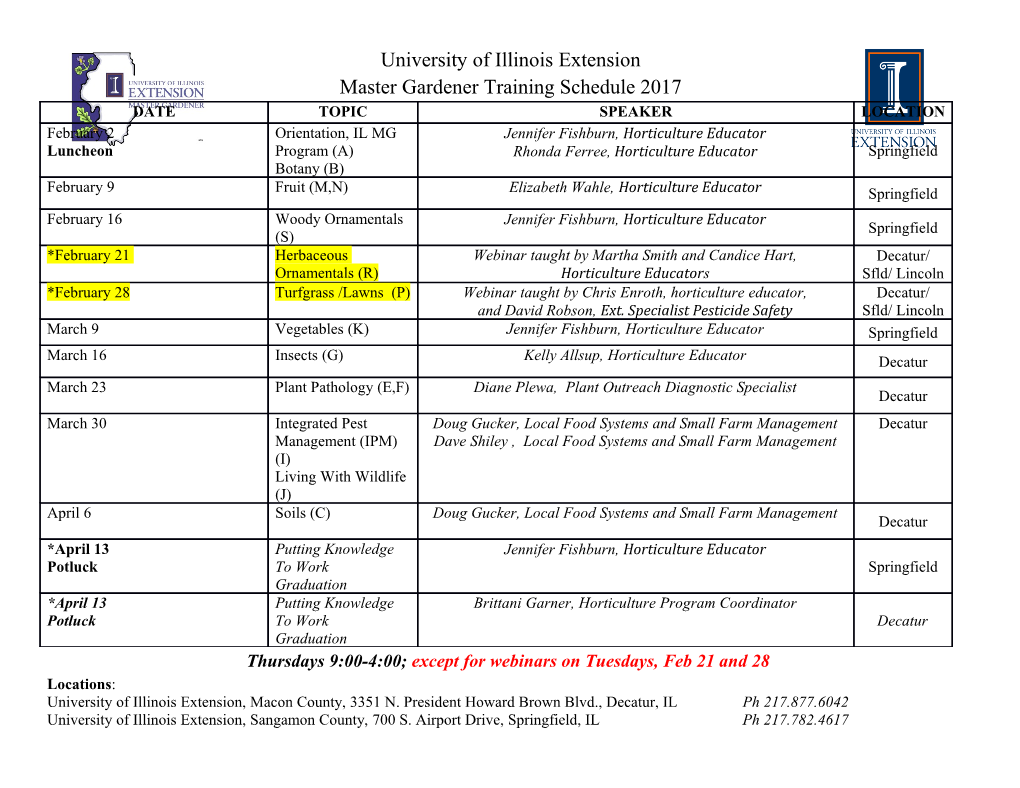
JOURNAL OF INDUSTRIAL AND doi:10.3934/jimo.2021122 MANAGEMENT OPTIMIZATION AN EXACT ALGORITHM FOR STABLE INSTANCES OF THE k-MEANS PROBLEM WITH PENALTIES IN FIXED-DIMENSIONAL EUCLIDEAN SPACE Fan Yuan Department of Operations Research and Information Engineering Beijing University of Technology, Beijing 100124, China Dachuan Xu∗ Beijing Institute for Scientific and Engineering Computing Beijing University of Technology, Beijing 100124, China Donglei Du Faculty of Management University of New Brunswick, Fredericton, NB Canada E3B 5A3, Canada Min Li School of Mathematics and Statistics Shandong Normal University, Jinan 250014, China (Communicated by Wenxun Xing) Abstract. We study stable instances of the k-means problem with penalties in fixed-dimensional Euclidean space. An instance of the problem is called α-stable if this instance exists a sole optimal solution and the solution keeps unchanged when distances and penalty costs are scaled by a factor of no more than α. Stable instances of clustering problem have been used to explain why certain heuristic algorithms with poor theoretical guarantees perform quite well in practical. For any fixed > 0, we show that when using a common multi- swap local-search algorithm, a (1 + )-stable instance of the k-means problem with penalties in fixed-dimensional Euclidean space can be solved accurately in polynomial time. 1. Introduction. For many optimization problems, certain well-known heuristic algorithms perform much better than what their theoretical performance guarantee suggests. To explain this paradox, many existent works seek to study their sta- ble instances, a concept introduced in Bilu et al. [8] and Awasthi et al. [5]. An instance of a problem is called α-stable if its optimal solution is sole and remains unchanged even if the problem's input parameters are scaled by at most an α factor. An instance of a clustering problem is called α-stable if this instance exist a sole optimal solution and this sole optimal solution remains unchanged after some dis- tances of this instance are scaled by α and distances between any two points can be 2020 Mathematics Subject Classification. Primary: 90C27; Secondary: 68W25. Key words and phrases. Local search, stable instance, k-means, approximation algorithm, fixed-dimensional Euclidean space. ∗ Corresponding author: Dachuan Xu. 1 2 FAN YUAN, DACHUAN XU, DONGLEI DU AND MIN LI scaled differently. The motivation to study stable instances is that, for some com- mon clustering problems, certain well-know heuristics are actually polynomial-time algorithms for α-stable instances of these problems, and hence offering one explana- tion of the aforementioned paradox as instances encountered in practice may very well be α-stable. Before we formally introduce the problem we are interested in this work, we review relevant literature. Clustering problems have been studied since the 1950's in many fields of science: biomedical engineering, statistical science, medical engineering, computer science, physical information engineering, and more. According to the different objective functions, many different kinds of clustering problems are generated. The most common one is the k-means problem. This problem has been studied by many scholars due to its wide application value. In this problem we are given a set D of n points in d-dimensional Euclidean space Rd, and an integer k. Our target is to d pick a set of points f1,...,fk2R with size k and assign them as center points. We want to minimize the sum of squared distances of each point to its nearest center point. However, the time complexity of selecting the center points from the Rd space is too large, so a lot of work studies the discrete version of the k-means problem. When the centres must be chosen from a given finite set F , we call it a discrete k-means problem. Matouˇsek[22] show that a discrete k-means problem can be transformed by a k-means problem with a small loss. It is well known that the discrete k-means problem is NP-hard for k = 2 in Rd when d is not a constant. Again, in the case of arbitrary k in R2 [2, 11, 21] the discrete k-means problem is NP-hard too. There are quite a lot of research results for this problem in literature. For arbitrary dimensions, Kanungo et al. [17] present a 9 + local search algorithm, and the best ratio 6.357 is given by Ahmadian et al. [1]. For fixed dimensions, a PTAS is given independently by Friggstad et al. [15] and Cohen-Addad et al. [10]. Our problem is closely related to the k-means problem with penalties in fixed dimension Euclidean space. The k-means problem with