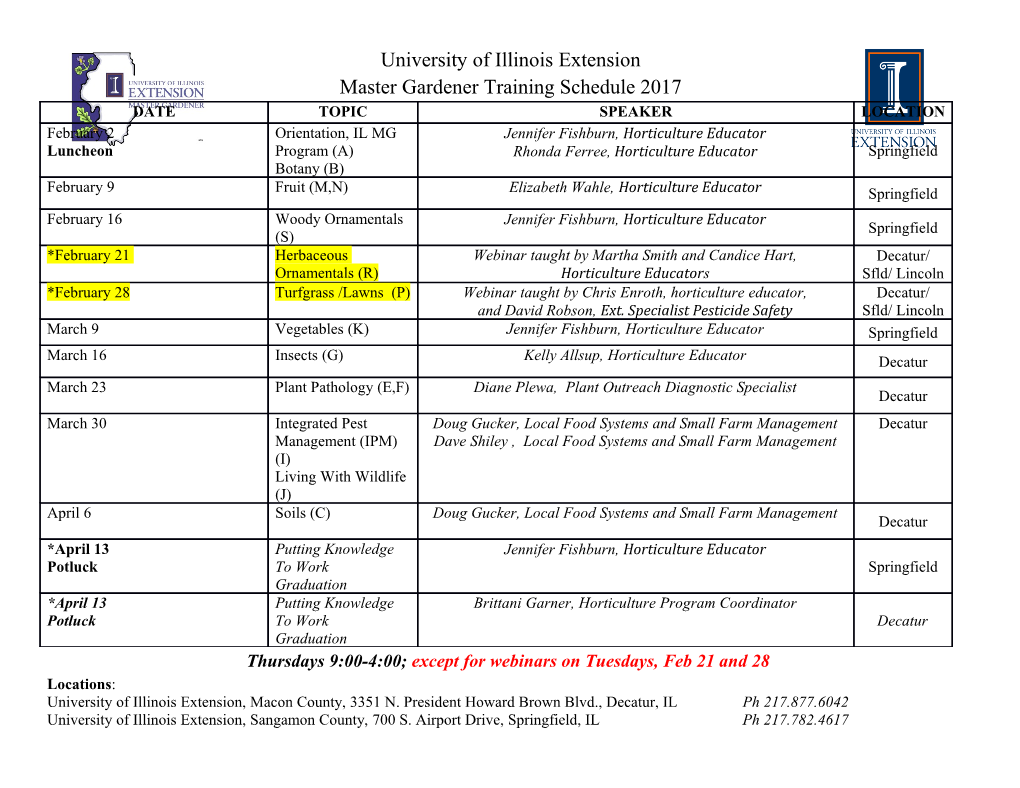
Rational Vaccine Design by Reverse & Structural Vaccinology and Ontology by Edison Ong A dissertation submitted in partial fulfillment of the requirements for the degree of Doctor of Philosophy (Bioinformatics) in the University of Michigan 2021 Doctoral Committee: Associate Professor Yongqun He, Chair Professor Denise Kirschner Professor Kayvan Najarian Associate Professor Zhenhua Yang Professor Yang Zhang Edison Ong [email protected] ORCID iD: 0000-0002-5159-414X © Edison Ong 2021 Acknowledgments First of all, I would like to acknowledge my advisor Dr. Yongqun (Oliver) He, for his admirable mentorship. He trained me to be a motivated and well-organized person throughout my pursuit of the doctoral program. I appreciate the opportunity to work in his laboratory and contribute to scientific research. With his support, I designed and completed many projects, resulting in multiple publications. I feel thankful that he showed me how to be independent and creative to all the possibilities as a scientist. My special thanks go to the former Dr. He laboratory members, Dr. Sirarat Sarntivijai, Dr. Asiyah Yu Lin, Dr. Rebecca Racz, and Zuoshuang Xiang, for their supports and advice that are valuable throughout my dissertation work. The committee member's help and guidance have maximized my potential to finish the dissertation. They supported my interdisciplinary research involving machine learning, structural biology, microbiology, immunology, and epidemiology. My sincere thanks go to Dr. Zhenhua Yang, and she provided me many grateful supports. She showed her enthusiasm for science and gave me indispensable advice for my project and future career. Dr. Yang offered me many collaboration opportunities in tuberculosis related projects. In particular, Dr. Yang introduced me to work with the Michigan Department of Health & Human Services (MDHHS) to process Mycobacterium tuberculosis whole-genome sequence data. I also want to thank Dr. Marty Soehnlen and the MDHHS laboratory staff for their warm supports. I would like to recognize the invaluable assistance that Dr. Yang Zhang has given to me on structural biology and its application in vaccine design. Dr. Zhang has provided much valuable advice that is critical to my dissertation work. I would also like to thank Dr. Xiaoqiang Huang and Mr. Robin Pearce for their huge contribution to my structural vaccinology work. I wish to show my gratitude to Dr. Kayvan Najarian and Dr. Denise Kirschner for their insightful advice in machine learning and tuberculosis studies. Dr. Najarian offered his knowledgeable machine learning experience and guided me throughout my design of machine learning-based reverse vaccinology work. Dr. Kirschner provided many positive and warm suggestions that facilitate understanding in immunology and professional networking in the field. I would like to express my gratitude to the Department of Computational Medicine and Bioinformatics and Unit for Laboratory Animal Medicine (ULAM), the advisors and staff, Dr. Margit Burmeister, Dr. Maureen Sartor, Dr. Robert C. Dysko, and Dr. Jeffrey de Wet, and Julia Eussen. I appreciate the financial support from the National Institutes of Health (NIH) Integrated Network-based