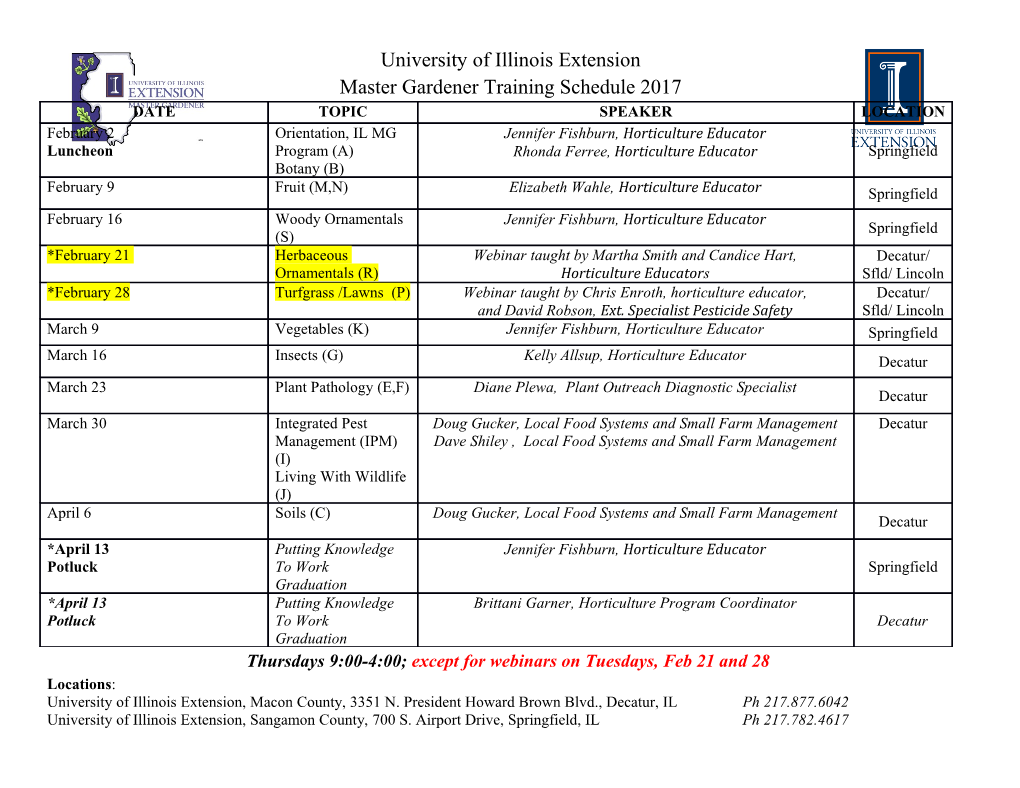
Journal of Computer and Communications, 2021, 9, 20-35 https://www.scirp.org/journal/jcc ISSN Online: 2327-5227 ISSN Print: 2327-5219 Deepfakes Detection Techniques Using Deep Learning: A Survey Abdulqader M. Almars College of Computer Science and Engineering, Taibah University, Yanbu, Saudi Arabia How to cite this paper: Almars, A.M. Abstract (2021) Deepfakes Detection Techniques Using Deep Learning: A Survey. Journal of Deep learning is an effective and useful technique that has been widely ap- Computer and Communications, 9, 20-35. plied in a variety of fields, including computer vision, machine vision, and https://doi.org/10.4236/jcc.2021.95003 natural language processing. Deepfakes uses deep learning technology to ma- Received: April 12, 2021 nipulate images and videos of a person that humans cannot differentiate Accepted: May 16, 2021 them from the real one. In recent years, many studies have been conducted to Published: May 19, 2021 understand how deepfakes work and many approaches based on deep learn- ing have been introduced to detect deepfakes videos or images. In this pa- Copyright © 2021 by author(s) and Scientific Research Publishing Inc. per, we conduct a comprehensive review of deepfakes creation and detection This work is licensed under the Creative technologies using deep learning approaches. In addition, we give a thorough Commons Attribution International analysis of various technologies and their application in deepfakes detection. License (CC BY 4.0). Our study will be beneficial for researchers in this field as it will cover the re- http://creativecommons.org/licenses/by/4.0/ cent state-of-art methods that discover deepfakes videos or images in social Open Access contents. In addition, it will help comparison with the existing works because of the detailed description of the latest methods and dataset used in this do- main. Keywords Deepfakes, Deep Learning, Fake Detection, Social Media, Machine Learning 1. Introduction With the technology becoming accessible to any user, lots of deepfake videos have been spread through social media. Deepfake refers to manipulated digital media such as images of videos where the image or video of a person is replaced with another person’s likeness. In fact, deepfake is one of the increasingly serious issues in modern society. Deepfake has been frequently used to swipe faces of popular Hollywood celebrities over porn images videos deepfake was also used to produce misleading information and rumors for politicians [1] [2] [3]. In DOI: 10.4236/jcc.2021.95003 May 19, 2021 20 Journal of Computer and Communications A. M. Almars 2018, a fake video for Barack Obama was created to putting words he never ut- tered [4]. In addition, In the US 2020 election, deepfakes have already been used to manipulate Joe Biden videos showing his tongue out. These harmful uses of deepfakes can have a serious impact on our society and can also result in spreading miss leading information, especially on social media. Generative adversarial networks (GANs) [5] are generative and sophisticated deep learning technologies that can be applied to generate fake images and vid- eos that hard for a human to identify from the true ones. Those models are used to train on a data set and then create fake images and videos. This kind of deep- fake model requires a large set of training data for those deepfake media. The larger data set, the more believable and realistic images and videos can be created by the model. In fact, the large availability of presidents and Hollywood celebri- ty’s videos on social media can help individuals to produce realistic fake news and rumors that can bring a serious impact on our society. Recent studies show that deepfake video and images have become heavily cir- culated through social channels. Detection of deepfake videos and images, there- fore, has become increasingly critical and important. To encourage researchers, many organizations such as United States Defense Advanced Research Projects Agency (DARPA), Facebook Inc and Google launched a research initiative in attempting the detection and prevention of deepfake [6] [7]. As a result, many deep learning approaches such as long short-term memory (LSTM), recurrent neural network (RNN) and even the hybrid approaches has been proposed to in order to detect deepfakes images and videos and to bring up more research over this field [8] [9] [10] [11]. The current studies show that deep neural networks made a remarkable result in terms of detecting fake news and rumors in social media posts. This work primarily focuses on providing a comprehensive study for deepfake detection using deep-learning methods such as Recurrent Neural Network (RNN), Convolutional Neural Network (CNN), and Long short-term memory (LSTM). This survey will be useful and beneficial for researchers in this field as it will give: 1) details summary of the current