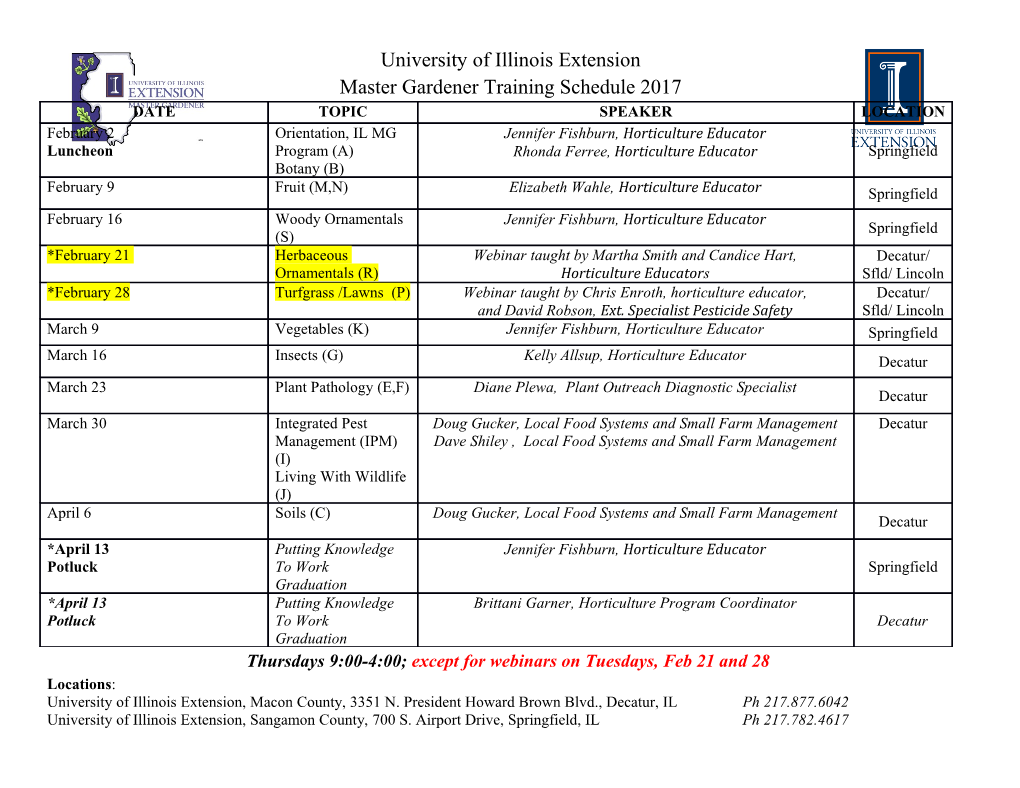
C HA PTER 4 2 BIOSTATISTICS 101 Stephan J. LaPointe, DPM, PhD INTRODUCTION distribution is what we learned in grade school when teachers graded our papers as the bell curve. The normal The assumption for the sake of this manuscript is that the distribution occurs whenever the measurement is the result reader has an interest in and understands the importance of small independent random factors. An individual statistics are currently playing in our podiatric medical measurement in the normal distribution is more likely to fall practice. This will only increase in the future. There is near the mean (or average) than far away from it and equally already more attention being paid to quality and value of likely to be above or below the mean. If the data are not clinical research. In addition efforts are already underway to likely to follow these criteria then the normal distribution measure our outcomes and prove that the care we provide is does not apply. This could occur if the students got the financially responsible and clinically meaningful. This new answers to the test and most scored at or near 100 since the understanding will significantly impact, change and improve data would be skewed and the scores cannot be above 100. how we practice our profession. All parties involved, especially our patients, will benefit. An entire manuscript could be Mean and Standard Deviation dedicated to this premise alone. Whenever the measurements meet the criteria of the This manuscript is based primarily and almost entirely on normal distribution two parameters can fully describe the a text Primer of Biostatistics, Fifth Edition, by Stanton A. data. They are the mean and the standard deviation. The Glantz, Ph.D. The book is well written and intended to be mean is simply the average of the data. read by medical professionals and researchers. This manuscript for the sake of brevity hits on the salient points without the examples and much of the prose found in the text. The intent is to provide the reader with quick reference when reviewing journal articles or about to embark in study of their own. Where µ is the mean, X is the arithmetic sum of the Certainly a more thorough explanation of the principles of individual measuremenƩts and N is the total number of statistics can be found in the Primer of Biostatistics. My measurements. recommendation would be to either purchase the fifth The second parameter, the standard deviation, describes edition or wait for the seventh edition. The latter is likely to how the data varies about the mean. To define the variability be released by the time you read this manuscript. This of the data we calculate how far away from the mean each does not cover all the topics in the text, but provides an measurement is by subtracting the mean from each value. introduction to some of the statistical methods contained We square this value since, if a measurement is below the therein that should start someone on their journey to mean, it results in a negative value. This is called the understanding statistics. population variance. PART 1: CHARACTERIZING DATA Population variance = sum of (measurement of one member – mean)2 / number of members Normal Distribution Part I will focus on ordinal data (data with ranking such as The equivalent mathematical expression is: numbers) that comply with the normal distribution since this includes most of the studies and data we will be exposed to. What is the normal distribution? The normal or Gaussian CHAPTER 42 215 Since the units of the population variance are squared (i.e. ( ) and the standard deviation of the sample means ( ). cm2 in the case of length measurements) we take the square The mean of the sample means will be equal to the mean root of the population variance to arrive at the standard µ of the entire population. However, the standard deviation. Mathematically this is expressed as: deviation of the sample means does not equal the standard deviation of the underlying population. It is a measure of the variability of the means not the underlying population. The means themselves cancel out much of the underlying variability of the population so this value will always be So now we have two parameters the mean and the standard smaller than the standard deviation of the population. This deviation that define the data assuming the data follow the value is called the standard error of the mean. The true normal distribution. standard error of the mean ( ) is defined as: Other characteristics of the normal distribution are that roughly 68 percent of the measurements fall within one standard deviation and about 95 percent fall within two standard deviations. where is the population