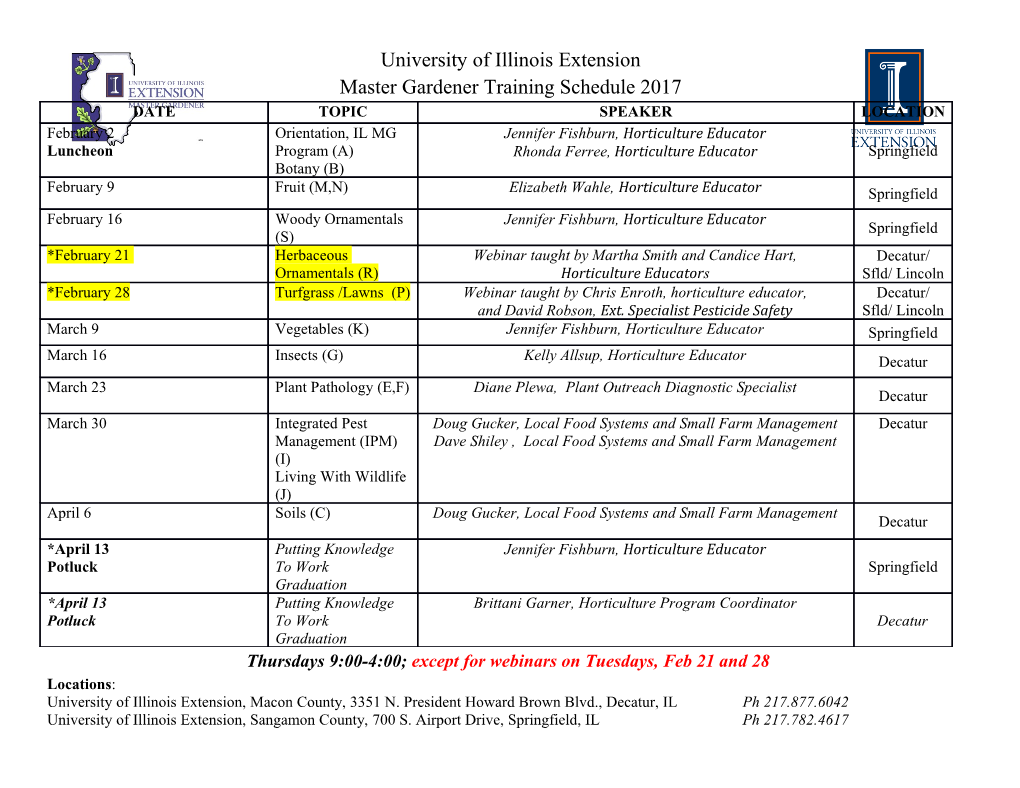
FUZZ-IEEE 2016 Tutorial: FUZZ-IEEE-03 Type-2 Fuzzy Ontology and Fuzzy Markup Language for Real-World Applications Organized by Chang-Shing Lee, National University of Tainan, Taiwan Giovanni Acampora, Nottingham Trent University, UK Yuandong Tian, Facebook AI Research, USA 24 July, 2016 0 / 71 FUZZ-IEEE 2016 Tutorial: FUZZ-IEEE-03 Part 1: Type-2 Fuzzy Ontology and Applications Chang-Shing Lee, NUTN, Taiwan Part 2: Fuzzy Markup Language Giovanni Acampora, NTU, UK Part 3: Real-World Application on Game of Go Yuandong Tian, Facebook AI Research, USA 1 / 71 FUZZ-IEEE 2016 Tutorial: FUZZ-IEEE-03 Part 1 Type-2 Fuzzy Ontology and Applications Chang-Shing Lee National University of Tainan, Taiwan 2 / 71 Research Team 3 / 71 Co-Sponsors 4 / 71 Type-2 Fuzzy Ontology Applications • FML IEEE 1855-2016 Standard • Type-2 Fuzzy Set • Fuzzy Ontology • Game of Go Application • Personalized Diet Recommendation • Adaptive Learning Application 5 / 71 FML IEEE 1855-2016 6 / 71 Introduction to T2FS (1/5) 7 / 71 Introduction to T2FS (2/5) i m ~ (x ) Vertical Slice u A 2 W 1 x'1 ~ Wx'N UMF(A) ~ UMF(A) u i i MF1(x ) MFN (x ) i u u MFN (x ) 1 n ~ m A (x, u) Embedded T2 FS i x MF1(x ) u ~ Embedded LMF(A) x T1 FS 0 i ~ l x r Some eye Contact (A) Uncertainty About Uncertainty About Left End-Point Right End-Point 8 / 71 Introduction to T2FS (3/5) u 1 ~ ~ UMF (A) UMF (A) Embedded FS ~ LMF (A) ~ ~ FOU (A) FOU (A) X 9 / 71 Introduction to T2FS (4/5) Type-2 FLS Output Processing Crisp Rules Defuzzifier outputs y ≠Y Crisp Type-reducer inputs Fuzzifier Type-reduced x ≠X Set(Type-1) Fuzzy Fuzzy input sets Inference output sets ~ ~ F Ax (or Ax ) x 第二型模糊邏輯系統 10 / 71 Introduction to T2FS (5/5) u u ~ Low Low 1.0 1.0 UMF 0.8 0.8 0.6 0.6 0.4 0.4 LMF 0.2 0.2 0 0 0 ( ) 5 10 15 20 25 30 40 x C 0 5 10 15 20 25 30 40 x( C) mA (xÅ,u) 1.0 ~ Å 0.8 uA (x ,u) 0.6 1.0 0.4 0.8 0.2 5 10 15 20 25 30 40 0 0.6 0.2 x 0.4 0.4 0.6 0.2 0.8 1.0 0 0.2 0.4 0.6 0.8 1.0 u u 11 / 71 12 / 71 13 / 71 14 / 71 15 / 71 16 / 71 Dynamic Assessment and IRT-based Learning Application 17 / 71 18 / 71 19 / 71 20 / 71 21 / 71 Video Demonstration • Taiwan Open 2009 • Human vs. Computer