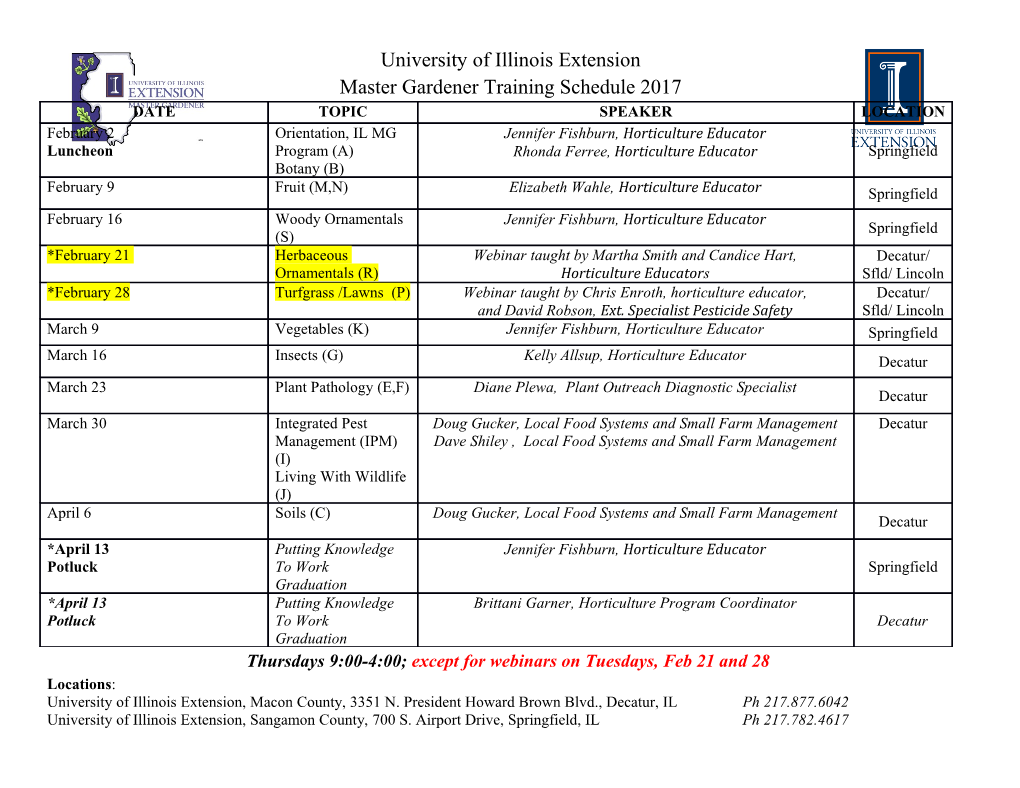
Proceedings of the Thirtieth AAAI Conference on Artificial Intelligence (AAAI-16) Infinite Plaid Models for Infinite Bi-Clustering Katsuhiko Ishiguro, Issei Sato,* Masahiro Nakano, Akisato Kimura, Naonori Ueda NTT Communication Science Laboratories, Kyoto, Japan *The University of Tokyo, Tokyo, Japan Abstract (such as k-means) for matrices: the entire matrix is parti- tioned into many rectangle blocks, and all matrix entries are We propose a probabilistic model for non-exhaustive and assigned to one (or more) of these blocks (Fig. 1). However, overlapping (NEO) bi-clustering. Our goal is to extract a few for knowledge discovery from the matrix, we are only inter- sub-matrices from the given data matrix, where entries of a ested in few sub-matrices that are essential or interpretable. sub-matrix are characterized by a specific distribution or pa- rameters. Existing NEO bi-clustering methods typically re- For that purpose exhaustive bi-clustering techniques require quire the number of sub-matrices to be extracted, which is post-processing in which all sub-matrices are examined to essentially difficult to fix a priori. In this paper, we extend identify “informative” ones, often by painful manual effort. the plaid model, known as one of the best NEO bi-clustering On the contrary, the goal of non-exhaustive bi-clustering algorithms, to allow infinite bi-clustering; NEO bi-clustering methods is to extract the most informative or significant without specifying the number of sub-matrices. Our model sub-matrices, not partitioning all matrix entries. Thus the can represent infinite sub-matrices formally. We develop a non-exhaustive bi-clustering is more similar to clique detec- MCMC inference without the finite truncation, which poten- tion problems in network analysis: not all nodes in a net- tially addresses all possible numbers of sub-matrices. Exper- iments quantitatively and qualitatively verify the usefulness work are extracted as clique members. Non-exhaustive bi- of the proposed model. The results reveal that our model can clustering would greatly reduce the man-hour costs of man- offer more precise and in-depth analysis of sub-matrices. ual inspections and knowledge discovery, because it ignores non-informative matrix entries (Fig. 1). Hereafter let us use “bi-clustering” to mean “NEO bi- Introduction clustering”. There has been a plenty of bi-clustering re- searches over years, e.g. (Cheng and Church 2000; Lazze- In this paper, we are interested in bi-clustering for matrix roni and Owen 2002; Caldas and Kaski 2008; Shabalin et data analysis. Given the data, the goal of bi-clustering is to al. 2009; Fu and Banerjee 2009). However, there is one fa- extract a few (possibly overlapping) sub-matrices1 from the tal problem that has not been solved yet: determining the matrix where we can characterize entries of a sub-matrix by number of sub-matrices to be extracted. Most existing bi- a specific distribution or parameters. Bi-clustering is poten- clustering methods assume that the number of sub-matrices tially applicable for many domains. Assume an mRNA ex- is fixed a priori. This brings two essential difficulties. First, pression data matrix, which consists of rows of experimen- finding