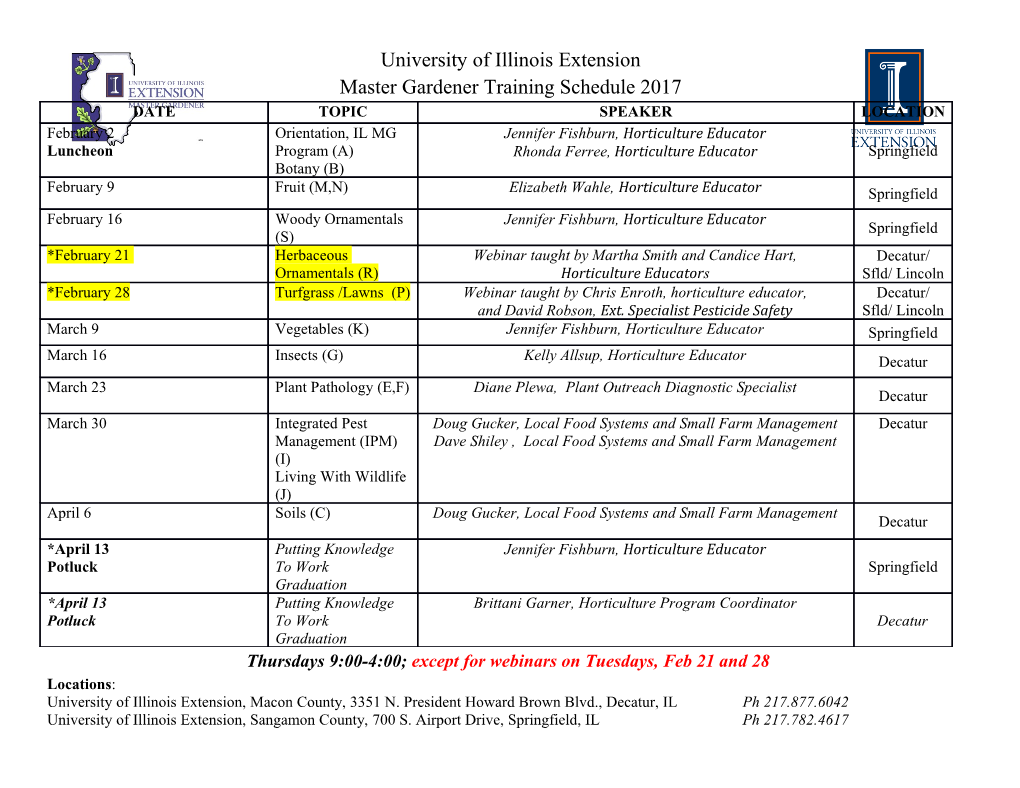
Fisher Information April 6, 2016 Debdeep Pati 1 Fisher Information Assume X ∼ f(x j θ) (pdf or pmf) with θ 2 Θ ⊂ R. Define @ 2 I (θ) = E log f(X j θ) X θ @θ @ where @θ log f(X j θ) is the derivative of the log-likelihood function evaluated at the true value θ. Fisher information is meaningful for families of distribution which are regular: 1. Fixed support: fx : f(x j θ) > 0g is the same for all θ. @ 2. @θ log f(x j θ) must exist and be finite for all x and θ. 3. If EθjW (X)j < 1 for all θ, then @ k @ k Z Z @ k E W (X) = W (x)f(x j θ)dx = W (x) f(x j θ)dx @θ θ @θ @θ 1.1 Regular families One parameter exponential families: Cauchy location or scale family: 1 f(x j θ) = π(1 + (x − θ)2) 1 f(x j θ) = πθ(1 + (x/θ)2) and lots more. (Most families of distributions used in applications are regular). 1.2 Non-regular families Uniform(0; θ) Uniform(θ; θ + 1): 1 1.3 Facts about Fisher Information Assume a regular family. 1. @ E log f(X j θ) = 0: θ @θ @ Here @θ log f(X j θ) is called the \score" function S(θ). Proof. @ Z @ E log f(X j θ) = log f(x j θ) f(x j θ)dx θ @θ @θ Z @ f(x j θ) = @θ f(x j θ)dx f(x j θ) Z @ = f(x j θ)dx @θ @ Z = f(x j θ)dx = 0 @θ since R f(x j θ)dx = 1 for all θ. @ 2. IX (θ) = Varθ @θ log f(X j θ) . @ Proof. Since Eθ @θ log f(X j θ) = 0 @ @ 2 Var log f(X j θ) = E log f(X j θ) = I (θ): θ @θ θ @θ X 3. If X = (X1;X2;:::;Xn) and X1;X2;:::;Xn are independent random variables, then IX (θ) = IX1 (θ) + IX2 (θ) + ··· IXn (θ). Proof. Note that n Y f(x j θ) = fi(xi j θ) i=1 2 where fi(· j θ) is the pdf (pmf) of Xi. Observe that n @ X @ log f(X j θ) = log f (X j θ) @θ @θ i i i=1 and the random variables in the sum are independent. This n @ X @ Var log f(X j θ) = Var log f (X j θ) @θ @θ i i i=1 Pn so that IX (θ) = i=1 IXi (θ) by 2. 4. If X1;X2;:::;Xn are i.i.d and X = (X1;X2;:::;Xn), then IXi (θ) = IX1 (θ) for all i so that IX (θ) = nIX1 (θ). 5. An alternate formula for Fisher information is @2 I (θ) = E − log f(X j θ) X θ @θ2 R R R @ Proof. Abbreviate f(x j θ)dx as f, etc. Since 1 = f, applying @θ to both sides, @ Z Z @f Z @ 0 = f = = @θ · f @θ @θ f Z @ = log f f: @θ @ Applying @θ again, @ Z @ 0 = log f f @θ @θ Z @ @ = log f f @θ @θ Z @2 Z @ @f = log f · f + log f @θ2 @θ @θ Noting