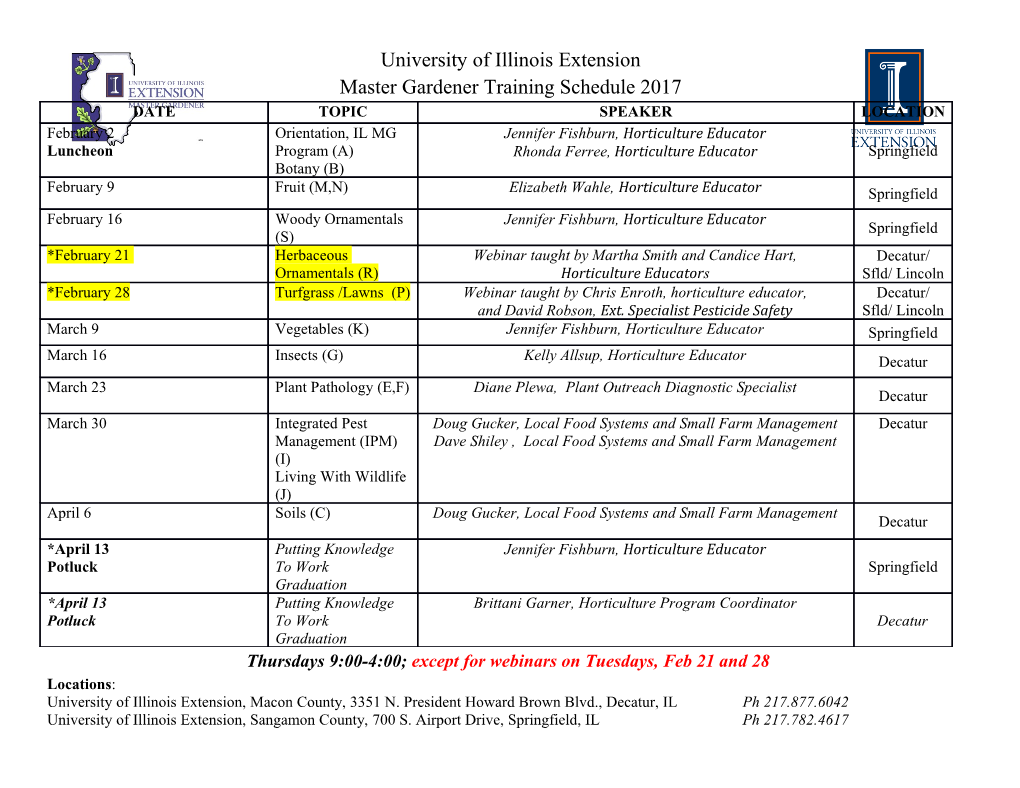
Small Area Estimation (SAE) for Non- sampled Areas Using Cluster Information Rahma Anisa Khairil Anwar Notodiputro Anang Kurnia (Bogor Agricultural University) The Problem A nasional survei by BPS (Center of National Statistic) Jakarta We need to estimate parameters in sub-district level for Bogor municipality and regency of West Java The Problem Out of 48 sub-districts of Bogor 2 sub-districts contain no sample Non-sampled sub-districts Mucipality We propose SAE of non-sampled parameters using additional cluster information and auxiliary variables Data Data source • SUSENAS is a national socio-economic survey conducted annually by BPS (Center of Statistic) • Multistage random sampling: All districts were chosen (complete) sub-districts (random sampling) households (pps samples) • PODES is an administrative record of village data in Indonesia published by BPS in every three years Variable of interest • Observed variable: average per capita expenditures per month in small area (sub-district) in regency and municipality of Bogor which is obtained from SUSENAS 2010 • Auxiliary variable: the number of minimarket in each village in regency and municipality of Bogor which is obtained from PODES 2011 • Sub-districts were defined as area levels and the villages were defined as unit level. Data Sub-districts Samples Population Sub-districts Samples Population Sub-districts Samples Population NANGGUNG 31 360 CISEENG 32 255 BOGOR SELATAN 107 764 LEUWILIANG 29 443 G. SINDUR 16 401 BOGOR TIMUR 50 318 PAMIJAHAN 47 506 RUMPIN 46 441 BOGOR UTARA 104 527 CIBUNGBULANG 31 411 CIGUDEG 32 539 BOGOR TENGAH 57 431 CIAMPEA 30 463 SUKAJAYA 16 284 BOGOR BARAT 142 790 DRAMAGA 32 315 JASINGA 16 456 TANAH SEREAL 105 629 CIOMAS 31 516 TENJO 48 194 LEUWISADENG 0 271 TAMANSARI 32 364 P. PANJANG 16 307 TENJOLAYA 0 158 HOUSEHOLDS CIJERUK 16 258 CIGOMBONG 15 295 CARINGIN 32 353 CIAWI 16 345 CISARUA 32 261 MEGAMENDUNG 16 257 SUKARAJA 30 542 BABAKAN MADANG 27 260 SUKAMAKMUR 32 249 CARIU 16 155 TANJUNGSARI 16 173 JONGGOL 47 362 CILEUNGSI 62 638 KELAPA NUNGGAL 16 215 GUNUNG PUTRI 76 1003 CITEUREUP 31 481 CIBINONG 58 943 BOJONG GEDE 43 662 TAJUR HALANG 15 356 KEMANG 15 319 RANCA BUNGUR 15 192 PARUNG 16 231 The Approach Small sample size EBLUP National Survey (sampled area) Zero sample size (non-sampled area) Alternative solution: Smaller Subpopulation EBLUP level Cluster Indirect Global model Information estimation prediction Development of The Models Basic Model MODEL-0 • Model for population • Prediction model for non-sampled area synthetic model which is a global model Existing modified EBLUP model MODEL-1 • Model for population • Prediction model for non-sampled area (Anisa et al. ,2014) Development of The Models Existing modified EBLUP model MODEL-2 • Model for population • Prediction model for non-sampled area (Anisa et al. ,2014) The Proposed Model • Model for population • Prediction model for non-sampled area Design of Simulation 44 sampled areas Population: 8 clusters 496 units 46 areas 2 non-sampled areas Steps : generate and calculate response variable Repeated Repeated times 1000 modeling the data and predict the parameter evaluate all models based on RB and RRMSE Simulation Results • Median of RB for non-sampled area (%) Model-0 Model-1 Model-2 Proposed Model 84.3268 74.7944 7.5564 -1.1512 • Median of RRMSE for non-sampled area (%) Model-0 Model-1 Model-2 Proposed Model 99.6123 98.1212 40.5751 38.9159 This results has indicated that proposed model showed the best performance compared with the others in predicting parameters of non- sampled area. Results of Application Clustering of sub-districts yielded eight clusters. This information have been incorporated into the proposed model under log-scale linear mixed model. • Estimates of per capita expenditures per month prediction of non-sampled area (Rupiah) Sub-district Standard EBLUP Model Proposed Model Leuwisadeng 518,357.70 321,482.90 Tenjolaya 489,113.10 317,559.90 • Estimates of Root Mean Squares Error (RMSE) over non-sampled area (Rupiah) Sub-district Standard EBLUP Model Proposed Model Leuwisadeng 146,196.79 133,605.09 Tenjolaya 135,239.97 105,700.52 smaller RRMSE Conclusion We conclude that cluster information can improve prediction of non- sampled areas by modifying the global synthetic model into local prediction models using the proposed model. References Anisa R, Kurnia A, Indahwati. 2014. Cluster Information of Non-Sampled Area in Small Area Estimation. IOSR Journal of Mathematics 10(1): 15-19. Das K, Jiang J, Rao JNK. 2004. Mean Square Error of Empirical Predictor. The Annals of Statistics 32(2): 818-840. Johnson RA, Wichern DW. 2007. Applied Multivariate Statistical Analysis 6th Edition. London: Prentice-Hall. Kurnia A. 2009. An empirical best prediction method for logarithmic transformation model in small area estimation with particular application to susenas data [doctoral disertation]. Bogor Agricultural University. Rao JNK. 2003. Small Area Estimation. New York: John Wiley & Sons. Saei A, Chambers R. 2005. Empirical Best Linear Unbiased Prediction for Out of Sample Area, Working paper M05/03, Southampton Statistical Sciences Research Institute..
Details
-
File Typepdf
-
Upload Time-
-
Content LanguagesEnglish
-
Upload UserAnonymous/Not logged-in
-
File Pages12 Page
-
File Size-