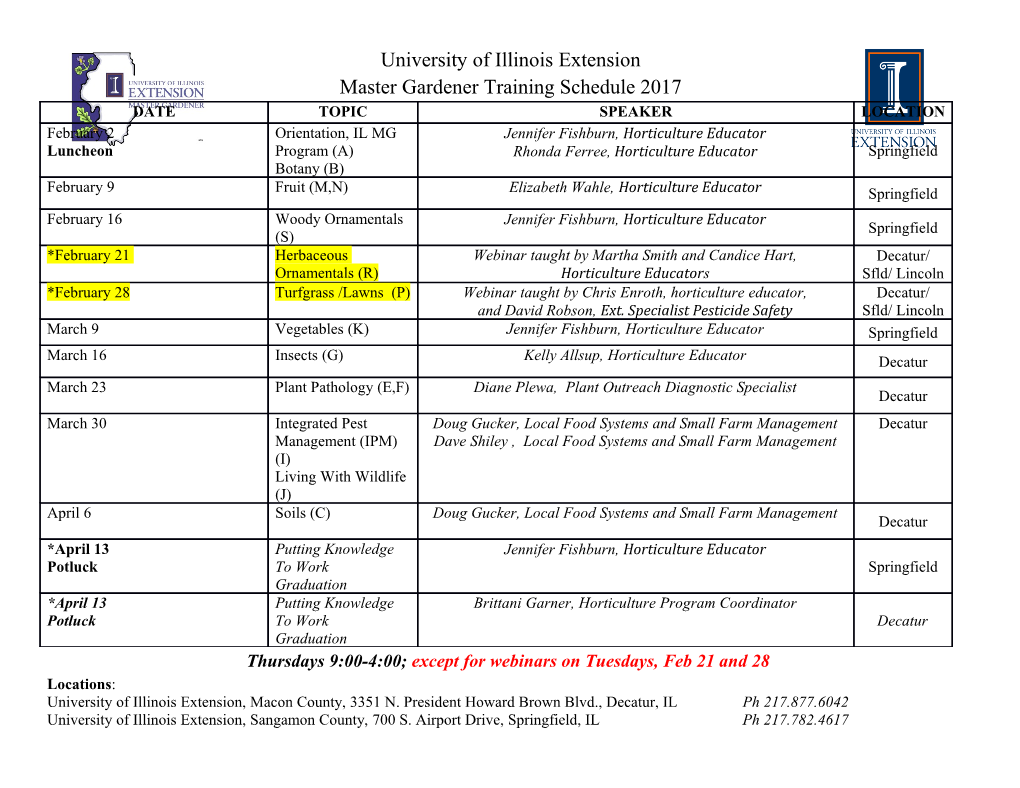
Restricted Isometry Constants in Compressed Sensing Bubacarr Bah Doctor of Philosophy University of Edinburgh 2012 Declaration I declare that this thesis was composed by myself and that the work contained therein is my own, except where explicitly stated otherwise in the text. (Bubacarr Bah) 3 To my grandmother, Mama Juma Sowe. 4 Acknowledgement I wish to express my deepest gratitude to my supervisor, Professor Jared Tanner, for his guid- ance and support during the course of my PhD. I would follow this with special thanks to my second supervisor, Dr. Coralia Cartis, for her regular monitoring of my progress and her encouragement. Further special thanks to my sponsor the University of Edinburgh through the MTEM Scholarships created in 2007 by the Principal, Professor Sir Timothy O’Shea. My sincere gratitude goes to Professor Endre Suli of Oxford University who informed me of, and recommended me for, this PhD opening with Professor Jared Tanner and also to my MSc dissertation supervisor, Dr. Radek Erban of Oxford University, for recommending me for this PhD programme. Many thanks to both the admin and support staff of the School of Mathematics, University of Edinburgh, that contributed to making my PhD programme proceed smoothly. Similar thanks go to members of the Mathematics Teaching Organisation of the school. I would like to thank the following people for contributing to my research either by responding to a question I posed, discussing an issue pertaining to my research or giving me feedback on my research: Dennis Amelunxen, Jeff Blanchard, Burak Burke, Prof. David Donoho, Martin Lotz, Andrew Thompson, Ke Wei, Pavel Zhlobich. I would also like to thank my officemates not only for the cordial company but for the occasional research related discussions. I wish to thank members of the Applied and Computational Mathematics research group of the school and members of the Edinburgh Compressed Sensing Reading group. I wish to also thank the first and second set of officials of the Edinburgh SIAM Student Chapter whom I worked with to run the chapter. Similarly, my thanks go to my friends in Edinburgh that made my stay in the city comfortable and also friends and family in Scotland and England for the moral support. I would also like to thank the staff of the Institute of Mathematics and its Applications (IMA) at Minnesota who facilitated my visit to the IMA and my Gambian hosts and friends in Minnesota during this visit. My gratitude goes to my family and to all my friends and well wishers for their unflinching support and encouragement. Above all, special thanks to my parents and wife for the invaluable support. 5 6 Bubacarr Bah 6 Abstract Compressed Sensing (CS) is a framework where we measure data through a non-adaptive linear mapping with far fewer measurements that the ambient dimension of the data. This is made possible by the exploitation of the inherent structure (simplicity) in the data being measured. The central issues in this framework is the design and analysis of the measurement operator (matrix) and recovery algorithms. Restricted isometry constants (RIC) of the measurement matrix are the most widely used tool for the analysis of CS recovery algorithms. The addition of the subscripts 1 and 2 below reflects the two RIC variants developed in the CS literature, they refer to the ℓ1-norm and ℓ2-norm respectively. The