that @f @f = @θ · f; @θ f @ = log f f; @θ 3 this becomes Z @2 Z @ 2 0 = log f · f + log f · f @θ2 @θ or @2 0 = E log f(X j θ) + I (θ): @θ2 X Example: Fisher Information for a Poisson sample. Observe X = (X1;:::;Xn) iid ~ Poisson(λ). Find IX(λ). We know IX(λ) = nIX1 (λ). We shall calculate IX1 (λ) in three ~ ~ ways. Let X = X1. Preliminaries: λxe−λ f(x j λ) = x! log f(x j λ) = x log λ − λ − log x! @ x log f(x j λ) = − 1 @λ λ @2 x − log f(x j λ) = @λ2 λ2 Method #1: Observe that @ 2 X 2 I (λ) = E log f(X j λ) = E − 1 X λ @λ λ λ X X EX = Var (sinceE = = 1) λ λ λ λ Var(X) λ λ 1 = = = = λ2 λ2 λ2 λ Method #2: Observe that @ X I (λ) = Var log f(X j λ) = Var − 1 X λ @λ λ X 1 = Var = (as in Method#1): λ λ Method #3: Observe that @2 X λ 1 I (λ) = E − log f(X j λ) = E = = : X λ @λ2 λ λ2 λ2 λ 4 n Thus IX(λ) = nIX1 (λ) = λ . ~ Example: Fisher information for Cauchy location family. Suppose X1;X2;:::;Xn iid with pdf 1 f(x j θ) = : π(1 + (x − θ)2) Let X = (X1;:::;Xn);X ∼ f(x j θ). Find IX(θ). ~ ~ Note that IX(θ) = nIX1 (θ) = nIX (θ). Now ~ @ @f log f(x j θ) = @θ @θ f −1 π(1+(x−θ)2)2 · 2(x − θ)(−1) = 1 π(1+(x−θ)2) 2(x − θ) = (1 + (x − θ)2) Now @ 2 I (θ) = E log f(X j θ) X @θ 2(X − θ) 2 = E 1 + (X − θ)2 Z 1 2(x − θ) 2 1 = 2 2 dx −∞ 1 + (x − θ) π(1 + (x − θ) ) 4 Z 1 (x − θ)2 = 2 3 dx: π −∞ (1 + (x − θ) ) Letting u = x − θ; du = dx, 4 Z 1 u2 IX (θ) = 2 3 du π −∞ (1 + u ) 8 Z 1 u2 = 2 3 du: π 0 (1 + u ) 5 Substituting x = 1=(1 + u2), u = (1=x − 1)1=2; du = 0:5(1=x − 1)−1=2(−1=x2)dx, 8 Z 1 u2 IX (θ) = 2 3 du π 0 (1 + u ) 8 Z 1 u2 1 2 = 2 2 du π 0 (1 + u ) 1 + u 8 Z 1 = (1 − x)x2 · (1=2)(1=x − 1)−1=2(1=x2)dx π 0 4 Z 1 = x1=2(1 − x)1=2dx π 0 4 Z 1 = x3=2−1(1 − x)3=2−1dx (Beta integral) π 0 p 4 Γ(3=2)Γ(3=2) 4 (0:5 π)2 = = π Γ(3=2 + 3=2) π 2! 1 = : 2 Hence IX(θ) = n=2. ~ 2 Uses of Fisher Information • Asymptotic distribution of MLE's • Cram´er-RaoInequality (Information inequality) 2.1 Asymptotic distribution of MLE's • i.i.d case: If f(x j θ) is a regular one-parameter family of pdf's (or pmf's) and θ^n = θ^n(Xn) is the MLE based on Xn = (X1;:::;Xn) where n is large and X1;:::;Xn are iid from f(x j θ), then approximately, 1 θ^ ∼ N θ; n nI(θ) where I(θ) ≡ IX1 (θ) and θ is the true value. Note that nI(θ) = IXn (θ). More formally, ^ θn − θ p d = nI(θ)(θ^n − θ) ! N(0; 1) q 1 nI(θ) 6 as n ! 