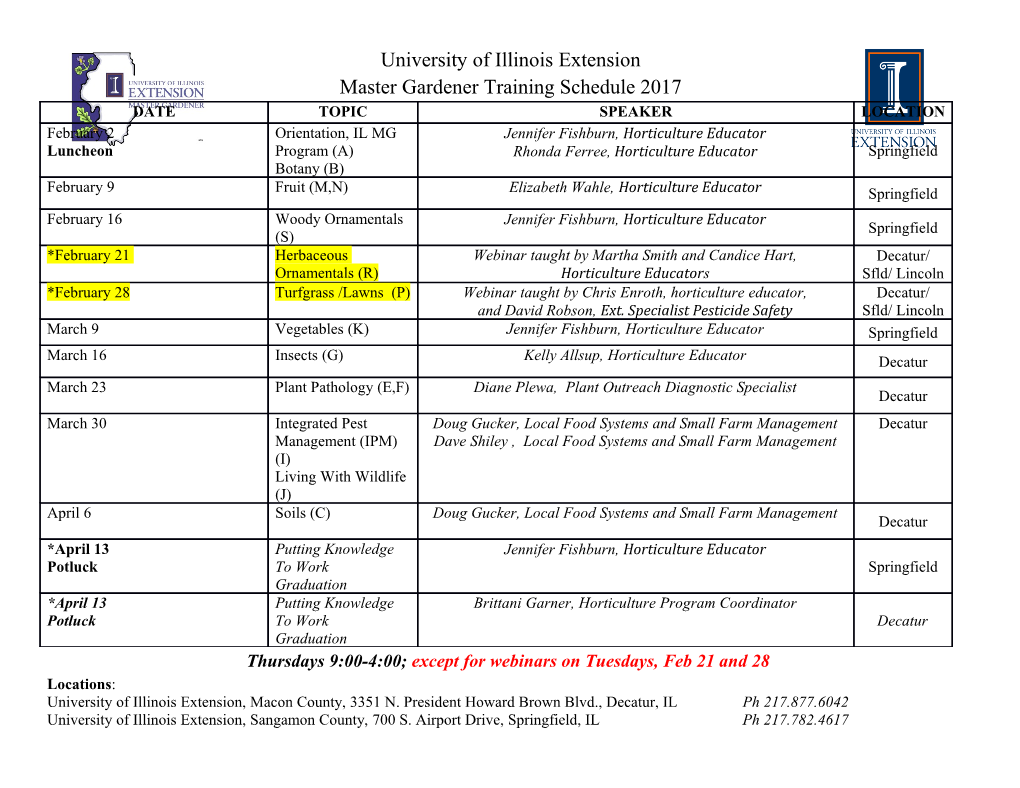
Basic Formulas in Probability Theory and Useful Identities Yevgeny Levanzov∗ Useful identities and notions. 1. 8p 2 R, (1 + p) ≤ ep. Mostly used in the form of (1 − p) ≤ e−p for p 2 [0; 1]. Thus, for t ≥ 0, we have that (1 − p)t ≤ e−tp. p n n 2. Stirling’s approximation: n! ∼ 2πn( e ) , where the sign ∼ means that the two quan- tities are asymptotic (that is, their ratio tends to 1 as n tends to infinity). n nk en k 3. k ≤ k! ≤ ( k ) . 2n n p 4. n = Θ(4 = n). 5. log n! = Θ(n log n). 6. We say that a property holds almost surely, if over a sequence of sets Sn, the prob- ability that Sn would have the property tends to 1 as n tends to infinity (notation for such probability: 1 − o(1)). 7. The averaging argument: let X be a random variable and µ = E[X] be its expectation. Then, X gets some value a ≥ µ with a positive probability (and the same goes for some value b ≤ µ). Conditional probability. Pr[A\B] • Definition: Let A; B be some events with Pr[B] > 0. Then: Pr[A j B] = Pr[B] . Pr[B j A]Pr[A] • Bayes’ law: Let A; B be some events with Pr[B] > 0. Then: Pr[A j B] = Pr[B] . ∗Department of Mathematics and Computer Science, Weizmann Institute of Science, Rehovot, Israel. E-mail: [email protected]. 1 The law of total probability. Let A be some event and B1;:::;Bk be a collection of pairwise disjoint events whose k union is the entire sample space (i.e., Pr[Bi \ Bj] = 0 for all i 6= j, and Pr[[i=1Bi] = 1). Then: k X Pr[A] = Pr[A j Bi]Pr[Bi] i=1 Linearity of expectation. Pk Let X1; :::; Xk be some random variables and a1; : : : ; ak 2 R, and let X = i=1 aiXi. Then: k k X X E[X] = E[ aiXi] = aiE[Xi] i=1 i=1 The law of total expectation. Let X; Y be some random variables. Then: E[X] = EY [EX [X j Y ]] Variance and standard deviation. Let X be a random variable. Its variance defined by: 2 2 Var(X) = E[X ] − (E[X]) Its standard deviation defined by: σ(X) = pVar(X) Pairwise and mutual independence. Let X1; :::; Xk be some random variables. • X1; :::; Xk are pairwise independent if for all i 6= j, and all a; b 2 R, we have that: Pr[Xi = a ^ Xj = b] = Pr[Xi = a]Pr[Xj = b] • X1; :::; Xk are mutually independent if for all a1; : : : ; ak 2 R, we have that: k k Y Pr[^i=1(Xi = ai)] = Pr[Xi = ai] i=1 2 Remark 1: the definition