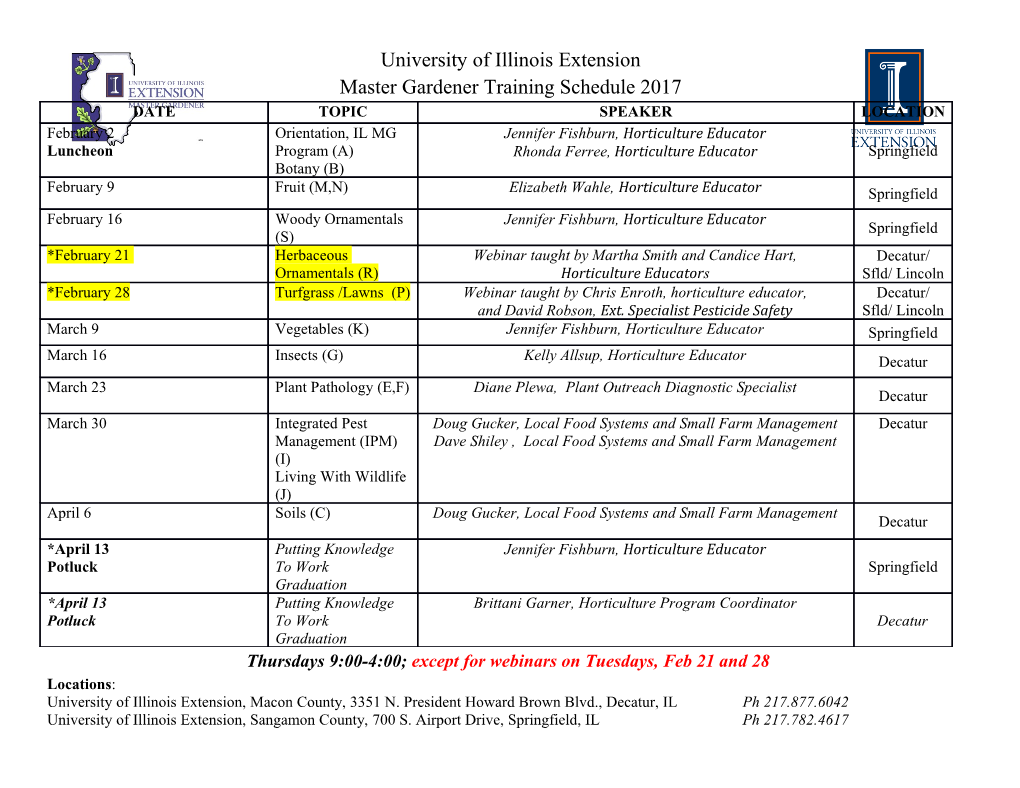
Paolo Podio-Guidugli Accademia Nazionale dei Lincei and Department of Mathematics, Universita` di Roma TorVergata Two Lectures about the Virial Theorem HMM Summer School at OIST - Okinawa, Japan 7 and 8 April 2017 PLAN Lecture I - Deterministic Virial I.1 Discrete and Newtonian I.2 Continuum and Eulerian Lecture II - Probabilistic Virial II.1 Recap of Statistical Mechanics II.2 The Equipartition Theorem II.3 The Virial Theorem Lecture I - Deterministic Virial I.1 Discrete and Newtonian – Ordinary forces – Fluctuating forces – Particle systems I.2 Continuum and Eulerian Main reference: G. Marc and W.G. McMillan, The virial theorem. Adv. Chem. Phys. 58, 209-361 (1985). I.1 Deterministic Virial, Discrete and Newtonian Ordinary forces “The mean vis viva [ kinetic energy] of the system is equal to its ⌘ [force] virial” (Rudolf Clausius, 1870) A[f x]= 2 A[k], 2 k := mx˙ x˙ mx¨ = f , x = x(t) o · − · ( − mx¨ = f mx¨ x = f x =: the virial of force f . ) · · Note that d (x˙ x) x˙ x˙ dt · − · x¨ x = 8 . · > 2 > 1 d < (x x) x˙ x˙ 2 dt2 · − · > With a use of upper alternative,:> d (mx˙ x) mx˙ x˙ = f x, dt · − · · or rather, on setting w := mx˙ x and 2 k := mx˙ x˙ , · · f x =˙w 2k. · − Introduce the time-average operator 1 ⌧ A[f]:= lim f(t)dt . ⌧ + ⌧ 7! 1 Z0 Then, for f(t)=g˙(t), we have that 1 A[f]= lim g(⌧) g(0) =0, ⌧ + 7! 1 ⌧ − provided that g only takes finite values; in particular, A[w˙ ]=0. An averaging of f x = w˙ 2k yields the Virial Theorem: · − A[f x]= 2 A[k] · − Fluctuating forces Langevin (1908) studies Brownian particles suspended in a viscous fluid at rest by means of macroscopic Newtonian mechanics: mx¨ = f + F, 1 where to standard Stokesian drag f = µ− x˙ he − adds fluctuating force F, giving the motion a stochastic character; • makes use of lower alternative: • 2 1 d 1 1 d m (x x)+ µ− (x x)=mx˙ x˙ + F x; 2 dt2 · 2 dt · · · takes arithmetic mean (no probabilistic expectation!) over large • collection of identical particles; characterizes the fluctuating force by assuming that the mean of the • virial term F x be null. · Particle systems Set • d (m x˙ x ) m x˙ x˙ = f x , f = f + f e dt i i · i − i i · i i · i i ij i j=i X X X X6 On choosing o g = mass center, get Virial Theorem in split • ⌘ form: A[F g]= 2 A[K ] · − g 8 e F := fi , 2Kg := Mg˙ g˙ ,M:= mi > · > > P P > <> A (x x ) f + x f e = 2 A[K ] i − j · ij i · i − rel i>j > ⇥ X X X ⇤ > 2Krel := mix˙ i x˙ i > · > :> P I.2 Deterministic Virial, Continuum and Eulerian Eulerian balance of linear momentum ni 0 = d ni + divT ⇢v˙ , d = dist. force, T = Cauchy stress − After dyadic multiplication by ' and integration by parts, 0 = ' d ni + ' c (grad ')T T ⇢ ' v˙ , c = Tn, ⌦ ⌦ − − ⌦ ZPt Z@Pt ZPt ZPt where c = contact forces at a point of @Pt. For ' x, ⌘ d ⇢ x v˙ = ⇢ x x˙ ⇢ v v , v = x˙ , P ⌦ dt P ⌦ − P ⌦ Z t ⇣ Z t ⌘ Z t so that d x d ni + x c T T = ⇢ x x˙ ⇢ v v. P ⌦ @P ⌦ − P dt P ⌦ − P ⌦ Z t Z t Z t ⇣ Z t ⌘ Z t Recall f x = w˙ 2k A[f x]= 2 A[k] · − ) · − Now, by taking the trace of d x d ni + x c T T = ⇢ x x˙ ⇢ v v , P ⌦ @P ⌦ − P dt P ⌦ − P ⌦ Z t Z t Z t ⇣ Z t ⌘ Z t we get ni x d + x c tr T = W˙ (Pt) 2K(Pt) Pt · @Pt · − Pt − 9 R R R 1 ) W (Pt)= ⇢x x˙ ,K(Pt):= ⇢ v v > Pt · 2 Pt ⌦ = R R ;> ni A x d + x c tr T = 2 A[K(Pt)], ) · · − − ZPt Z@Pt ZPt ⇥ ⇤ a statement of the Virial Theorem in Continuum Mechanics. By taking the symmetric part of d x d ni + x c T T = ⇢ x x˙ ⇢ v , P ⌦ @P ⌦ − P dt P ⌦ − P ⌦ Z t Z t Z t ⇣ Z t ⌘ Z t we get ni ] 1 sym x d + x c symT = I¨(Pt), P ⌦ @P ⌦ − P 2 ⇣ Z t Z t ⌘ Z t where I (Pt):= ⇢ x x = Euler inertia tensor of part Pt, ⌦ ZPt T ] := ⇢ v v + T = virial stress. − ⌦ Some call T ] the Reynolds stress, because of the form of momentum balance typical of fluid mechanics that O. Reynolds liked: ni @t(⇢v)+div(⇢ v v T)=d , ⌦ − where ⇢ v v = convective current and T = diffusive current. ⌦ − Lecture II - Probabilistic Virial II.1 Recap of Statistical Mechanics – The microcanonical ensemble – Microcanonical and time averages – How to construct an equilibrium thermodynamics II.2 The Equipartition Theorem II.3 The Virial Theorem – The Virial Theorem and the gas law Main references: - J.P. Sethna, Entropy, Order Parameters, and Complexity. Oxford Master Series in Physics, Oxford Press, 2006. - K. Huang, Statistical Mechanics. 2nd Ed. J. Wiley, 1987. II.1 Recap of Statistical Mechanics The microcanonical ensemble Consider classical Hamiltonian system N identical particles of invariable mass m • confined in a 3D box of volume V (finite molecular volume V/N) • 1 1 2 H(q, p)=K(p)+U(q),K(p)= m− p ,f(q)= @ U(q). • 2 | | − q If both particle-particle and particle-wall collisions entail no energy losses, then the total energy of the system is conserved. Three macroscopic conservation conditions – of number, volume, and energy – define the Gibbsian microcanonical ensemble N,V,E . { } Note @ other motion-related conserved quantities: confinement in a box destroys both translational and rotational symmetries. for all practical purposes, when a system is in equilibrium, all mi- • crostates of the system having the same energy initially, and hence forever, are regarded as equivalent; all microstates accessed by the system along one of its trajectories • have equal energy; (ergodicity concept) all ly many microstates of a given energy • 1 are going to be visited, sooner or later, except perhaps a subset of measure zero . We now proceed to measure (not to count!) ‘how many’ initial states there are for a given assignment of initial energy. ⌦(E)= ✓(E H(q, p))dqdp , qp-plane, ✓( )=Heaviside f. • − Z ⌘ · ZZ = volume of subgraph of H(q, p)=E ⌦(E + dE) ⌦(E)= ✓(E + dE H(q, p)) ✓(E H(q, p)) dqdp • − − − − ZZ = volume of (infinitesimal) energy shell Heaviside vs. Dirac Let D denote distributional differentiation. The distributional rela- tionships between the Heaviside and Dirac functions is f(x )= ✓(x x0)f 0(x) dx = D✓(x x )f(x)dx − 0 − − − 0 ZIR ZIR for all test functions f. Hence, D✓(x x )=δ(x x ). − 0 − 0 ⌦0(E)= δ(E H(q, p)) dqdp , δ( )=Dirac f. • − · ZZ volume partition f. := E ⌦(E)= ✓(E H(q, p)) dqdp • 7! − ZZ surface partition f. := E !