research studies; 2) datasets used in this field; 3) the limitations of the current approaches and insights of future work. The contributions of our survey are summarized as follows. This is the first review that covers the current deep learning methods for deepfake detection and discusses their limitations. In this survey, we also cover all the challenges that are faced by the research- ers in this area and provide an outlook of future directions. In this review, we summarize and present available annotated datasets that are used in this field. The rest of the article is structured as follows: Section 2 summaries the related work, Section 3 contains deepfake Creation and deep learning Detection Tech- niques, Section 4 contains the public available dataset used in the Deepfake field, the challenges and the open issues are discussed in Section 5, Section 6 concludes the research. DOI: 10.4236/jcc.2021.95003 21 Journal of Computer and Communications A. M. Almars 2. Related Work 2.1. Basics of Artificial Neural Networks (ANNs) The basic concept of Artificial Neural Networks (ANNs) is partially inspired by how the human brain functions. Figure 1 shows artificial neural networks ar- chitecture. Neural networks are multi layers networks that consist of a single input layer, one or multi hidden layers and one output layers. The input to neur- al networks is a set of input values [12]. The goal of neural networks is to predict and classify those values into predefined categories. The first layer in neural network is the input layers which takes input values and pass them to the next layer [13]. In our example, the input values are x1, x2, x3 and x4. The second layer is the Hidden layers which a set of connected unites called artificial neurons (nodes). The edges that connect the neurons represents how all the neurons are interconnected and how can receive and send signals through multi layers. Each connection has a weight associated with it which represents the connections between two units. In our network, the 1st hidden layer consists of 3 neurons and the 2nd layer contains 4 neurons. Each neuron receives number of inputs from previous layer and a bias value. A bias value is an extra value which equal to 1. If a neuron has n inputs, it should have n weight values which can be represented by the following learning formula (Equations (1) and (2)): z= xw11 + xw 2 2 + xw 33 + + xwnn +∗ b 1 (1) = + z∑n=1 xwnn b (2) The third layer is the output layer which reads the output from previous layer and predicts the output values y1, y2 and y3. The goal of the learning and pre- dicting process is to adjust the connection weights between those units to reduce the error and predict the output values. Activation functions are used in neural = + network to determine the output values z∑n=1 xwnn b of the model. Acti- vation function aims to normalize the values into a smaller range. Equations (3), (4) and (5) are the most common activation functions used in neural network. 1 sigmoid( z) = (3) 1+− exp( z) Figure 1. Artificial neural networks architecture. DOI: 10.4236/jcc.2021.95003 22 Journal of Computer and Communications A. M. Almars eezz− − tanh ( z) = (4) eezz− − ReLU( zz) = Max( 0, ) (5) Sigmoid function Equation (3) is a widely used function that squashes values between a range [0, 1]. The tanh function Equation (4) is zero-centered which means it outputs values between [−1, 1] instead of [0, 1]. The rectified linear function (ReLU) Equation (5) is non-linear function that outputs the values di- rectly if positives, otherwise, it will outputs zero. Compared with the other func- tion’s methods, ReLU has become the defuel activation function for many ap- plications of neural networks as it easy to compute and fast to train. To predict the input values of neural networks, the input values should be faded in a forward direction. This process of feeding inputs in this way is called Forward propagation. Thus, each hidden layer in neural network reads the in- puts from previous layer, processes it thought the activation function and finally predicts the output values. Back propagation is a back forward process which aims at optimizing the weights to make sure that the neural network can cor- rectly predict the outputs. To achieve this, stochastic gradient descent is used to reduce the error cost. 2.2. Deep Learning Deep learning is a machine learning method based on the same idea of neural network [13] [14]. In deep learning, the word deep indicates the use of multiple hidden layers in the network. Inspired by artificial networks, the deep learning architecture uses an unbounded number of hidden layers of bounded size to ex- tract higher information from raw input data. The number of hidden layers is determined based on the complexity of the training data [6].
Details
-
File Typepdf
-
Upload Time-
-
Content LanguagesEnglish
-
Upload UserAnonymous/Not logged-in
-
File Pages16 Page
-
File Size-