RIC2 of a matrix A measures how close to an isometry is the action of A on vectors with few nonzero entries, measured in the ℓ2-norm. This, and related quantities, provide a mechanism by which standard eigen-analysis can be applied to topics relying on sparsity. Specifically, the upper and lower RIC of a matrix A of size n N is the maximum and the minimum 2 × deviation from unity (one) of the largest and smallest, respectively, square of singular values of N all k matrices formed by taking k columns from A. Calculation of the RIC2 is intractable for most matrices due to its combinatorial nature; however, many random matrices typically have bounded RIC2 in some range of problem sizes (k,n,N). We provide the best known bound on the RIC2 for Gaussian matrices, which is also the smallest known bound on the RIC2 for any large rectangular matrix. Our results are built on the prior bounds of Blanchard, Cartis, and Tanner in Compressed Sensing: How sharp is the Restricted Isometry Property?, with improvements achieved by grouping submatrices that share a substantial number of columns. RIC2 bounds have been presented for a variety of random matrices, matrix dimensions and sparsity ranges. We provide explicit formulae for RIC bounds, of n N Gaussian matrices 2 × with sparsity k, in three settings: a) n/N fixed and k/n approaching zero, b) k/n fixed and n/N approaching zero, and c) n/N approaching zero with k/n decaying inverse logarithmically in N/n; in these three settings the RICs a) decay to zero, b) become unbounded (or approach inherent bounds), and c) approach a non-zero constant. Implications of these results for RIC2 based analysis of CS algorithms are presented. The RIC2 of sparse mean zero random matrices can be bounded by using concentration bounds of Gaussian matrices. However, this RIC2 approach does not capture the benefits of 7 8 Bubacarr Bah the sparse matrices, and in so doing gives pessimistic bounds. RIC1 is a variant of RIC2 where the nearness to an isometry is measured in the ℓ1-norm, which is both able to better capture the structure of sparse matrices and allows for the analysis of non-mean zero matrices. We consider a probabilistic construction of sparse random matrices where each column has a fixed number of nonzeros whose row indices are drawn uniformly at random. These matrices have a one-to-one correspondence with the adjacency matrices of fixed left degree expander graphs. We present formulae for the expected cardinality of the set of neighbours for these graphs, and present a tail bound on the probability that this cardinality will be less than the expected value. Deducible from this bound is a similar bound for the expansion of the graph which is of interest in many applications. These bounds are derived through a more detailed analysis of collisions in unions of sets using a dyadic splitting technique. This bound allows for quantitative sampling theorems on existence of expander graphs and the sparse random matrices we consider and also quantitative CS sampling theorems when using sparse non mean- zero measurement matrices. 8 Contents Abstract 8 1 Introduction 15 1.1 RestrictedIsometryConstants(RIC) . ....... 16 1.1.1 Euclidean Norm Restricted Isometry Constants (RIC2) .......... 16 1.1.2 Manhattan Norm Restricted Isometry Constants (RIC1).......... 20 1.2 RandomMatrixTheoryofGaussianMatrices . .... 20 1.2.1 RandomMatrixTheoryEnsembles. 