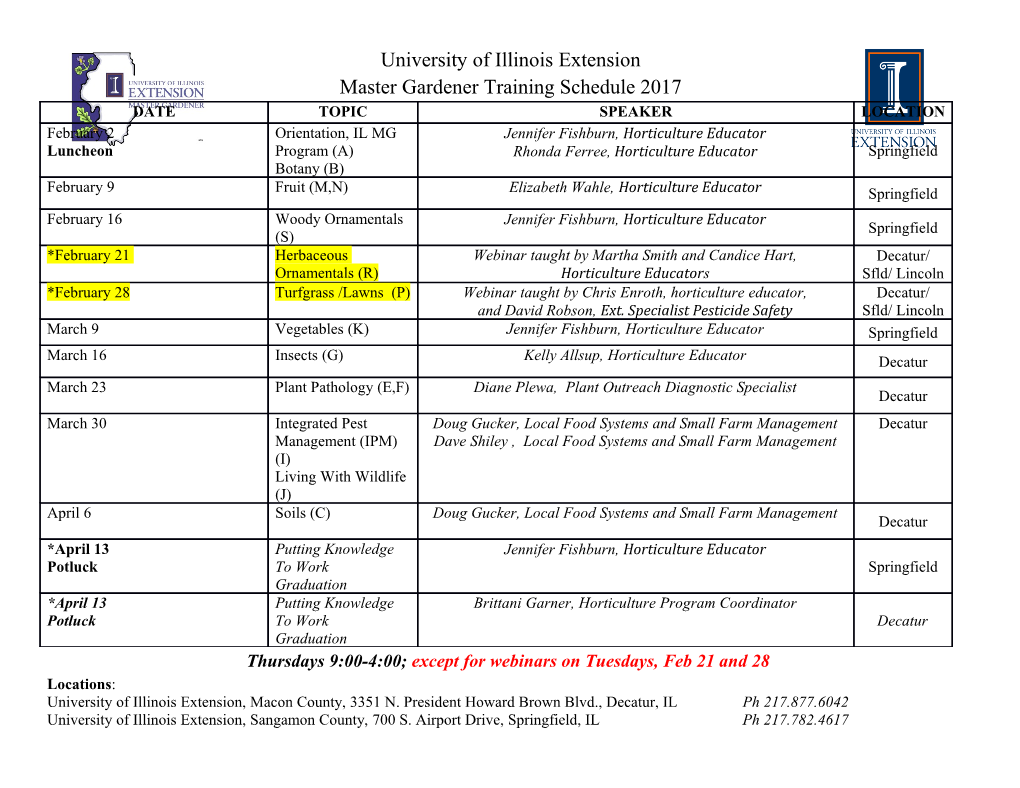
AUTOMATIC IDENTIFICATION AND REMOVAL OF LOW QUALITY ONLINE INFORMATION A Thesis Presented to The Academic Faculty by Steve Webb In Partial Fulfillment of the Requirements for the Degree Doctor of Philosophy in the College of Computing Georgia Institute of Technology December 2008 Copyright c 2008 by Steve Webb AUTOMATIC IDENTIFICATION AND REMOVAL OF LOW QUALITY ONLINE INFORMATION Approved by: Calton Pu, Advisor Ling Liu College of Computing College of Computing Georgia Institute of Technology Georgia Institute of Technology Mustaque Ahamad Shyhtsun Felix Wu College of Computing Department of Computer Science Georgia Institute of Technology University of California, Davis Nick Feamster Date Approved: 13 August 2008 College of Computing Georgia Institute of Technology For My Mother iii ACKNOWLEDGEMENTS The quest to obtain a Ph.D. is often characterized as a rollercoaster with numerous peaks and valleys, and as any Ph.D. candidate will tell you, I have experienced my fair share of those peaks and valleys. Fortunately, throughout the highest of highs and the lowest of lows, I have been surrounded by people that have offered constant encouragement and guidance. First and foremost, I am eternally grateful for the undying support that I have received from my family. I am particularly thankful for my mother, Anne, and my grandmother, Anna Ross. These two women have always been the most important people in my life, and without their unconditional love and support, I would not be the man I am today. Thank you both for everything you have done for me, and know that I will always love you. I am also thankful for my father, John. He taught me the importance of hard work and dedication, and I am truly grateful for those lessons. I love you, Dad. The rest of my family is equally amazing, and I truly appreciate their love and support. Beyond my immediate family, I am also blessed to be an honorary member of the Hammond and Little families. We might not be connected by blood, but I still love you with all of my heart. At Georgia Tech, my advisor, Calton Pu, was a constant source of inspiration. Regard- less of what graduate school or life threw at me, Calton was always there to put things in their proper perspective. In my very first meeting with Calton, he congratulated me for choosing personal and intellectual growth over the shortsighted allure of immediate financial gains. At the time, I have to admit that I thought he was crazy. But now, I understand exactly what he meant, and I cherish the journey we completed together. I am also grateful for Ling Liu’s guidance. At every one of my DISL or DoI presentations, I could always rely on her to make interesting observations about my work, and ultimately, her insights greatly enhanced my dissertation research. Leo Mark and H. Venkateswaran were also very positive influences at Tech. I could always expect a smile and a wave when I saw them on campus. iv I am indebted to Calton, Ling, Mustaque Ahamad, Nick Feamster, and Shyhtsun Felix Wu for reading and commenting on my dissertation. Their feedback was incredibly valuable, and I truly appreciate it. In addition to my committee members, I was fortunate enough to be exposed to a number of amazing people throughout my graduate career. Galen Swint and Kang Li were essentially my second and third advisors during my first year at Tech, and I appreciate every piece of advice they gave me. Subramanyam Chitti was an amazing collaborator, and I genuinely enjoyed every conversation, all-nighter, and Tin Drum trip we shared together. Jinpeng Wei and Younggyun Koh will always have a special place in my heart because we all endured the Systems Qualifier together. Li Xiong was an amazing officemate and an even better Master Shake. James Caverlee was one of the primary reasons I came to campus every day, and the three years we shared an office together were among the highlights of my life. Tim Garcia, Danesh Irani, and Qinyi Wu were also amazing officemates. I will miss tossing Frisbees with them all over the Klaus Building at all hours of the night. Finally, I am very thankful for the assistance I received from Deborah Mitchell, Mary Claire Thompson, and Susie McClain. I cherish my friendships with all of you, and I hope we will always stay in touch. v TABLE OF CONTENTS DEDICATION . iii ACKNOWLEDGEMENTS . iv LIST OF TABLES . ix LIST OF FIGURES . xi SUMMARY . xiv I INTRODUCTION . 