(E)= δ(E H(q, p)) dqdp • 7! − ZZ measures boundary of region in where H(q, p) E. Z Note that ⌦0(E)=!(E) 1 microcanonical probability meas.:= ⇢˜ (z)= δ(E H(z)) • E !(E) − Exercise Let h(x) 0 for x<0, h(x) >efor all x>x4. Show that ⌘ ⌦(e)= ✓(e h(x)) dx =(x x )+(x x ) • − 2 − 1 4 − 3 ZIR !(e)= δ(e h(x)) dx =1+1+1+1, • − ZIR so that integration of d!(e)=δ(e h(x)) dx literally counts all − microstates x1,...,x4 where h(x)=e. Microcanonical and time averages microcanonical average of F : • 1 F (E) := F (q, p) δ(E H(q, p)) dqdp h i !(E) − ZZ (E,d)-trajectory: • a trajectory of the N-particle system under study, confined within a volume V , of energy E and duration d that is, a solution t (q(t),p(t)),t [0,d) of the motion equa- 7! 2 tions which complies with confinement condition and initial con- ⇥ ditions (q(0),p(0)), and is such that H(q(0),p(0)) = E. 1 d ⇤ time average of F : F (E) := lim F (q(t),p(t))dt. • d d !1 Z0 Microcanonical and time averages (cont.ed) Given a (E,d)-trajectory, one assumes that (i) • 1 d F (E) F (q(t),p(t))dt , h i ' d Z0 with an approximation that becomes better and better the more accurately the given trajectory visits all parts of the state space where the energy takes the prescribed value; (ii) when d , the system tends to statistical equilibrium, that • !1 is, to a situation when all quantities that are not conserved (such as F itself) are anyway independent of the initial conditions. SM, MD, and the Ergodic Hypothesis A basic assumption in SM – oftentimes referred to as the ergodic hy- pothesis – is that, for each given E, F (E) = F (E), E, h i 8 so that the microcanonical average can be calculated from a time aver- age. The role of MD is precisely to allow for evaluating statistical averages via time averages along trajectories: statistical average at equilibrium time average along trajectories ' SM MD Note| Averages and{z trajectories are} to| be chosen consistent{z with the} ensemble that fits the system under study. MD, CM, and experimental validation Current MD simulations: basic cell of attomole size • X duration of nanosecond order • T At the macroscopic scale of CM, the space-time regions considered by MD are to be re- • X ⇥ T garded as (point, instant) pairs (x, t) the measured value F (x, t) of a field F should confirm the predic- • tion F (x, t) derived from solving an appropriate initial/boundary- value problem e To relate F (x, t) with F as evaluated by means of an MD simulation, basic cell should be “large enough” (i.e., much larger than the • correlation length of the spatial correlation function of interest) duration should be “long enough” (i.e., much longer than the re- • laxation time of the property of interest) Providing these quantitative conditions are met, MD furnishes a bridge between SM and CM: statistical average at equilibrium time average along trajectories ' SM MD measured value .
Details
-
File Typepdf
-
Upload Time-
-
Content LanguagesEnglish
-
Upload UserAnonymous/Not logged-in
-
File Pages36 Page
-
File Size-