the appropriate number of sub-matrices is very diffi- tal conditions and columns of genes. Given such data, biolo- cult. Recall that determining “k” for classical k-means clus- gists are interested in detecting pairs of specific conditions × tering is not trivial in general. And the same holds, per- gene subsets that have different expression levels compared haps more difficult (Gu and Liu 2008), for bi-clustering. to other expression entries. From a user × product purchase Second, sub-optimal choices of the sub-matrix number in- record, we can extract sub-matrices of selected users × par- evitably degrade bi-clustering performances. For example, ticular products that sell very good. Successful extraction of assume there are K = 3 sub-matrices incorporated in the such sub-matrices is the basis for efficient ad-targeting. given data matrix. Solving the bi-clustering by assuming More specifically we study the non-exhaustive and over- K = 2(< 3) never recover the true sub-matrices. If we solve lapping (NEO) bi-clustering. Here we distinguish between with K = 5(> 3), then the model seeks for two extra sub- the exhaustive and non-exhaustive bi-clustering. matrices to fulfill the assumed K; e.g. splitting a correct sub- Exhaustive bi-clustering (e.g. (Erosheva, Fienberg, and matrix into multiple smaller sub-matrices. Lafferty 2004; Kemp et al. 2006; Roy and Teh 2009; There are few works on this problem. One such Nakano et al. 2014)) is an extension of typical clustering work (Ben-Dor et al. 2003) suffers computational complex- Copyright c 2016, Association for the Advancement of Artificial ity equivalent to the cube to the number of columns; thus it Intelligence (www.aaai.org). All rights reserved. is feasible only for matrices with very few columns. Gu and 1By sub-matrix, we mean a direct product of a subset of row Liu (Gu and Liu 2008) adopted the model selection approach indices and a subset of column indices. based on BIC. However model selection inevitably requires 1701 Data matrix. Background Exhaustive Bi-clustering Baseline: (simplified) Bayesian Plaid model Partitions the entire matrix into many blocks. Bi-clustering has been studied intensively for years. A sem- Post-processing: inal paper (Cheng and Church 2000) has applied the bi- manually inspect all blocks clustering technique for the analysis of gene-expression :-( data. After that, many works have been developed (e.g. (Sha- balin et al. 2009; Fu and Banerjee 2009)). Among them, the Plaid model (Lazzeroni and Owen 2002) is recognized as one of the best bi-clustering methods in several review stud- Non-exhaustive and Overlapping (NEO) Bi-clustering ies (Eren et al. 2013; Oghabian et al. 2014). Bayesian mod- Extracts only “possibly interesting” sub-matrices: els of the plaid model have been also proposed and reported sharing coherent characteristics. effective (Gu and Liu 2008; Caldas and Kaski 2008). Post-processing: only a limited number of sub-matrices! We adopted a simplified version of Bayesian Plaid mod- :-) els (Caldas and Kaski 2008) as the baseline. The observed data is a matrix of N1 × N2 continuous values, and K is the Reduce man-hour cost! number of sub-matrices to model interesting parts. We de- fine the simplified Bayesian Plaid model as