1 II PRELIMINARIES . 9 2.1 Statistical Classification of Email Spam . 9 2.2 Dimensionality Reduction . 12 III THE IMPORTANCE OF LARGE CORPORA FOR EMAIL SPAM CLASSIFI- CATION . 14 3.1 Corpora Descriptions . 16 3.2 Evaluation of Small Corpora Training Sets . 22 3.3 Evaluation of Large Corpora Training Sets . 36 3.4 Related Work . 41 3.5 Summary . 43 IV LARGE-SCALE EVALUATION OF EMAIL SPAM CLASSIFIERS . 46 4.1 Experimental Setup . 47 4.2 Baseline Evaluation of Spam Filter Effectiveness . 50 4.3 Evaluation of Spam Filter Effectiveness Against Camouflaged Messages . 53 4.4 Baseline Evaluation of Spam Filter Effectiveness Revisited . 57 4.5 Related Work . 59 4.6 Summary . 60 V EVOLUTIONARY CHARACTERISTICS OF EMAIL SPAM . 61 5.1 Experimental Evaluation Method . 64 5.2 Evidence of Extinction . 68 5.3 Survival and Co-Existence . 72 5.4 Related Work . 79 vi 5.5 Summary . 80 VI DEFENDING CLASSIFIERS AGAINST CAMOUFLAGED EMAIL SPAM . 82 6.1 Background on the Spam Arms Race . 84 6.2 Related Work . 86 6.3 Baseline Evaluation of Learning Filters . 87 6.4 Evaluation of Camouflage Attacks . 90 6.5 Evaluation of Retrained Filter Defense . 94 6.6 Learning Filters Resisting Camouflage Attacks . 99 6.7 Summary . 106 VII INTEGRATING DIVERSE EMAIL SPAM FILTERING TECHNIQUES . 108 7.1 Related Work . 109 7.2 Description . 110 7.3 Implementation . 112 7.4 Summary . 116 VIII USING EMAIL SPAM TO IDENTIFY WEB SPAM AUTOMATICALLY . 117 8.1 The Webb Spam Corpus . 119 8.2 Sample Applications . 128 8.3 Summary . 136 IX CHARACTERIZING WEB SPAM USING CONTENT AND HTTP SESSION ANALYSIS . 138 9.1 Corpus Summary . 140 9.2 Content Analysis . 140 9.3 HTTP Session Analysis . 153 9.4 Related Work . 156 9.5 Summary . 157 X PREDICTING WEB SPAM WITH HTTP SESSION INFORMATION . 158 10.1 Related Work . 159 10.2 Web Page Retrieval Using HTTP . 161 10.3 Experimental Setup . 164 10.4 Webb Spam and WebBase Results . 171 vii 10.5 WEBSPAM-UK2006 Results . 176 10.6 Summary . 181 XI EXPLORING THE DARK SIDE OF SOCIAL ENVIRONMENTS . 182 11.1 Background on Social Networking Communities . 183 11.2 Traditional Attacks Targeting Social Networking Communities . 185 11.3 New Attacks Against Social Networking Communities . 194 11.4 Summary . 200 XII USING SOCIAL HONEYPOTS TO IDENTIFY SPAMMERS . 202 12.1 Related Work . 204 12.2 Social Spam . 205 12.3 Social Honeypots . 207 12.4 Social Honeypot Data Analysis . 209 12.5 Summary . 220 XIII CONCLUSIONS AND FUTURE RESEARCH DIRECTIONS . 222 13.1 Countering DoI Attacks in Email Systems . 222 13.2 Countering DoI Attacks in the World Wide Web . 224 13.3 Countering DoI Attacks in Social Environments . 225 REFERENCES . 227 VITA ............................................ 238 viii LIST OF TABLES 1 Corpus summaries. 17 2 Four combinations of training sets. 24 3 Stable false positive results for the Ling-spam spam and small legitimate training sets. 27 4 Stable false negative results for the Ling-spam spam and small legitimate training sets. 28 5 Stable false positive results for the SpamAssassin spam and small legitimate training sets. 29 6 Stable false negative results for the SpamAssassin spam and small legitimate training sets. 30 7 Stable false positive results for the SpamArchive spam and small legitimate training sets. 32 8 Stable false negative results for the SpamArchive spam and small legitimate training sets. 34 9 Stable false positive results for the small spam and Enron legitimate training sets. 35 10 Stable false negative results for the small spam and Enron legitimate training sets. 36 11 Stable false positive results for the SpamArchive spam and Enron legitimate training sets. 37 12 Stable false negative results for the SpamArchive spam and Enron legitimate training sets. 38 13 False positive results for various training and test set sizes. ..
Details
-
File Typepdf
-
Upload Time-
-
Content LanguagesEnglish
-
Upload UserAnonymous/Not logged-in
-
File Pages255 Page
-
File Size-