils d'analyse automatisés reposant sur la vision numérique et les méthodes non-intrusives telles que la microscopie à contraste de phase (PCM) sont nécessaires. Comme les images PCM sont difficiles à analyser en raison du halo lumineux entourant les cellules et de la difficulté à distinguer les cellules individuelles, le but de ce projet était de développer des algorithmes de traitement d'image PCM dans Matlab® afin d’en tirer de l’information reliée à la morphologie cellulaire de manière automatisée. Pour développer ces algorithmes, des séries d’images de myoblastes acquises en PCM ont été générées, en faisant croître les cellules dans un milieu avec sérum bovin (SSM) ou dans un milieu sans sérum (SFM) sur plusieurs passages. La surface recouverte par les cellules a été estimée en utilisant un filtre de plage de valeurs, un seuil et une taille minimale de coupe afin d'examiner la cinétique de croissance cellulaire. Les résultats ont montré que les cellules avaient des taux de croissance similaires pour les deux milieux de culture, mais que celui-ci diminue de façon linéaire avec le nombre de passages. La méthode de transformée par ondelette continue combinée à l’analyse d'image multivariée (UWT-MIA) a été élaborée afin d’estimer la distribution de caractéristiques morphologiques des cellules (axe majeur, axe mineur, orientation et rondeur). Une analyse multivariée réalisée sur l’ensemble de la base de données (environ 1 million d’images PCM) a montré d'une manière quantitative que les myoblastes cultivés dans le milieu SFM étaient plus allongés et plus petits que ceux cultivés dans le milieu SSM. Les algorithmes développés grâce à ce projet pourraient être utilisés sur d'autres phénotypes cellulaires pour des applications de criblage à haut débit et de contrôle de cultures cellulaires. iii iv Abstract Automated cell detection and characterization is important in many research fields such as wound healing, embryo development, immune system studies, cancer research, parasite spreading, tissue engineering, stem cell research and drug research and testing. Studying in vitro cellular behavior via live-cell imaging and high-throughput screening involves thousands of images and vast amounts of data, and automated analysis tools relying on machine vision methods and non-intrusive methods such as phase contrast microscopy (PCM) are a necessity. However, there are still some challenges to overcome, since PCM images are difficult to analyze because of the bright halo surrounding the cells and blurry cell-cell boundaries when they are touching. The goal of this project was to develop image processing algorithms to analyze PCM images in an automated fashion, capable of processing large datasets of images to extract information related to cellular viability and morphology. To develop these algorithms, a large dataset of myoblasts images acquired in live-cell imaging (in PCM) was created, growing the cells in either a serum-supplemented (SSM) or a serum-free (SFM) medium over several passages. As a result, algorithms capable of computing the cell-covered surface and cellular morphological features were programmed in Matlab®. The cell-covered surface was estimated using a range filter, a threshold and a minimum cut size in order to look at the cellular growth kinetics. Results showed that the cells were growing at similar paces for both media, but their growth rate was decreasing linearly with passage number. The undecimated wavelet transform multivariate image analysis (UWT- MIA) method was developed, and was used to estimate cellular morphological features distributions (major axis, minor axis, orientation and roundness distributions) on a very large PCM image dataset using the Gabor continuous wavelet transform. Multivariate data analysis performed on the whole database (around 1 million PCM images) showed in a quantitative manner that myoblasts grown in SFM were more elongated and smaller than cells grown in SSM. The algorithms developed through this project could be used in the future on other cellular phenotypes for high-throughput screening and cell culture control applications. v vi Table of Contents Résumé ............................................................................................................................. iii Abstract ............................................................................................................................. v Table of Contents ............................................................................................................. vii Tables list ......................................................................................................................... xi Figures list ....................................................................................................................... xiii Abbreviations list ............................................................................................................. xxi Remerciements ............................................................................................................. xxix Foreword ....................................................................................................................... xxxi Chapter 1. Introduction .................................................................................................. 1 Chapter 2. Literature Review ......................................................................................... 7 2.1 High throughput Screening (HTS) ......................................................................... 7 2.2 Culture of myoblasts ............................................................................................ 11 2.2.1 Cell culture .................................................................................................... 11 2.2.2 Culture medium ............................................................................................ 14 2.2.3 Cellular phenotypes associated to the myogenic lineage ............................. 15 2.2.4 Cell morphology ............................................................................................ 23 2.2.5 Cellular growth .............................................................................................. 30 2.3 Microscopy ........................................................................................................... 34 2.3.1 Types of microscopy ..................................................................................... 34 2.3.2 Controlled environment for live cell imaging ................................................. 43 2.3.3 Cell culture sample integrity ......................................................................... 44 2.3.4 Microscopic image acquisition ...................................................................... 48 2.4 Image processing ................................................................................................ 56 2.4.1 Definition of a digital image .......................................................................... 56 2.4.2 Fourier transform of a digital image .............................................................. 58 2.4.3 Image pre-processing ................................................................................... 62 2.4.4 Segmentation algorithms .............................................................................. 68 2.4.5 Pattern recognition ....................................................................................... 83 2.4.6 Wavelet transforms ....................................................................................... 95 2.4.7 Classifiers ................................................................................................... 112 2.4.8 Parameters calibration ................................................................................ 113 2.4.9 Commercial products/softwares for image processing ............................... 121 vii 2.5 Multivariate data analysis ................................................................................... 125 2.5.1 Matrix of features ........................................................................................ 125 2.5.2 Multivariate techniques ............................................................................... 126 Chapter 3. Materials and Methods ............................................................................ 141 3.1 Cell culture ......................................................................................................... 141 3.2 Microscopy ......................................................................................................... 141 3.3 Image acquisition ............................................................................................... 145 3.4 Image processing and data analysis .................................................................
Details
-
File Typepdf
-
Upload Time-
-
Content LanguagesEnglish
-
Upload UserAnonymous/Not logged-in
-
File Pages434 Page
-
File Size-