Cellular Signatures (LINCS), National Institute of Diabetes and Digestive and Kidney Diseases (NIDDK) Kidney Precision Medicine Project (KPMP), National Institute of Allergy and Infectious Diseases (NIAID) Immunology Database and Analysis Portal (ImmPort), and University of Michigan Rackham Predoctoral Fellowship and ULAM bridge fund. I also appreciate the European Bioinformatics Institute's support and funding during my summer internship in the United Kingdom in 2017. I am grateful that Dr. Giovanni Paternostro and Dr. Carlos Piermarocchi offered me a research programmer position after my undergraduate degree. With my valuable bioinformatics research experience throughout my two years of work, I eventually pursued a doctoral degree in Bioinformatics. The experience of the whole Ph.D. and writing the dissertation is not easy for me, and I appreciate the help from all the people around me. I would like to show my deepest gratitude to my wife, Mei U, who has been a great accompany in my journey. We met each other in kindergarten, and it was her encouragement leading to my passion for biology. We have been supporting, encouraging, and educating each other from high school to university. She has been, and always will be, my Sirius in the night. I would also thank my aunts, Elisa and Kate, who fully supported me when I decided to apply for a doctoral degree. They provided me with everything needed to succeed in my academic and career. Finally, I would like to dedicate my dissertation to my mother, Emily, who inspired my scientific curiosity. She always gave me numerous supports and precious memory in my childhood. I wish she would be here to see me finish the doctoral degree. Still, I am assured that she will be proud of her boy being a diligent and dedicated person. Table of Contents Acknowledgments 3 List of Tables vi List of Figures 8 List of Abbreviations xi Abstract xiv Chapter 1 Introduction 1 1.1 Challenges in Vaccine-preventable Diseases and Outbreaks 2 1.2 Immunity and Vaccines 3 1.3 Reverse Vaccinology 5 1.4 Structural Vaccinology 10 1.5 Vaccine-informatics and Ontology 14 1.6 Dissertation Outline 16 Chapter 2 Identification of New Features from Known Bacterial Protective Vaccine Antigens Enhances Rational Vaccine Design 18 2.1 Abstract 18 2.2 Introduction 18 2.3 Methods 20 2.5.1 Protective Antigens and Background Pan-proteome Non-Protective Protein Sequences 20 2.5.2 Protein Properties Computations 21 2.5.3 Protein Sequence Properties Computations 23 2.5.4 Statistical Analysis 24 ii 2.4 Results 24 2.3.1 Collection of Protective Vaccine Antigens, Background, and Non-protective proteins 24 2.3.2 Subcellular Localization (SCL) Analysis 25 2.3.3 Adhesin Probability (AP) Analysis 29 2.3.4 Transmembrane α-helix (TMH) and β-barrel (TMB) 34 2.3.5 Conserved Domain Analysis 34 2.3.6 Functional Analysis 37 2.5 Discussion 40 2.6 Acknowledgement 45 Chapter 3 Vaxign-ML: Supervised Machine Learning Reverse Vaccinology Model for Improved Prediction of Bacterial Protective Antigens 47 3.1 Abstract 47 3.2 Introduction 47 3.3 Methods 52 3.5.1 Data Preparation 52 3.5.2 Supervised machine learning classification 54 3.5.3 Benchmarking and evaluation with an independent dataset 56 3.4 Results 58 3.3.1 Effect of