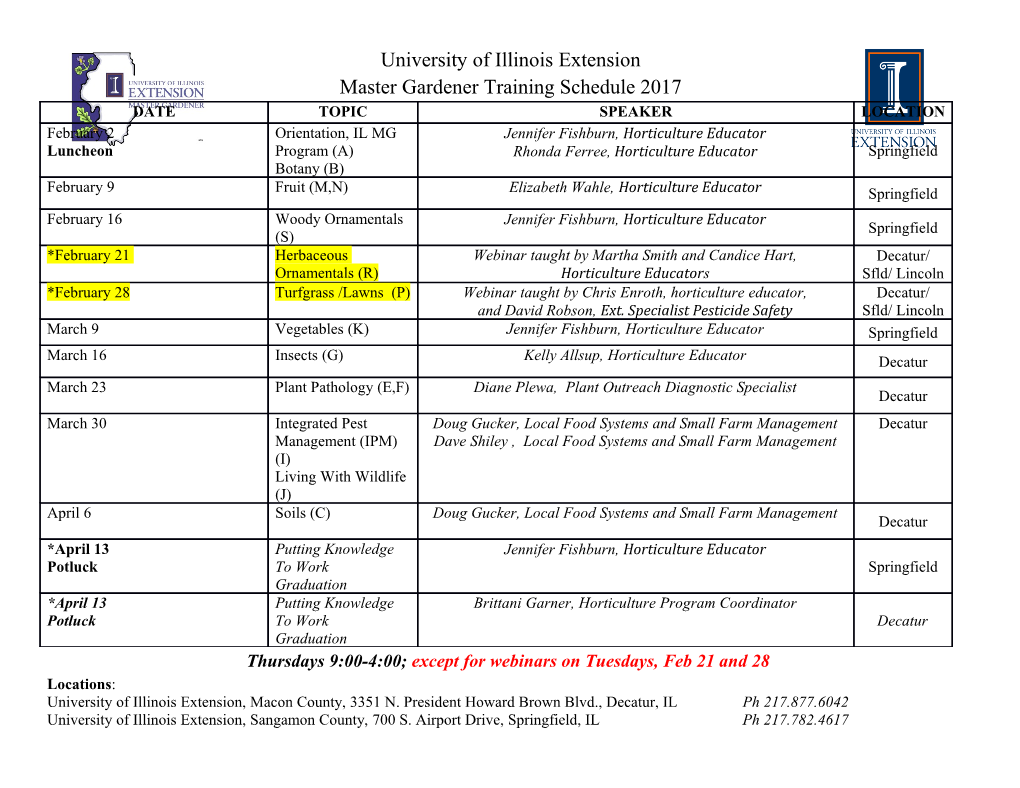
Liouville Equation In this section we will build a bridge from Classical Mechanics to Statistical Physics. The bridge is Liouville equation. We start with the Hamiltonian formalism of the Classical Mechanics, where the state of a system with m degrees of freedom is described by m pairs of conjugated variables called (generalized) coordinates and momenta fqs; psg, s = 1; 2; : : : ; m. The equations of motion are generated with the Hamiltonian function, H(fqs; psg), by the following rule @H q_s = ; (1) @ps @H p_s = ¡ : (2) @qs Fore example, if we have N three-dimensional particles of mass M interact- ing with each other via a pair potential U, and also interacting with some external potential V , then the Hamiltonian for this system reads: XN p2 XN X H = j + V (r ) + U(r ¡ r ) ; (3) 2M j i j j=1 j=1 i<j where rj and pj are the radius-vector and momentum of the j-th particle, respectively. In this example, m = 3N: each component of each radius- vector represents a separate degree of freedom. The following property of Eqs. (1)-(2) will be crucial for us. If we need to describe time evolution of some function A(fqs; psg) due to the evolution of coordinates and momenta, then the following relation takes place A_ = fH; Ag ; (4) where the symbol in the r.h.s. is a shorthand notation|called Poisson bracket|for the following expression X @H @A @H @A fH; Ag = ¡ : (5) s @ps @qs @qs @ps [The proof is straightforward. The chain rule for dA(fqs(t); ps(t)g)=dt and then Eqs. (1)-(2) forq _s andp _s.] 1 Hence, any quantity A(fqs; psg) is a constant of motion if, and only if, its Poisson bracket with the Hamiltonian is zero. In particular, the Hamiltonian itself is a constant of motion, since fH; Hg = 0, and this is nothing else than the conservation of energy, because the physical meaning of the Hamiltonian function is energy expressed in terms of coordinates and momenta. De¯nition: The phase space is a 2m-dimensional space of points, or, equivalently, vectors of the following form: X = (q1; q2; : : : ; qm; p1; p2; : : : ; pm) : (6) Each point/vector in the phase space represents a state of the mechanical system. If we know X at some time moment, say, t = 0, then the further evolution of X|the trajectory X(t) in the phase space|is unambiguously given by Eqs. (1)-(2), since these are the ¯rst-order di®erential equations with respect to the vector function X(t). (For the same reason di®erent trajectories cannot intersect!) The phase space is convenient for statistical description of mechanical system. Suppose that the initial state for a system is known only with a certain ¯nite accuracy. This means that actually we know only the proba- bility density W0(X) of having the point X somewhere in the phase space. If the initial condition is speci¯ed in terms of probability density, then the subsequent evolution should be also described probabilistically, that is we have to work with the distribution W (X; t), which should be somehow re- lated to the initial condition W (X; 0) = W0(X). Our goal is to establish this relation. We introduce a notion of a statistical ensemble. Instead of dealing with probability density, we will work with a quantity which is proportional to it, and is much transparent. Namely, we simultaneously take some large number Nens of identical and independent systems distributed in accordance with W (X; t). We call this set of systems statistical ensemble. The j-th member of the ensemble is represented by its point Xj in the phase space. The crucial observation is that the quantity Nens W (X; t) gives the concentration of the points fXjg. Hence, to ¯nd the evolution of W we just need to describe the evolution of the concentration of the points Xj, which is intuitively easier, since each Xj obeys the Hamiltonian equation of motion. A toy model. To get used to the ensemble description, and also to obtain some important insights, consider the following dynamical model with just one degree of freedom: H = (1=4)(p2 + q2)2 : (7) 2 The equations of motion are: q_ = (p2 + q2) p ; (8) p_ = ¡(p2 + q2) q : (9) The quantity ! = p2 + q2 (10) is a constant of motion, since, up to a numeric factor, it is a square root of energy. We thus have a linear system of equations q_ = !p ; (11) p_ = ¡!q ; (12) which is easily solved: q(t) = q0 cos !t + p0 sin !t ; (13) p(t) = p0 cos !t ¡ q0 sin !t ; (14) 2 2 where q0 ´ q(0), p0 ´ p(0), and ! = p0 + q0. We see that our system is a non-linear harmonic oscillator. It