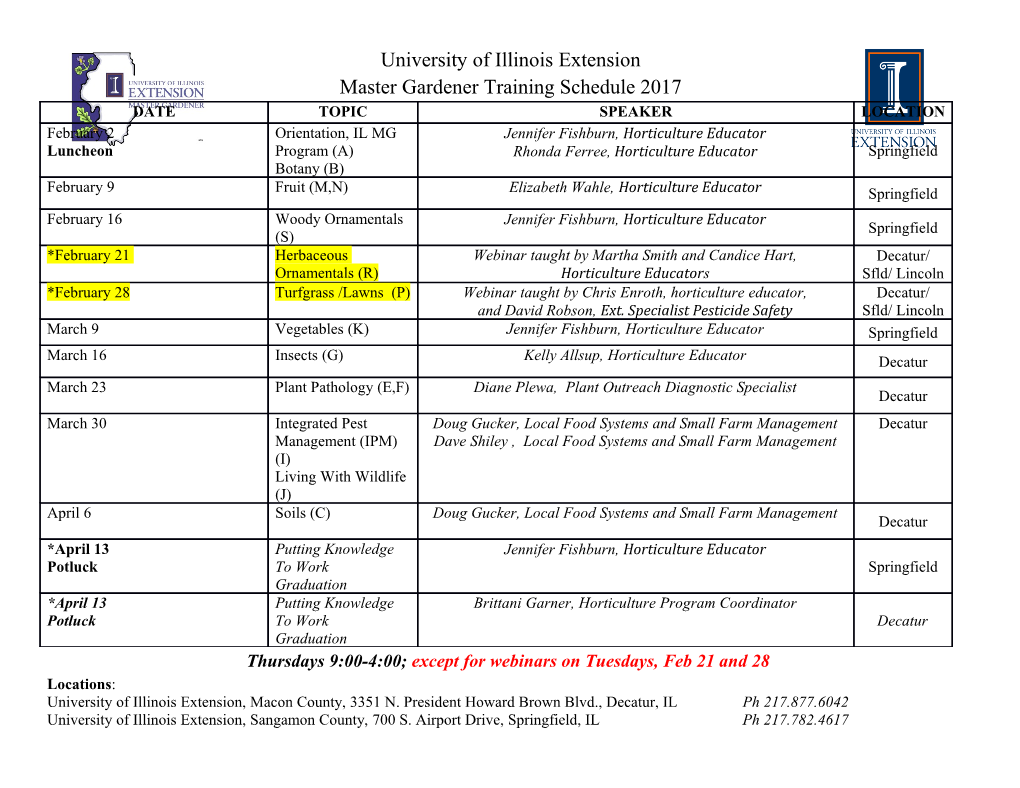
PAD5700 week three MASTER OF PUBLIC ADMINISTRATION PROGRAM PAD 5700 -- Statistics for public management Fall 2013 Sampling and confidence intervals Statistic of the week ̅ √ The standard deviation * We start with some discussion of data gathering. There is an old cliché in statistics (or any sort of analytical process) that if you put 'junk' in, you will get 'junk' out. Think of the recent collapse of the US economy, and as a result global economy: no one (effectively, click here and here for exceptions) saw it coming. The Bush administration's 2009 budget, for instance, was released early in 2008. In the Budget Message, the President confidently stated that "As we enter this New Year, our economy retains a solid foundation despite some challenges, revenues have reached record levels, and we have reduced the Federal deficit by $250 billion since 2004." In Table S-10 of the Summary Tables, the administration foresaw economic growth ('Real GDP' in the table) of 2.7% in 2008, followed by 3.0% growth in 2009. It wasn't just the Bush administration that was delusional. As this table also indicated, the CBO (Congressional Budget Office, widely respected as a competent, and unbiased referee in budget debates) expected growth of 1.7% and 2.8% for these two years, while the 'Blue Chip consensus' (the mean prediction of a number of highly respected private economic forecasters) was about halfway between the two. The actual result for 2008 was 1.0% growth, while 2009 saw about a 3.5% contraction in the economy. Why? Because the data that they plugged into their economic models was faulty. While numbers can be a very good way to describe reality (as we saw last week), statistics can be very bad at prediction, and even worse at predicting unprecedented events. The logic of statistics is that you plug past data into a dataset, develop a model to analyse it, then punch 'run'. It spits out a result. However if the future diverges sharply from the past -- if something fundamental changes -- by definition your past data will not have incorporated this. Indeed, even your model can be wrong. These same problems can occur even if the future doesn't reflect a break from the past, but the analyst fails to sample the data correctly. Imagine conducting a survey about voting preferences of Americans. If you hung out at Ponte Vedra and asked passersby you'd probably get a different result than if you hung out on the east side of Jacksonville. For two similar examples: how would you react if I told you that: 1. I played top 20 college hoops? 2. I placed second in a national championship 10k foot race? Page 1 of 13 PAD5700 week three Ethics On a similar note, it is widely asserted that there are lies, damned lies, and statistics. Not so. Numbers don’t lie, but people lie about numbers. People are also often clueless about numbers. Being clueless and wanting to lie are mutually reinforcing, too, as it is easier for those who want to use numbers to lie, to do so if they don’t know what they’re doing. So note Berman & Wang’s short section on ethics (pp. 10-15). For me, if the numbers don’t support what I think is reality, I’ll generally check the numbers. But if the numbers look legit, I flip-flop, or change my views. Sampling Statistics is driven largely by the concept of sampling. At its simplest, sampling refers to a process of measuring what is going on in a part of a larger population, to determine what is going on in the entire population. One might fairly ask: Q. Why not just measure the entire population? A. Because this can be difficult. Note the 2000 US federal election in Florida. Who won? Well, Governor Bush did, he got the electoral votes, and became President. But what I mean is: who got the most votes? We don't know who got the most votes, we will probably never know, and frankly the point is that given human (and technical) imperfection, in any election that is that close in an electorate that is that large, we probably can’t measure the vote exactly. It can even be difficult to measure smaller populations. Take an issue that is near and dear to most people: seals in Labrador. Assume you want to monitor their weight. Once you set up the scales, how do you get all of the little blighters to turn up to be weighed? Instead, you have to go out and catch them. You may not get them all, if you don't, maybe you missed the leaner, quicker ones, and so your sample is biased by an over-representation of the seal equivalent of couch potatoes (or perhaps: cod potatoes?). Again: measuring a population can be difficult. The discussion so far also serves to introduce some technical jargon: Observation -- the individual unit of a sample, population of 'random variable' (e.g. the individual MPA program) Sample -- a random (or otherwise representative) portion of a population or random variable (e.g. a random selection of every tenth MPA program) Population -- the entire set of individual observations of interest (MPA programs in the US) Random variable -- the underlying social/historical/biological/etc process generating the individual observations in a population (the range of possible MPA program outcomes). From Berman & Wang: Hypothesis – what we think might be going on, and so want to test for. o Theory – one of the least understood words in the (American) English language. See an online dictionary definition. In the social sciences, #1, 3, 5 are what we refer to as theory. #2, 4 and 6 are what we refer to as hypotheses. o Dependent variable – what we are trying to explain o Independent variable – what we think explains variation in the dependent variable, and so otherwise referred to as ‘explanatory’ variables. Page 2 of 13 PAD5700 week three o Key independent variable – often there is a single variable whose effect on the dependent variable particularly interests us. o Control variables – other variables that are added to the model to hold these constant, so that the independent effect of the key independent variable can be ascertained. o Example – we saw this in our discussion of week one of determinants of good socio- economic outcomes. In Figure 2 and Table 3, economic freedom has a strong, positive effect on socio-economic outcomes (specifically: ‘human development’). Yet when public services (an indicator of good government) are introduced to the model, the impact of economic freedom dissipates. We will try to tease out this relationship as the course goes on, but for now let’s hypothesize that the relationship is a complex one: economic freedom and good public services are necessary for improved health, education and income; and these, in turn, make the provision of public services (and realization of economic freedom?) possible. o Correlation or association – this is implied by causation, below. In correlation, A and B co- vary: as one changes, the other does, as well. o Causal relationships – a change in A results in a change in B. Berman and Wang’s take on this: “Causation requires both empirical (that is, statistical) correlation and (2) a plausible cause-and-effect argument” (p. 25). o Target population and sampling frame o Unit of analysis o Sampling error -- random error, an inevitable part of sampling o Sampling bias -- systematic error, resulting from a conceptual mistake in sampling Six steps to research design (Berman & Wang, p. 28). 1. Define the activity and goals that are to be evaluated. 2. Identify which key relationships will be studied. 3. Determine the research design that will be used. 4. Define and measure study concepts. 5. Collect and analyze the data. 6. Present study findings. Sampling designs: o Simple randomness -- the functional equivalent of a lottery. o Stratified random sampling -- cheat a little, by holding mini lotteries within known important sub-sections of the population, to try to overcome the inevitable sampling bias resulting from typically unrepresentative rates of non-responses. Re-weighting – On sampling bias, above, when random sampling yields a sample that does not share known characteristics of the broader population, under-represented groups are re- weighted, with their results multiplied by an appropriate figure: if left-handed leftwing lesbian Luddite Lebanese Lutherans make up 5% of your population, but only 2.5% of the people who responded to your survey; simply count these responses twice. o Disproportionate stratified sampling -- drawing more people from especially small, but especially important groups, to ensure that a large enough sub-sample is drawn from this group to allow for statistically significant results. o 'Cluster' sampling -- geographical units essentially become the unit of analysis in the sampling process, with these randomly selected. o Convenience sampling -- talk to whoever walks by 1st and Main while the longsuffering intern is standing there conducting interviews. Page 3 of 13 PAD5700 week three o Purposive sampling -- want to know what teens think? Don't hang out at the vets club. o Quota sampling -- not unlike stratified random sampling. o Snowball sampling -- follow referrals. Sampling distributions We now come to a key transition in the conceptual understanding of this stuff. The idea here is to estimate the population mean (N). If we could directly measure N, we wouldn't bother sampling. After all, no one cares what a random sample of 600 people thinks about anything, no one even knows who those 600 or so people are. The only reason we ask 600 randomly selected people what they think about stuff is because we really want to know what society thinks about these issues, but it is too difficult to discern the opinion of all of society (see the earlier discussion of the 2000 election, for instance).
Details
-
File Typepdf
-
Upload Time-
-
Content LanguagesEnglish
-
Upload UserAnonymous/Not logged-in
-
File Pages13 Page
-
File Size-