Go @ IEEE WCCI 2012 • Human vs. Computer Go @ FUZZ-IEEE 2011 • Human vs. Computer Go in Taiwan in 2011 22 / 71 Adaptive linguistic assessment Game Results Repository Domain Expert Domain Expert Game Results T2FS Construction Repository Mechanism KB/RB Adaptive Go-Ranking Repository Assessment Ontology PSO Model Human vs. MoGoTW Estimation Mechanism T2FS-based Genetic Learning Adaptive UCT-based Mechanism Go-Ranking Mechanism Bradley-Terry Model Estimation Mechanism T2FS-based Fuzzy Inference Mechanism MoGoTW Players Human-Performance Mapping Mechanism Semantic Analysis Mechanism Personal Profile Repository Players Rank Repository 23 / 71 Go-ranking assessment ontology Adaptive Go-Ranking Assessment Ontology Domain Layer 7x7 13x13 9x9 . 19x19 Category Layer God . NCKU Class Layer IEEE WCCI Temple FUZZ-IEEE . NUTN 2012 Where 2013 . Amateur Professional Who Certificated Rank Player Player 6D . Gender Age MoGoTW Male 45 2013/7/9 . 2013/7/8 . When . How White Time Setting Machine Spec 45mins/Side Rule HP ProLiant DL785 Komi Black Round 7.5 Chinese 2 Round1 Game 13 Game14 . SN ,GR . Game11 13 13 SN14,GR14 . Game1K-1 SN11,GR11 Game12 SN1K-1,GR1K-1 Game1K SN12,GR12 RoundN SN1K,GR1K ~ ~ ~ . SN WinningRate ~ Komi 121145 60 . GameWeight 7.5 19 . RankMethod RankActual 6.38D 6D What SN: Simulation Number ~ ~ 24 / 71 GR: Game Result Low Medium H~igh Fuzzy inference structure Note: (1) M denotes number of fired rules Output Lay e r (2) xÅ = {GW Å,WRÅ, SN Å, KomiÅ} Rank (xÅ) (3) GW is GameWeight AVG (4) WR is WinningRate Type-Reduction Layer Rankl (xÅ) Rankr (xÅ) KM KM Consequent Layer 1 1 M M [Rank , Rank ] [Rank l , Rank r ] . l r . KM KM Rule Layer 1 1 M M . [ f (xÅ), f (xÅ)] . [ f (xÅ), f (xÅ)] MIN MIN Antecedent Layer ~ [m ~ (Kom iÅ), m Komi (Kom iÅ)] [m ~ ( Å), m ~ ( Å)] Komi Medium Medium . GW GW Å ~ Å GWLow [m ~ (Komi ), m Komi (Komi )] GWLow KomiLow Low . ~ ~ [m (GW Å),m GW (GW Å)] GWMedium Medium ~ [m ~ (GW Å), m GW (GW Å)] GWHigh High ~ [m ~ (KomiÅ),m Komi (KomiÅ)] KomiHigh High Input Layer S N Å GW Å WRÅ KomiÅ 25 / 71 Personalized Diet Recommendation 26 / 71 Diet assessment / recommendation ontology Adaptive Diet Assessment Ontology Domain Layer Japan UK Taiwan . USA Category Layer NUTN Class Layer FuCheng . ChiKu Campus RongYu Campus Campus . OASE CASDL VCI Lab. Where Lab. Lab. Under- Assistant Graduate Advisor Who Graduate . 