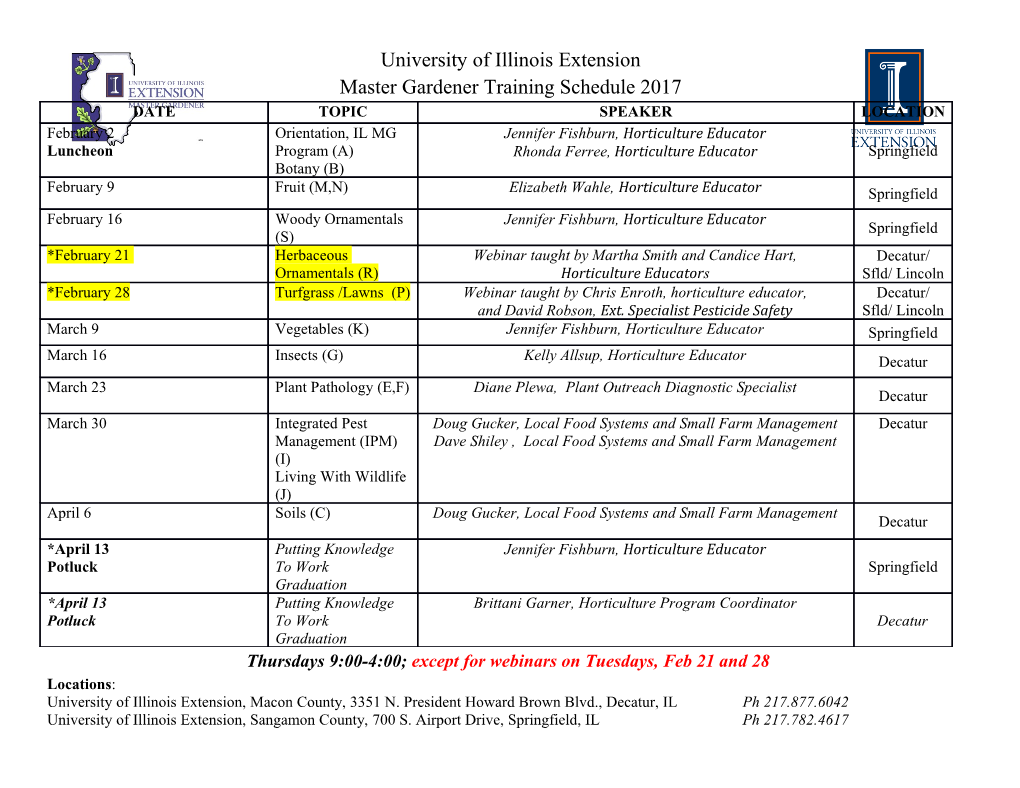
Quantitative Analysis READING 12 FUNDAMENTALS OF PROBABILITY ❑ BASICS OF PROBABILITY KEY POINTS 1.)0<P<1 2.)A Random Variable is a variable whose value is unknown or a function that assigns values to each of an experiment's outcomes. 3.) An Unconditional Probability is the chance that a single outcome results among several possible outcomes. The term refers to the likelihood that an event will take place irrespective of whether any other events have taken place or any other conditions are present. 4.)The probability that a random variable will have a specific outcome given that some other outcome has occurred, is referred to as Conditional Probability. P(A/B)=P(A∩ 퐵) /푃(퐵) 5.) The probability that both A and B will occur is written P(AB) and referred to as the Joint Probability. P(AB) =P(A/B) x P(B) ❑ EVENT AND EVENT SPACES EVENT Event is a single outcome or a combination of outcomes for a random variable. EVENT SPACE The event space E is the set of the possible distinct outcomes of the random process. The event space is sometimes called the sample space. When rolling a normal six-sided die and recording the uppermost face, the event space is E={1,2,3,4,5,6}. ❑INDEPENDENT EVENTS Two events are independent events if knowing the outcome of one does not affect the probability of the other. When two events are independent following must hold: 1.) P(A) X P(B) = P(AB) 2.) P(A/B) = P(A) 3.) If A1 , A2 ,……..AN are independent events , their joint probability P( A1 and A2……..and An)is equal to P(A1) x P(A2) x………..x P(An) ❑ MUTUALLY EXCLUSIVE EVENTS Two events are mutually exclusive events if they cannot both happen. ❑CONDITIONALY INDEPENDENT EVENTS Two conditional probabilities , P(A/C) and P(B/C) , may be independent or dependent regardless of weather the unconditional the unconditional probabilities ,P(A) and P(B) , are independent or not. When two events are conditionally independent events , P(A/C) x P(B/C) = P(AB/C) ❑TOTAL PROBABILITY RULE . The Total Probability Rule states that if the conditional events Bi are mutually exclusive and exhaustive then: P(A)=P(A/B1)P(B1) + P(A/B2)P(B2) +…..+P(A/Bn)P(Bn) ❑JOINT PROBABILITY Given a conditional probability and the unconditional probability of the conditional event, we can calculate the Joint Probability of both events using P(AB) =P(A/B) x P(B) , .❑BAYES RULE Bayes, Rule allows us to use information about the outcome of one event to improve our estimates of the unconditional probabilities of another event. P(A/B) = P(B/A) x P(A) / P(B) . Questions 1.) A dealer in a casino has rolled a five on a single die three times in a row. What is the probability of her rolling another five on the next roll, assuming it is a fair die? A. 0.200. B. 0.001. C. 0.167. D. 0.500. Explanation (0.167) The probability of a value being rolled is 1/6 regardless of the previous value rolled. 