performs harmonic oscillations, but in contrast to a linear harmonic oscillator, the frequency of oscillations is a function of energy. Now we take Nens = 1000 replicas of our system and uniformly dis- tribute them within the square 0:75 · q · 1:25, ¡0:25 · p · 0:25 of the two-dimensional phase space. Then we apply the equations of motion (13)- (14) to each points and trace the evolution. Some characteristic snapshots are presented in Fig. 1. In accordance with the equationsq of motion, each 2 2 point rotates along corresponding circle of the radius p0 + q0. Since our oscillators are non-linear, points with larger radii rotate faster, and this leads to the formation of the spiral structure. The number of the spiral windings increases with time. With a ¯xed number of points in the ensemble, at some large enough time it becomes simply impossible to resolve the spiral struc- ture. For all practical purposes, it means that instead of dealing with the actual distribution W (X; t), which is beyond our \experimental" resolution, we can work with an e®ective distribution We® (X; t) obtained by slightly smearing W (X; t). [Actually, this or that sort of \smearing" (either ex- plicit or implicit) is an unavoidable ingredient of any Statistical-Mechanical description!] In contrast to the genuine distribution W (X; t) that keeps in- creasing the number of spiral windings, the smeared distribution We® (X; t) 3 saturates to a certain equilibrium (=time-independent) function, perfectly describing our ensemble at large times (see the plot for t = 1000). With our equations of motion, we see that the generic structure of our equilibrium 2 2 We® (X) (no matter what is the initial distribution) is We® (X) = f(p + q ), the particular form of the function f coming from the initial distribution. Indeed, with respect to an individual member of the ensemble, the evolu- tion is a kind of roulette that randomizes the positionp of corresponding phase 2 2 space point Xj along the circle of the radius p + q . Below we will see how this property is generalized to any equilibrium ensemble of Hamiltonian systems. 4 Figure 1: Evolution of the ensemble of 1000 systems described by the Hamil- tonian (7). After playing with a toy model, we are ready to consider a general case. From now on we normalize the function W (X; t) to the number of the en- semble members. Correspondingly, the number of points in the phase space volume ­0 at the time t is given by the integral Z N­0 (t) = W (X; t) d­ ; (15) ­0 where d­ = dq1 : : : dqm dp1 : : : dpm is the element of the phase space volume; the integration is over the volume ­0. To characterize the rate of variation of the number of points within the volume ­0, we use the following time derivative Z @ _ N­0 = W (X; t) d­ : (16) ­0 @t 5 By the de¯nition of the function W (X; t), its variable X does not depend on time, so that the time derivative deals only with the variable t. _ There is an alternative way of calculating N­0 . We may count the num- ber of points that cross the surface of the volume ­0 per unit time: Z _ N­0 = ¡ J ¢ dS : (17) surface of ­0 Here J is the flux of the points [number of points per unit (and perpendicular to velocity) surface per unit time]; dS = n dS, where n is the unit normal vector at a surface point, and dS is the surface element. We assume that n is directed outwards and thus write the sign minus in the right-hand side of (17). In accordance with the known theorem of calculus, the surface integral (17) can be converted into the bulk integral Z Z J ¢ dS = r ¢ J d­ ; (18) surface of ­0 ­0 where r is the vector di®erential operator µ ¶ @ @ @ @ r = ;:::; ; ;:::; : (19) @q1 @qm @p1 @pm We arrive at the equality Z Z @ W (X; t) d­ = ¡ r ¢ J d­ : (20) ­0 @t ­0 Since Eq. (20) is true for an arbitrary ­0, including an in¯nitesimally small one, we actually have @ W (X; t) = ¡ r ¢ J : (21) @t This is a quite general relation, known as the continuity equation. It arises in theories describing flows of conserving quantities (say, particles of fluids and gases). The dimensionality of the problem does not matter. Now we are going to independently relate the flux J to W (X; t) and thus end up with a closed equation in terms of W (X; t). By the de¯nition of J we have J = W (X; t) X_ ; (22) 6 because the flux of particles is always equal to their concentration times velocity.
Details
-
File Typepdf
-
Upload Time-
-
Content LanguagesEnglish
-
Upload UserAnonymous/Not logged-in
-
File Pages7 Page
-
File Size-