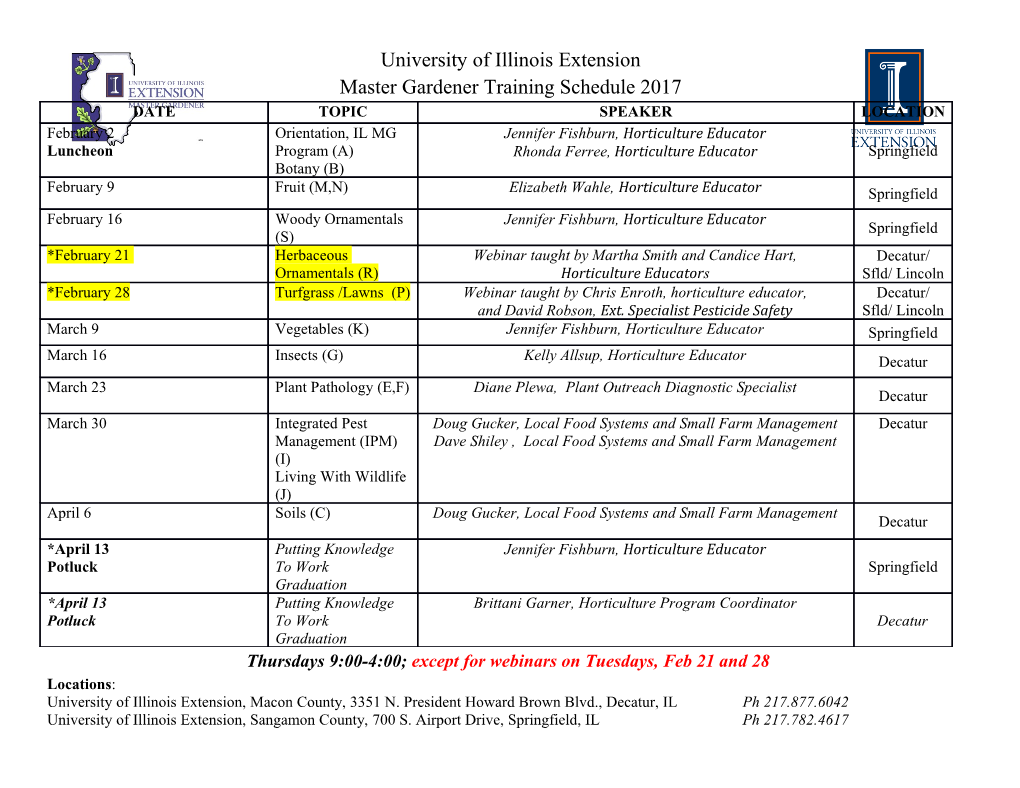
January 2003 Trevor Hastie, Stanford University 1 Boosting Trevor Hastie Statistics Department Stanford University Collaborators: Brad Efron, Jerome Friedman, Saharon Rosset, Rob Tibshirani, Ji Zhu http://www-stat.stanford.edu/∼hastie/TALKS/boost.pdf January 2003 Trevor Hastie, Stanford University 2 Outline • Model Averaging • Bagging • Boosting • History of Boosting • Stagewise Additive Modeling • Boosting and Logistic Regression • MART • Boosting and Overfitting • Summary of Boosting, and its place in the toolbox. Methods for improving the performance of weak learners. Classification trees are adaptive and robust, but do not generalize well. The techniques discussed here enhance their performance considerably. Reference: Chapter 10. January 2003 Trevor Hastie, Stanford University 3 Classification Problem Bayes Error Rate: 0.25 1 1 1 1 1 1 1 1 1 1 1 1 1 1 1 1 1 11 1 1 1 1 1 11 0 1 0 101 10 1 1 1 1 1 1 11 1 11 11 1 1 1 1 1 0 1 1 1 1 11 1 1 101 0 11 1 0 1 1 1 1 010 11 11 0 01 11 1 1 1 1 010 0 01 1001010 1 0 1 1 1 11 11 0 00001000101 00001111100 10 1 11101 10000100000 000001001011010 0 1 1 1 0 100 100100000010000010001 11 000010 000011000000 00010 1 1 1 1 11 1 1 1 00 010100010100000000101010 0000101 1101 1 1 1 1 0 11100000000101000011001 00000 1 1 1 1 11 1 10000000000010000000000010000101010010 11011 X2 1 1 1 00 00000 0 1000 0 1 0 0 10 0 00101000000001000010000010 1 1 1 111 1 11010100100000010001010010101010 1100 010 01 1 1 1 010 0010100000000 0001001101001 0 11 0 10110101101 000000000010 0 11 11 1 1 1 10 0101101000100000000 1 11 11 1 1 1 1 1 11 10 1 01 1000100 011 110 1 1 1 1 1 111111 1 110101010 1 11 1 111 1 1001 100 0 010 0 1 1 1 11 11 00 0 11 10 11 1 1 1 1 11101 01 1 1 1 111 1 1 1 1 1 1 11 1 1 1 11 1 1 1 1 1 1 111 1 1 11 1 1 1 1 1 1 1 1 1 1 1 11 1 1 1 1 1 1 1 1 1 -6-4-20246 1 1 -6 -4 -2 0 2 4 6 X1 Data (X, Y ) ∈ Rp ×{0, 1}. X is predictor, feature; Y is class label, response. (X, Y ) have joint probability distribution D. Goal: Based on N training pairs (Xi,Yi)drawn from D produce a classifier Cˆ(X) ∈{0, 1} Goal: choose Cˆ to have low generalization error R(Cˆ)=PD(Cˆ(X) = Y ) = ED[1(Cˆ(X)= Y )] January 2003 Trevor Hastie, Stanford University 4 Deterministic Concepts Bayes Error Rate: 0 1 1 1 11 1 1 1 1 1 11 11 1 11 1 1 1 1 11 1 1 1 1 11 1 1 1 1 1 1 1 1 11 1 1 11 1 1 1 1 1 1 111 