11/1/2009 . 11/14/2009 . 11/30/2009 When ~Diet Goal How 2000(2000)kcal ~Fats & Nuts Vegetables Whole Grains & 6(6) servings ~ Starches Meats & 3.5(3.5)servings ~Low-Fat Milk ~ 1.5(1.5) servings 12(12) servings ~ Fruits ~ Proteins 3.5(3.5) servings 6(6)servings Actual Caloric Intake 2500~ (2500)kcal What Breakfast Dinner Lunch ~Corn Soup . 1(1)portion ~ Dumpling ~Corn Soup Caramel 1.5(1.5) portions 1(1)portion Seafood Spaghetti Pudding with Tomato Sauce ~ Black Tea ~ 1(1) portion Pork Bun Soy Milk ~ 1(1) portion ~ ~ 1(1) portion . 1(1) portion 1(1) portion Whole Grains Fats & Meats & Proteins Sugar Vegetables & Starches Nuts ~ ~ ~ Fruits Low-Fat Milk ~ ~ 10(9.65) 72(72)g 0.5(0.5) serving ~ ~ 14.5(14.5) 9(9.3) servings 0(0) serving 1(0.6) serving servings servings ~Carbohydrate ~ Fat 1197(1196.8)kcal ~ Protein 874(874.35)kcal FGB 407(407.4)kcal ~ PCF 1(0.66) PCC ~ PCR ~ PCP 35(35.28)% ~ 48(48.29)% ~ 124(123.93)% 16(16.44)% PCR: Percentage of Caloric Ratio FGB: Food Group Balance . DHL DHL: Dietary Healthy Level DHL~ Method ~ DO DO: Desired Output 3.4(3.42) 4(4) PCC: Percentage of Calories from Carbohydrate PCP: Percentage of Calories from Protein Rclass_Semantic PCF: Percentage of Calories from Fat ~ ~ ~ Recommended 27 / 71 VeryLow Low Medium High~ VeryHigh~ . Semantics Layer Personalized diet recommendation Step 6.2 Taiwan T2 FS-based Learning Mechanism Step 5 Step 6 … Step 6.1 … Training Data T2 FS-based Genetic Learning Mechanism Personalized KB Step 1 … Step 4 Step 4.1 Domain Experts Nutritional Balanced Step 6.3 Mechanism T2 FS-based Fuzzy Inference Food Item Database Mechanism Step 2 Step 4.2 Ontology Experts … Caloric Balanced Step 6.4 Mechanism Linguistic Knowledge Meal Record Database Discovery Mechanism Step 3 Adaptive Personal … Diet Assessment and Step 7.1 Step 8 Step 4.3 Recommendation Ontology Type-2 Six-Food-Group Subjects Balanced Mechanism … Dietary Health Balanced Computation Mechanism Level Repository T2GFML Repository Step 7.2 28 / 71 T2FS fuzzy variables