1. • More general case: (Assuming various regularity conditions) If f(x j θ) is a one- ~ parameter family of joint pdf's (or joint pmf's) for data Xn = (X1;:::;Xn) where n is large (think of a large dataset arising from regression or time series model) and θ^n = θ^n(Xn) is the MLE, then 1 θ^n ∼ N θ; IXn (θ) where θ is the true value. 2.2 Estimation of the Fisher Information If θ is unknown, then so is IX(θ). Two estimates I^ of the Fisher information IX(θ) are @2 I^ = I (θ^); I^ = − log f(X j θ)j 1 X 2 @θ2 θ=θ^ where θ^ is the MLE of θ based on the data X. I^1 is the obvious plug-in estimator. It can be difficult to compute IX (θ) does not have a known closed form. The estimator I^2 is suggested by the formula @2 I (θ) = E − log f(X j θ) X @θ2 It is often easy to compute, and is required in many Newton- Raphson style algorithms for finding the MLE (so that it is already available without extra computation). The two estimates I^1 and I^2 are often referred to as the \expected" and \observed" Fisher information, respectively. As n ! 1, both estimators are consistent (after normalization) for IXn (θ) under various regularity conditions. ^ ^ For example: in the iid case: I1=n; I2=n, and IXn (θ)=n all converge to I(θ) ≡ IX1 (θ). 2.3 Approximate Confidence Intervals for θ Choose 0 < α < 1 (say, α = 0:05). Let z∗ be such that P (−z∗ < Z < z∗) = 1 − α where Z ∼ N(0; 1). When n is large, we have approximately p ^ IX(θ)(θ − θ) ∼ N(0; 1) 7 so that ∗ p ^ ∗ P − z < IX(θ)(θ − θ) < z ≈ 1 − α or equivalently, s s 1 1 P θ^ − z∗ < θ < θ^ + z∗ ≈ 1 − α: IX(θ) IX(θ) This approximation continues to hold when IX(θ) is replaced by an estimate I^ (either I^1 or I^2): r1 r1 P θ^ − z∗ < θ < θ^ + z∗ ≈ 1 − α: I^ I^ Thus r1 r1 θ^ − z∗ ; θ^ + z∗ I^ I^ is an approximate 1 − α confidence interval for θ. (Here θ^ is the MLE and I^ is an estimate of the Fisher information.) 3 Cramer-Rao Inequality Let X ∼ Pθ; θ 2 Θ ⊂ R. ~ Theorem 1. If f(x j θ) is a regular one-parameter family, EθW (X) = τ(θ) for all θ, and ~ ~ τ(θ) is differentiable, then fτ 0(θ)g2 Varθ(W (X)) ≥ : ~ IX(θ) ~ Proof.
Details
-
File Typepdf
-
Upload Time-
-
Content LanguagesEnglish
-
Upload UserAnonymous/Not logged-in
-
File Pages18 Page
-
File Size-