11 1 1 1 1 1 11 11 11 111 11 111 1 1 1 1 1 1 111 1 1 1 1 11 1 0 00 1 11 1 1 1 1 1 11 11 11 11 000 0 0 1 11 1 11 1 1 1 11 000 00 01 1 1 1 1 000 0 01 11 1 1 1 1 111 00000 000 0000 00 0000 1 1 11 1 1 1 110000 0 0000 0 0 0 00 1 1 1 1 11 11110 000 00 0000000000000 0 11 1111 1 1 1 1000000 000 0 00 0 0 000 0 1 1 1 11 1100 00000000000 00000 0000 0 111 11 1 1 1 0 00000 000 00000000000000000 001 1 1 1 1 11 00000 0 000000 000000000 0 0000 11 1 1 11 1 1 X2 1 1 1 0 00 00000 0 0000000 0 0 1 1 1 0000 00000 0000000 0 000 1 1 11 1 100 0 00 0 00 0000000 000000 0111 1 1 11 1 11 1 000 000 000000 00 0 000 0 01111 1 1 11 1 00 00 0000000000000 000 00000 11111 1 1 1 1 1 11 0 0000000000 000 11 1 1 1 1 1 11 1 0 000000000000000 0 0 1 111 11 1 11 11000000 000 00 00 11 1 1 1 1 1 1 111 00 0 0 1 11111 1 1 1 1 1 11 00 00 0000 11 1 1 1 11 11 1 111 1 1 1 1 1 1 1 111 1111 11 11 1 1 1 1 11 11 11 1 111 1 11 111 1 1 11 1 1 1 1 1 1111 1 1 1 1 1 1 1 1 1 1 11 1 1 1 111 1 1 1 1 1 1 1 1 11 -3-2-10123 -3 -2 -1 0 1 2 3 X1 X ∈ Rp has distribution D. C(X)isdeterministic function ∈ concept class. Goal: Based on N training pairs (Xi,Yi = C(Xi)) drawn from D produce a classifier Cˆ(X) ∈{0, 1} Goal: choose Cˆ to have low generalization error R(Cˆ)=PD(Cˆ(X) = C(X)) = ED[1(Cˆ(X)= C(X))] January 2003 Trevor Hastie, Stanford University 5 Classification Tree Sample of size 200 1 94/200 x.2<-1.06711 x.2>-1.06711 1 0 0/34 72/166 x.2<1.14988 x.2>1.14988 0 1 40/134 0/32 x.1<1.13632 x.1>1.13632 0 1 23/117 0/17 x.1<-0.900735 x.1>-0.900735 1 0 5/26 2/91 x.1<-1.1668 x.2<-0.823968 x.1>-1.1668 x.2>-0.823968 1 1 0 0 0/12 5/14 2/8 0/83 x.1<-1.07831 x.1>-1.07831 1 1 1/5 4/9 January 2003 Trevor Hastie, Stanford University 6 Decision Boundary: Tree Error Rate: 0.073 1 1 1 1 1 1 1 1 1 1 1 1 1 1 1 1 1 1 0 11 1 11 1 1 0 0 1 1 1 0 11 1 1 0 0 0 000 1 1 1 00 0 0 0 00 1 0 0 0 000 0 1 0 0 0 0 11 1 0 0 0 1 1 1 1 0 0 0 0 0 0 1 1 1 1 0 0 0 0 0 0 00 X2 0 0 0 0000 00 0 0 0 0 1 1 0 00 0000 0 0 0 0 0 00 0 00 00 00 1 000 0 1 1 1 000 000 0 0 0 1 1 0 0 00 1 1 1 1 0 10 1 1 1 1 01 11 1 1 1 1 1 1 11 1 1 1 11 1 1 1 1 1 1 1 11 1 1 1 1 1 1 1 -3-2-10123 -3 -2 -1 0 1 2 3 X1 When the nested spheres are in IR10,CART produces a rather noisy and inaccurate rule Gˆ(X), with error rates around 30%. January 2003 Trevor Hastie, Stanford University 7 Model Averaging Classification trees can be simple, but often produce noisy (bushy) or weak (stunted) classifiers. • Bagging (Breiman, 1996): Fit many large trees to bootstrap-resampled versions of the training data, and classify by majority vote. • Boosting (Freund & Shapire, 1996): Fit many large or small trees to reweighted versions of the training data. Classify by weighted majority vote. In general Boosting Bagging Single Tree. “AdaBoost ··· best off-the-shelf classifier in the world” — Leo Breiman, NIPS workshop, 1996. January 2003 Trevor Hastie, Stanford University 8 Bagging Bagging or bootstrap aggregation averages a given procedure over many samples, to reduce its variance — a poor man’s Bayes. See pp 246. Suppose G(X,x) is a classifier, such as a tree, producing a predicted class label at input point x. To bag G, we draw bootstrap samples X∗1,...X∗B each of size N with replacement from the training data. Then ˆ { ∗b }B Gbag(x) = Majority Vote G(X ,x) b=1. Bagging can dramatically reduce the variance of unstable procedures (like trees), leading to improved prediction. However any simple structure in G (e.g a tree) is lost. January 2003 Trevor Hastie, Stanford University 9 Original Tree Bootstrap Tree 1 0 0 10/30 7/30 x.2<0.39 x.2<0.36 x.2>0.39 x.2>0.36 0 1 0 1 3/21 2/9 1/23 1/7 x.3<-1.575 x.1<-0.965 x.3>-1.575 x.1>-0.965 1 0 0 0 2/5 0/16 1/5 0/18 Bootstrap Tree 2 Bootstrap Tree 3 0 0 11/30 4/30 x.2<0.39 x.4<0.395 x.2>0.39 x.4>0.395 0 1 0 0 3/22 0/8 2/25 2/5 x.3<-1.575 x.3<-1.575 x.3>-1.575 x.3>-1.575 1 0 0 0 2/5 0/17 2/5 0/20 Bootstrap Tree 4 Bootstrap Tree 5 0 0 13/30 12/30 x.2<0.255 x.2<0.38 x.2>0.255 x.2>0.38 0 1 0 1 2/16 3/14 4/20 2/10 x.3<-1.385 x.3<-1.61 x.3>-1.385 x.3>-1.61 0 0 1 0 2/5 0/11 2/6 0/14 January 2003 Trevor Hastie, Stanford University 10 Decision Boundary: Bagging Error Rate: 0.032 1 1 1 1 1 1 1 1 1 1 1 1 1 1 1 1 1 1 0 11 1 11 1 1 0 0 1 1 1 0 11 1 1 0 0 0 000 1 1 1 00 0 0 0 00 1 0 0 0 000 0 1 0 0 0 0 11 1 0 0 0 1 1 1 1 0 0 0 0 0 0 1 1 1 1 0 0 0 0 0 0 00 X2 0 0 0 0000 00 0 0 0 0 1 1 0 00 0000 0 0 0 0 0 00 0 00 00 00 1 000 0 1 1 1 000 000 0 0 0 1 1 0 0 00 1 1 1 1 0 10 1 1 1 1 01 11 1 1 1 1 1 1 11 1 1 1 11 1 1 1 1 1 1 1 11 1 1 1 1 1 1 1 -3-2-10123 -3 -2 -1 0 1 2 3 X1 Bagging averages many trees, and produces smoother decision boundaries.
Details
-
File Typepdf
-
Upload Time-
-
Content LanguagesEnglish
-
Upload UserAnonymous/Not logged-in
-
File Pages43 Page
-
File Size-