data resampling strategies on the classification 58 3.3.2 Extreme gradient boosting as the best performing model 62 3.3.3 Biological and physicochemical features in Vaxign-ML 66 3.3.4 Benchmarking Vaxign-ML 66 3.3.5 Vaxign-ML predicting current clinical trial or licensed vaccines 69 3.5 Discussion 71 3.6 Acknowledgement 73 Chapter 4 COVID-19 Coronavirus Vaccine Design Using Reverse Vaccinology and Machine Learning 75 4.1 Abstract 75 4.2 Introduction 76 4.3 Methods 81 iii 4.3.1 Vaxign and Vaxign-ML Reverse Vaccinology Prediction 81 4.3.2 Phylogenetic Analysis 82 4.3.3 Immunogenicity Analysis 83 4.4 Results 85 4.4.1 SARS-CoV-2 N protein sequence is conserved with the N protein from SARS-CoV and MERS-CoV 85 4.4.2 Six adhesive proteins in SARS-CoV-2 identified as potential vaccine targets 86 4.4.3 Three adhesin proteins were predicted to induce strong protective immunity 88 4.4.4 Nsp3 as a vaccine candidate 91 4.5 Discussion 102 4.6 Acknowledgement 106 Chapter 5 Computational Design of SARS-CoV-2 Spike Glycoproteins to Increase Immunogenicity by T Cell Epitope Engineering 108 5.1 Abstract 108 5.2 Introduction 109 5.3 Methods 112 5.3.1 Computational redesign of SARS-CoV-2 S protein 112 5.3.2 MHC-II T cell epitope prediction and epitope content score calculation 116 5.3.3 Human epitope similarity and human similarity score calculation 117 5.3.4 Pre-existing immunity evaluation of the designed proteins 117 5.3.5 Foldability assessment of the designed proteins 118 5.3.6 Molecular dynamics (MD) simulation 120 5.4 Results 121 5.5 Discussion 141 5.6 Acknowledgement 143 Chapter 6 Ontology-based Vaccine Data Integration and Analysis 145 6.1 Abstract 145 6.2 Introduction 146 6.3 Methods 151 6.3.1 OHPI ontology development 151 6.3.2 VIO ontology development 153 iv 6.4 Results 155 6.4.1 Modeling Host-Pathogen Interactions with OHPI 155 6.4.2 Modeling Vaccine Investigation Data with VIO 161 6.5 Discussion 167 6.6 Acknowledgement 169 Chapter 7 Summary and Discussion 170 7.1 Summary 170 7.2 Future Direction of the Vaxign framework 174 7.3 The Promise of Precision Vaccinology 174 Bibliography 180 v List of Tables Table 2-1 A list of Gram-positive and Gram-negative bacteria used to analyze significant features associated with protective antigens. ................................................................................ 27 Table 2-2 Frequent conserved domains among reported protective antigens. .............................. 36 Table 3-1 Nested five-fold cross-validation evaluation metrics of five machine learning algorithms trained using four data resampling methods. .............................................................. 61 Table 3-2 Leave-one-pathogen-out evaluation metrics of five machine learning algorithms trained using four data re-sampling methods. ............................................................................... 65 Table 3-3 Benchmarking of Vaxign-ML compared to publicly available programs and epitope- based method. ............................................................................................................................... 68 Table 3-4
Details
-
File Typepdf
-
Upload Time-
-
Content LanguagesEnglish
-
Upload UserAnonymous/Not logged-in
-
File Pages225 Page
-
File Size-