2.)If X and Y are independent events, which of the following is most accurate? A. P(X or Y) = P(X) + P(Y). B. P(X | Y) = P(X). C. P(X or Y) = (P(X)) × (P(Y)). D. X and Y cannot occur together. Explanation (B) Note that events being independent means that they have no influence on each other. It does not necessarily mean that they are mutually exclusive. Accordingly, P(X or Y) = P(X) + P(Y) − P(X and Y). By the definition of independent events, P(X|Y) = P(X). READING 13 RANDOM VARIABLES .❑RANDOM VARIABLES DISCRETE RANDOM VARIABLES A Discrete Random Variable is one that can take on only a countable number of possible outcomes. If it can take on only two possible values, zero and one , it is referred to as Bernoulli Random Variable. CONTINUOUS RANDOM VARIABLES A Continues Random Variable has an uncountable number of possible outcomes. Because there are an infinite number of possible outcomes, the probability of any single value is zero. For Continues Random Variables, we measure probability over some positive interval. ❑ PROBABILITY FUCTION . PROBABILITY MASS FUNCTION A Probability Mass Function (PMF), f(x)=P(X=x), gives us the probability that the outcome of a discrete random variable, X, will be equal to a given number, x. For all of these PMFs , the sum of the probabilities of all possible outcome is 100% , a requirement for a PMF . For a Bernoulli Random Variable for which the P(x=1)=p , the PMF is f(x)=px (1-p)1-x . This yields P(x=1)=p and P(x=0)=1-p CUMULATIVE DISTRIBUTION FUNCTION A Cumulative Distribution function (CDF) gives us the probability that a random variable will take on value less than or equal to x [ i.e. ,F(x) =P(X≤ 풙) ]. For Bernoulli Random Variable, the CDF is: 0 x<0 F(x)=1-p 0≤ 풙 < ퟏ 1 x≥ ퟏ ❑EXPECTATION The Expected Value is the weighted average of the possible outcomes of a random variable , where the weights are the probabilities that the outcome will occur. E(X)= ∑푷 풙풊 풙풊 = 푷 풙ퟏ 풙ퟏ + 푷 풙ퟐ 풙ퟐ + … … 푷 풙풏 풙풏 Statistically speaking , our best guess of the outcome of a random variable is the Expected Value. PROPERTIES OF EXPECTED VALUE: 1. If c is any constant , then: E(cx) = cE(X) 2. If X and Y are any random variables , then: E(X+Y) =E(X) +E(Y) SKEWNESS, a measure of a distributions symmetry, is the standardized Third Moment. We standardize it by dividing it by the standard deviation cubed. = 퐸[ 푋 − 휇 3 ]/ 휎3 ❑ FOUR COMMON POPULATION MOMENTS The first moment, the MEAN of a random variable is its expected value E(X).which is represented by the Greek letter 휇 푚푢 . The second central movement of a random variable is its VARAIANCE, 휎2 . VARIANCE gives us information about how widely dispersed the values of random variable are around the mean. KURTOSIS, is the standard Forth Moment. It is a measure of the shape of the distribution , in particular the total probability in the tails of the distribution relative to the probability in the rest of the distribution. The higher the KURTOSIS, the greater the probability in the tails of the distribution. = 퐸[ 푋 − 휇 4 ]/ 휎4 Questions 1.) There is a 30% chance that the economy will be good