penalties is a natural gen- eralization of the classic k-means problem. Formally, the problem gives us a client point set D of n client points in d-dimensional Euclidean space Rd, a penalty cost pj > 0 for every client point j 2 D. This problem also give us a positive integer k ≤ n that represents the size of the set of centers. Our target is to find a set of d k points f1,...,fk2R to be the centers and find a client subset P ⊆ D to be the penalized client set so as to minimize the sum of squared distances of each client point in DnP to its nearest center and the sum of penalty costs for each client point in P . Tseng [23] proposed the penalized and weighted k-means problem with uni- form penalties. Zhang et al. [26] proposed the k-means problem with nonuniform penalties and give a (25 + )-approximation local search algorithm for this problem. The best result on this problem so far is a (19:849 + )-approximation primal-dual algorithm, which was proposed by Feng et al. [13]. Li et al. [19] design its approxi- mation algorithm by initializing the first clustering. Based on this algorithm, Li [18] presents a bi-criteria algorithm for k-means with penalties. Ji et al. [16] generalize the seeding algorithm to the variants of k-means problem with penalties. The main model studied in this work is the discrete k-means problem with penal- ties (k-MPWP). In this problem we are given a center point set F in Rd and a data point set D in Rd. We now formally define what a stable instance is for this prob- lem. Denote (F; D; η; p) as an instance of the k-means problem with penalties. η is AN EXACT ALGORITHM FOR STABLE INSTANCES 3 a metric distance between points in F [ D. A center point set S ⊆ F with j S j= k together with a penalized client set P ⊆ D is an solution of this problem. For any two points i; j 2 Rd, we use η(i; j) to define the distance between them. For every set S ⊆ F , and every j 2 D, denote η(S; j) := mini2S η(i; j). The cost of solution S is X 2 X cost(S) := η(S; j) + pj: j2DnP j2P For this problem, our target is to choose a best S ⊆ F to minimize the cost(S). For any feasible center set S of the k-MPWP, the corresponding penalty set P is 2 always chosen as P = j 2 D j pj < η(S; j) , implying that the penalty set P is 2 determined by the center set S. Hence if we set penalty cost pj > η(S; j) for all points j 2 D, then the k-MPWP is degenerated to the classic k-means problem. In this situation the penalty set P is empty. Definition 1.1. (α-stability). For a given constant α ≥ 1, we call an instance I = (F; D; η; p) of the metric k-means problem with penalties is α-stable if this instance exist a sole optimal solution O. This solution O is still the sole optimal solution in 0 0 0 0 all other related instances I = (F; D; η ; pj) with η(i; j) ≤ η (i; j) ≤ α · η(i; j) and 0 0 pj ≤ pj ≤ α · pj for all i; j 2 F [ D. (The distance function η only needs to satisfy symmetry and does not need to satisfy the triangle inequality.) For a large number of clustering problems, many people have studied how to solve their stable instance. Their target is to find polynomial-time exact algorithms for small α. Awasthi et al. [6] show that for a 3-stable instance of the k-means problem, we can find the optimal solution of the instancep in polynomial time. A few years later Balcan and Liang [7] show that for a (1+ 2)-stable instance of the k- means problems, we can find the optimal solution of the instance in polynomial time. Agelidakis et al. [3] show that for a 2-metric stable instance of the k-means problems, we can find the optimal solution of the instance in polynomial time. Friggstad et al. [14] study the stable instances of k-means problem in fixed-dimensional Euclidean space and prove that for any fixed > 0, for a (1+)-stable instance of the k-means problem, the optimal solution can be obtained in polynomial time. In combinatorial optimization, a widely used technique is called Local search technique. Local search technique is used to solve many problems, such as k-means problems [15,26], arc routing problems [20,24], facility location problems [4,9,12,25], multicoloring hexagonal graphs [27], and. In this work, we focus on a discrete k- means problem with penalties in fixed-dimensional Euclidean space. Our results show that for any fixed > 0, for a (1 + )-stable instance of this problem in fixed dimension Euclidean space (Rd, d is a fixed constant), the optimal solution of this instance can be obtained in polynomial time by using local search techniques.
Details
-
File Typepdf
-
Upload Time-
-
Content LanguagesEnglish
-
Upload UserAnonymous/Not logged-in
-
File Pages12 Page
-
File Size-