21 1.2.2 Extreme Eigenvalues of Gaussian and Wishart Matrices . ... 23 1.3 SparseRandomMatricesandExpanderGraphs . ...... 28 1.4 Motivating Example – Compressed Sensing . ... 32 1.4.1 MathematicsofCompressedSensing . 33 1.4.2 Applications of Compressed Sensing . 38 1.5 Conclusion ....................................... 38 2 ExtremeEigenvaluesofGaussianSubmatrices 41 2.1 Introduction...................................... 41 2.2 Prior RIC2 Bounds................................... 42 2.3 Improved RIC2 Bounds ................................ 44 2.3.1 Discussion on the Construction of Improved RIC2 Bounds. 47 2.4 Finite N Interpretations................................ 50 2.5 Implications for Compressed Sensing . .... 52 2.5.1 Phase Transitions of Compressed Sensing Algorithms . .... 53 2.6 Proofs.......................................... 58 2.6.1 Lemma2.3.5 .................................. 58 2.6.2 Theorem 2.3.2 and Propositions 2.4.1 and 2.4.2 . 59 3 Asymptotic Expansion of RIC2 for Gaussian Matrices 65 3.1 Introduction...................................... 65 3.2 Asymptotic Formulae for RIC2 Bounds........................ 65 3.3 Implications for Compressed Sensing . .... 68 3.4 AccuracyofMainResults(Formulae) . ... 69 3.4.1 Theorems 3.2.1: δ fixed and ρ 1...................... 69 ≪ 3.4.2 Theorems 3.2.2: ρ fixed and δ 1...................... 70 ≪ 1 3.4.3 Theorems 3.2.3: ρ = (γ log(1/δ))− and δ 1................ 70 ≪ 3.5 Proof of Compressed Sensing Corollaries . ..... 71 3.5.1 Proof of Corollary 3.3.1 . 72 3.5.2 Proof of Corollary 3.3.2 . 72 3.6 Proofs of Theorems 3.2.1 - 3.2.3 and Corollary 3.2.4 . ... 72 3.6.1 Theorem3.2.1 ................................. 74 3.6.2 Theorem3.2.2 ................................. 81 3.6.3 Theorem3.2.3 ................................. 88 3.6.4 Corollary 3.2.4 . 96 9 10 Bubacarr Bah 4 Sparse Matrices and Expander Graphs, with Application to Compressed Sensing 99 4.1 Introduction...................................... 99 4.2 SparseMatricesandExpanderGraphs . .100 4.2.1 MainResults ..................................100 4.2.2 Implications of RIC1 for Compressed Sensing and Expanders . 102 4.3 Discussion and Derivation of the Main Results . .103 4.3.1 Discussion of Main Results . 104 4.3.2 KeyLemmas ..................................106 4.4 Implications for Compressed Sensing Algorithms . .115 4.4.1 Performance Guarantees of Compressed Sensing Algorithms . .......115 4.4.2 Quantitative Comparisons of Compressed Sensing Algorithms . .118 4.5 Proofs..........................................119 4.5.1 Theorem 4.2.4 - Examples of Small Problems . 119 4.5.2 Theorem 4.2.4 for Large s ...........................126 4.5.3 Theorem4.2.6 .................................132 4.5.4 Main Corollaries . 135 5 Conclusions 139 5.1 Summary ........................................139 5.2 PossibleExtensions.................................. 144 10 List of Figures 1.1 The largest and smallest eigenvalues of a Wishart matrix . ..... 18 1.2 Illustration of a lossless (k,d,ǫ)-expandergraph. 29 2.1 CT bounds, UCT (δ, ρ) and LCT (δ, ρ), for (δ, ρ) (0, 1)2 .............. 43 2.2 BCT bounds, UBCT (δ, ρ) and LBCT (δ, ρ), for (δ,∈ ρ) (0, 1)2 ............ 44 2.3 BT bounds, UBT (δ, ρ) and LBT (δ, ρ), for (δ, ρ) (0,∈1)2 .............
Details
-
File Typepdf
-
Upload Time-
-
Content LanguagesEnglish
-
Upload UserAnonymous/Not logged-in
-
File Pages154 Page
-
File Size-