and a 70% chance that it will be bad. If the economy is good, your returns will be 20% and if the economy is bad, your returns will be 10%. What is your expected return? A. 13%. B. 15%. C. 17%. D. 18%. Explanation Expected value is the probability weighted average of the possible outcomes of the random variable. The expected return is: ((0.3) × (0.2)) + ((0.7) × (0.1)) = (0.06) + (0.07) = 0.13 2.)An analyst is currently considering a portfolio consisting of two stocks. The first stock, Remba Co., has an expected return of 12% and a standard deviation of 16%. The second stock, Labs, Inc., has an expected return of 18% and a standard deviation of 25%. The correlation of returns between the two securities is 0.25.If the analyst forms a portfolio with 30% in Remba and 70% in Labs, what is the portfolio's expected return? A. 15.0%. B. 17.3%. C. 21.5%. D. 16.2%. Explanation ER = Σ(ER )(W ) ER = w ER + w ER , where ER = Expected Return and w = % invested in each stock. ER = (0.3 × 12) + (0.70 × 18) = 3.6 + 12.6 = 16.2% READING 14 COMMON UNIVARIATE RANDOM VARIABLES ❑ THE UNIFORM DISTRIBUTION The Continuous Uniform Distribution is defined over a range that spans between some lower limit, a, and some upper limit , b, which serve as the parameters of the distribution. Outcomes can only occur between a and b and because we are dealing with continuous distribution, even if a<x<b , P(X=x)= 0. PDF : f(x) = 1/b-a for a≤ 풙 ≤ 풃 , 풆풍풔풆 풇 풙 = ퟎ E(x) = a+ b/2 Var(x) = (b-a)2 /12 ❑ BERNOULLI DISTRIBUTION A Bernoulli Random Variable only has two possible outcomes, success or failure. Probability of success = p , 1 Probability of failure =(1-p) , 0 MEAN: p VAR(x): p(1-p) PMF: f(x)=px (1-p)1-x CDF: 0 x<0 F(x)=1-p 0≤ 풙 < ퟏ 1 x≥ ퟏ ❑ BINOMIAL DISTRIBUTION The Binomial Random Variable may be defined as the number of successes in a given number of Bernoulli trails, whereby the outcome of each INDEPENDENT TRIAL can be either success or failure. 푛! ( − ) P(x) = P(X=x) = 푝푥 1 − 푝 푛 푥 푛−푥 !푥! Expected Value of X = E(X) =np Variance of X = np(1-p) = npq Where: n= number of trails p = probability of success in each independent trial q = probability of failure in each independent trail = (1-p) The binomial distribution model allows us to compute the probability of observing a specified number of "successes" when the process is repeated a specific number of times and the outcome for a given trail is either a success or a failure ❑ POISSION DISTRIBUTION Poisson Random Variable X refers to the NUMBER OF SUCCESS PER UNIT, The parameter (흀) refers to average or EXPECTED NUMBER OF SUCCESS PER UNIT. Probability of obtaining X successes given that 휆 successes are expected is given by: 휆푥푒−휆 p(X=x) = 푥! MEAN :휆 VARIANCE :휆 POISSION DISTRIBUTION can be used to calculate number of defects per batch in production or number of call per minute to a toll free number.
Details
-
File Typepdf
-
Upload Time-
-
Content LanguagesEnglish
-
Upload UserAnonymous/Not logged-in
-
File Pages181 Page
-
File Size-