of k-wise independence is a straightforward generalization of the definition of pairwise independence. Remark 2: mutual independence implies k-wise independence, but not vice versa (counterexample: let X; Y; Z = X⊕Y , where all 3 are binary RVs, and X; Y are inde- pendent). Stochastic dominance. Let X; Y be some random variables. We say that X stochastically dominates Y , according to first-order stochastic dominance, if for all a 2 R, we have that: Pr[X ≥ a] ≥ Pr[Y ≥ a]; and for some a 2 R, we have that: Pr[X ≥ a] > Pr[Y ≥ a] The probabilistic method. A high-level description of what is the probabilistic method (due to Noga Alon): To prove an existence of a structure (or a substructure) with some properties, define a probability space of potential structures, and show that a random point in this space has all properties with positive probability. Lovász local lemma. Let A1;:::;Ak be a collection of events with Pr[Ai] ≤ p < 1 for all i 2 [k], and such that each event Ai is mutually independent of all but at most d of the other events. Then: • If 4pd ≤ 1, then there is a nonzero probability that none of the events occurs. • If ep(d + 1) ≤ 1, then there is a nonzero probability that none of the events occurs. Markov’s inequality. Let X be a nonnegative random variable with a positive finite expectation. Then: 1 8t ≥ 1; Pr[X ≥ t [X]] ≤ E t Chebyshev’s inequality. Let X be a random variable with finite variance σ2 > 0. Then: 3 1 8t ≥ 1; Pr[jX − [X]j ≥ tσ] ≤ E t Chernoff-Hoeffding concentration bounds. P Let X = i2[n] Xi where Xi 2 [0; 1] for i 2 [n] are independently distributed random variables. Then: −2t2=n 8t > 0; Pr[jX − E[X]j ≥ t] ≤ 2e ; −"2 [X]=2 80 < " ≤ 1; Pr[X ≤ (1 − ")E[X]] ≤ e E ; −"2 [X]=3 80 < " ≤ 1; Pr[X ≥ (1 + ")E[X]] ≤ e E ; −t 8t ≥ 2eE[X]; Pr[X ≥ t] ≤ 2 Union Bound (Boole’s inequality). Let A1;:::;Ak be a collection of events, such that Pr[Ai] ≤ pj for j 2 [k]. Then: k X Pr[A1 [ A2 [ ::: [ Ak] ≤ Pr[Ai]; i=1 and so: k X Pr[A1 \ A2 \ ::: \ Ak] ≥ 1 − Pr[Ai] i=1 Azuma’s inequality. Let X1; :::; Xk be a Martingale, such that jXi+1 − Xij ≤ 1 for all 0 ≤ i < m. Then: p −t2=2 Pr[jXm − X0j ≥ t m] ≤ 2e 4.
Details
-
File Typepdf
-
Upload Time-
-
Content LanguagesEnglish
-
Upload UserAnonymous/Not logged-in
-
File Pages4 Page
-
File Size-