follows: λ ∼ λ, λ λ ∼ λ, λ , Our proposal; Infinite Bi-clustering 1,k Beta a1 b1 2,k Beta a2 b2 (1) NEO Bi-clustering with any number of sub-matrices. Infer the number of hidden sub-matrices automatically. z , , ∼ Bernoulli λ , , z , , ∼ Bernoulli λ , , (2) 1 i k 1 k 2 j k 2 k θ ∼ Normal μθ, (τθ)−1 ,φ∼ Normal μφ, (τφ)−1 , (3) k ⎛ ⎞ Figure 1: Exhaustive bi-clustering VS. NEO bi-clustering. ⎜ ⎟ ⎜ −1⎟ We offer the infinite bi-clustering: NEO bi-clustering with- xi, j ∼ Normal ⎝⎜φ + z1,i,kz2, j,kθk , (τ0) ⎠⎟ . (4) out knowing the exact number of distinctive sub-matrices. k Row and column indices are not consecutive: thus we do not In the above equations, k ∈{1,...,K} denotes sub-matrices, extract consecutive rectangles, but direct products of subsets i ∈{1,...,N } and j ∈{1,...,N } denote objects in the first of rows and columns. 1 2 (row) and the second (column) domains, respectively. λ1,k and λ2,k (Eq. (1)) are the probabilities of assigning an object to the kth sub-matrix in the first and the second domain. z1,i,k and z2, j,k in Eq. (2) represent the sub-matrix (factor) mem- berships. If z1,i,k = 1(0) then the ith object of the first do- multiple inference trials for different choices of model com- main is (not) a member of the kth sub-matrix, and similar plexities (the number of sub-matrices, K). This consumes a for z2, j,k. θk and φ in Eq. (3) are the mean parameters for the lot of computation and time resources. kth sub-matrix and the “background” factor. Eq. (4) com- bines these to generate an observation. Note that this obser- The main contribution of this paper is to propose a prob- vation process is simplified from the original Plaid models. abilistic model that allows infinite bi-clustering; NEO bi- Throughout the paper, however, we technically focus on the clustering without specifying the number of sub-matrices. modeling of Z thus we employ this simplified model. The proposed model is based on the plaid models (Lazze- As stated, existing bi-clustering methods including the roni and Owen 2002), which are known to be one of the Bayesian Plaid model require us to fix the number of sub- best bi-clustering methods (Eren et al. 2013; Oghabian et matrices, K, beforehand. It is very possible to choose a sub- al. 2014). The proposed Infinite Plaid models introduce a optimal K in practice since it is innately difficult to find the simple extension of the Indian Buffet Process (Griffiths and best K by hand. Ghahramani 2011) and can formulate bi-clustering patterns with infinitely many sub-matrices. We develop a MCMC in- Indian Buffet Process (IBP) ference that allows us to infer the appropriate number of Many researchers have studied sophisticated techniques for sub-matrices for the given data automatically. The inference exhaustive bi-clustering. Especially, the Bayesian Nonpara- does not require the finite approximation of typical varia- metrics (BNP) becomes a standard tool for the problem tional methods. Thus it can potentially address all possible of an unknown number of sub-matrices in exhaustive bi- numbers of sub-matrices, unlike the existing variational in- clustering (Kemp et al.
Details
-
File Typepdf
-
Upload Time-
-
Content LanguagesEnglish
-
Upload UserAnonymous/Not logged-in
-
File Pages8 Page
-
File Size-