u u 1 1 PCC (%) PCP (%) 0 20 40 60 80 100 0 20 40 60 80 100 (a) (b) u u 1 1 PCF (%) FGB -1 0 1 2 3 4 5 6 0 20 40 60 80 100 (c) (d) u u 1 1 PCR (%) DHL 0 40 80 120 160 200 0 2 4 6 8 10 12 (e) (f) 29 / 71 ~ ~ ~ ~ ~ ~ ~ ~ ~~ ~ ~ Low~ Low ~ Medium~ ~ ~ ~ uu Low VeryLow~ LowMediumMedium MediumMediumMedium HighHigh HighHighVeryHighHigh 1 Low High 11 PCC(%) PCRPCP(%) FGB PCF(%) DHL 10102010 20204020 3030 6030 4040 8040 5050 100506060 120607070 140708080 160908090 10010018090 100200 -1 10 2 1 3 2 4 3 5 4 6 5 7 6 8 9 10 11 12 u uu LowLow VeryLowMediumMediumLow Low MediumMedium HighHigh HighHighVeryHigh 1 Medium High u11 Low Low Medium High 1 FGB PCFPCC(%)(%) PCPDHL(%) 10 20 30 40 50 60 70 80 90 100 -1 101010 202 1 303 2 404 3 505 4 606 5 707 6 80808 90990 10010100 11 PCR12(%) 20 40 60 80 100 120 140 160 180 200 T2FS fuzzy variables ~ ~ ~ Low Medium ~ ~ ~ u High u Low Medium High 1 1 PCC(%) PCP(%) 10 20 30 40 50 60 70 80 90 100 10 20 30 40 50 60 70 80 90 100 (a) (b) u Low~ Medium High u Low Medium High ~ ~ ~ ~ ~ u1 Low Medium High 1 u Low Medium High 1 1 PCC(%) PCP(%) 10 20 30 40 50 60 70 80 90 100PCF(%) 10 20 30 40 50 60 70 80 FGB90 100 10 20 30 40 50 60 70 80 90 100 -1 0 1 2 3 4 5 6 u Low Medium (c) High u (d) 1 ~ ~ ~ ~ Low~ ~ Medium ~ High~ u Low Medium High u VeryLow Low Medium High VeryHigh 1 1 PCF(%) FGB 10 20 30 40 50 60 70 80 90 100 ( ) -1 0 1 2 3 4 5 6 PCR % DHL 20 40 60 80 100 120 140 160 180 200 1 2 3 4 5 6 7 8 9 10 11 12 (e) u VeryLow Low Medium (f) High VeryHigh u 1 Low Medium High 1 DHL PCR(%) 1 2 3 4 5 6 7 8 9 10 11 12 20 40 60 80 100 120 140 160 180 200 30 / 71 Adaptive Learning Application 31 / 71 Video Demonstration • Knowledge Web for World-Wide Students Learning (KWSLearn) – Website: https://sites.google.com/site/kwslearn/ – Cooperated Organization: • Boyo Social Welfare Foundation, Taiwan • Tainan City Government, Taiwan • National University of Tainan (NUTN), Taiwan • Center for Research of Knowledge Application & Web Service (KWS), NUTN, Taiwan • Ontology Application & Software Engineering (OASE) Lab.
Details
-
File Typepdf
-
Upload Time-
-
Content LanguagesEnglish
-
Upload UserAnonymous/Not logged-in
-
File Pages120 Page
-
File Size-