standard deviation and n is the sampleσsize. Sample Mean and Standard Deviation The best estimate of the standard error of the mean, Now, in most studies it is impossible to measure all the s x, from one single sample is: subjects so we sample some of the population. If we measure only a subset of the population with n measurements of a possible total N we call this the sample mean denoted as and defined mathematically as: Central Limit Theorem One interesting characteristic of the distribution of the sample means is that it will follow the normal distribution The sample standard deviation is denoted as s (or SD) in (or bell curve) even if the underlying population data does the following equation: not. The standard error of the mean will be larger for larger variability of the underlying population as noted by the standard deviation. This value will be smaller for a larger sample size as shown in the last equation above. This and the characteristics of the sample means and standard error The difference between the sample mean and standard of the means has led to the central limit theorem. deviation and the population mean and standard deviation is that we are substituting the entire population mean µ with • The distribution of sample means will be approximately the sample mean and in calculating the sample standard normal regardless of the distribution of the values deviation we are dividing by n -1 instead of n. The reason for in the original population from which the samples the latter is that the sample will never show as much were drawn. variability as the entire population. By dividing by a value • The mean value of the collection of all possible sample less than n (i.e. n -1) we are increasing the standard means will equal the mean of the original population. deviation so we do not underestimate it. • The standard deviation of the collection of all possible means of samples of a given size, called the standard Standard Error of the Mean error of the mean, depends on both the standard and s are derived from a sample of n of the entire deviation of the original population and the size of population N. How close are the sample mean and the the sample. standard deviation of the sample to actual mean and standard deviation to the underlying population? We One note is to be careful when authors report their data they introduce an additional parameter to help with this called often substitute the standard error of the mean in place of the standard error of the mean. This statistic measures how the standard deviation when describing their data since the close the sample mean is to the underlying entire population former will be smaller. This, however, fools the reader to mean. We take say 50 samples of size n of N and calculate believing there is less variability of the population data and (here’s where it gets tricky) the mean of the 50 sample means is a serious error. 216 CHAPTER 42 PART II: TESTING FOR THE then there is more variance between the groups than DIFFERENCE BETWEEN GROUPS within the groups and therefore we can reject the null hypothesis. There is indeed a difference between the Tests of significance are how we determine whether a groups and therefore the samples were not drawn from the treatment has an effect on the underlying population. We same population, (ie, one of the groups is different due to first assume that there is no significant difference due to the the treatment effect). treatment or treatments and call it the null hypothesis. The tests used to determine whether to accept or reject the null The P value hypothesis are collectively called analysis of variance. How The value of the F test statistic will depend on which do we do this? individuals in the underlying population are tested. If we We estimate the variance in two ways. First we calculate were to repeat this experiment 100 times when there is no the average variance for all the groups and call it the within difference between the groups most of the values of F will be groups variance. We have eliminated the treatment effects. close to 1 but some will be greater than 1. We want to calculate the value of F for which there is only a 5% chance that we reject the null hypothesis when indeed the null hypothesis is true. This value of F is determined to be a “big F” and we report that the P <0.05 (for 5%). It is possible, The within groups variance is based on the mean for with sheer bad luck, to reject the null hypothesis 5% of the that sample and therefore is independent of and will not be time at that level of F affected by any of the treatments.
Details
-
File Typepdf
-
Upload Time-
-
Content LanguagesEnglish
-
Upload UserAnonymous/Not logged-in
-
File Pages7 Page
-
File Size-