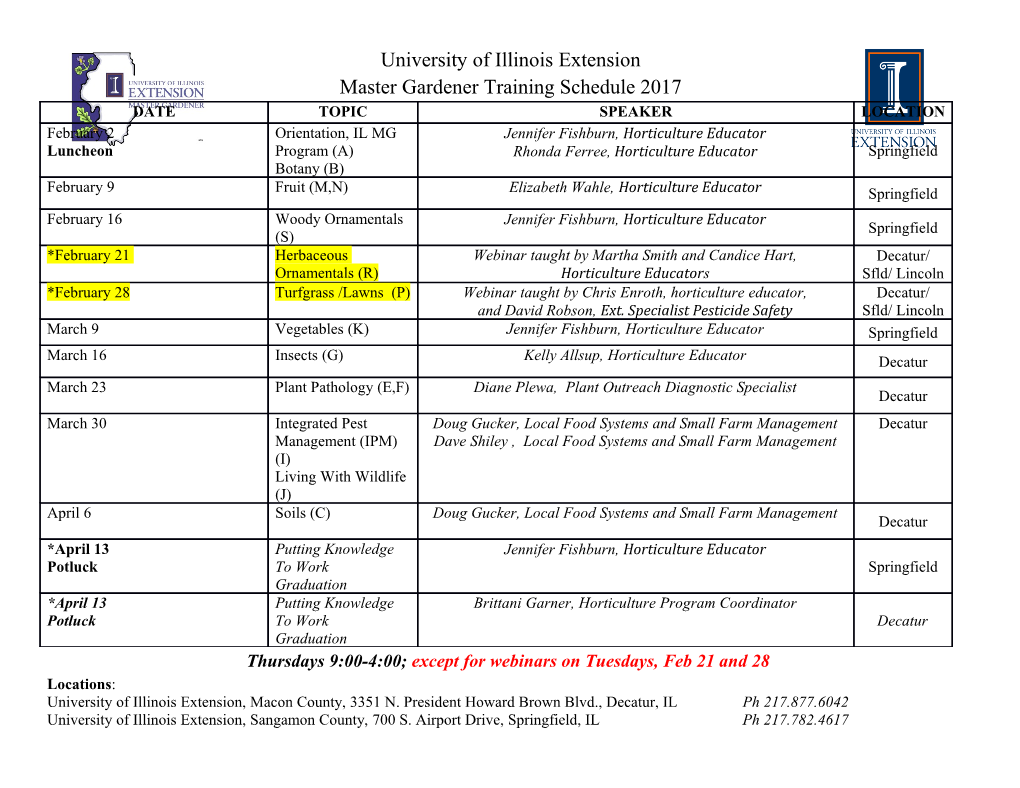
Wavelets and filterbanks Mallat 2009, Chapter 7 Outline • Wavelets and Filterbanks • Biorthogonal bases • The dual perspective: from FB to wavelet bases – Biorthogonal FB – Perfect reconstruction conditions • Separable bases (2D) • Overcomplete bases – Wavelet frames (algorithme à trous, DDWF) – Curvelets Wavelets and Filterbanks Wavelet side Filterbank side • Scaling function • Perfect reconstruction – Design (from multiresolution conditions (PR) priors) – Reversibility of the transform – Signal approximation • Equivalence with the – Corresponding filtering operation conditions on the wavelet § Condition on the filter h[n] → filters Conjugate Mirror Filter (CMF) – Special case: CMFs → • Corresponding wavelet Orhogonal wavelets families – General case → Biorthogonal wavelets Wavelets and filterbanks • The decomposition coefficients in a wavelet orthogonal basis are computed with a fast algorithm that cascades discrete convolutions with h and g, and subsample the output • Fast orthogonal WT ft() a [ n ] ( t n ) V =∑ 00ϕ −∈ n Since (t-n) is an orthonormal basis {ϕ }n∈Ζ +∞+∞ an[]= ft (),(ϕϕ t− n )= ft ()** ( t− ndt )= ft () ϕϕ ( n− tdtf )= * () n 0 ∫∫ −∞ −∞ ϕϕ()tt= (− ) Linking the domains z = e jω fˆ(ω) = fˆ(e jω ) ↔ f (z) Switching between the fˆ(ω +π ) = fˆ(e j(ω+π )) = fˆ(−e jω ) ↔ f (−z) Fourier and the z-domain fˆ(−ω) = fˆ(e− jω ) ↔ f (z−1) fˆ*(ω) = fˆ(−ω) ↔ f (z−1) +∞ f [n]↔ f (z) = ∑ f [k]z−k k=−∞ f [n −1]↔ z−1 f (z) unit delay Switching between the time and the z-domain f [−n]↔ f (z−1) reverse the order of the coefficients (−1)n f [n]↔ f (−z) negate odd terms Fast orthogonal wavelet transform • Fast FB algorithm that computes the orthogonal wavelet coefficients of a discrete signal a0[n]. Let us define ft()=∑ an00 [ ]ϕ ( t−∈ n) V n Since ϕ tn − is orthonormal, then { ( )}n∈Ζ an0[]= ft (),(ϕϕ t− n )= f∗ () n since is an orthonormal basis for V anjjn[]= f ,ϕ , ϕ jn, j dnjjn[]= f ,ψ , • A fast wavelet transform decomposes successively each approximation PVjf in the coarser approximation PVj+1f plus the wavelet coefficients carried by PWj+1f. • In the reconstruction, PVjf is recovered from PVj+1f and PWj+1f for decreasing values of j starting from J (decomposition depth) Fast wavelet transform • Theorem 7.7 – At the decomposition +∞ (1) a j+1[ p] = ∑h[n − 2 p]a j[n] = a j ∗ h[2 p] n=−∞ +∞ (2) d j+1[ p] = ∑ g[n − 2 p]a j[n] = a j ∗ g[2 p] n=−∞ – At the reconstruction +∞ +∞ a j[ p] = ∑ h[ p − 2n]a j+1[n] + ∑ g[ p − 2n]d j+1[n] =a j+1 ∗ h[n] + d j+1∗ g[n] (4) n=−∞ n=−∞ ⎧x[ p] n = 2 p x[p] x[n] = ⎨ ⎩ 0 n = 2 p +1 x"!n$# Proof: decomposition (1) [pV ] V [ p ] [ p ], [ n ] [ n ] (b) ϕϕϕϕϕjjjj++11∈⊂→ + 1 =∑ jjj + 1 n but j+1 j 112⎛⎞tp− 2 * ⎛⎞tn− ϕϕjj+1[p ], [ n ] = ϕ⎜⎟ ϕ⎜⎟ dt (a) ∫ jj+1 jj+1 22⎝⎠22⎝⎠ let tp− 2 j+1 t ' ttpttptpt'2=−jjjjj−→ 2=+ 2'2++11 →− 2= 2'→= 2 j+1 2 then ⎛⎞tp− 2 j+1 ⎛⎞t ' ϕϕ⎜⎟j+1 = ⎜ ⎟ ⎝⎠2 ⎝⎠2 ⎛⎞tn− 2 j **tpn'2 tt'' tt t ϕϕ⎜⎟j =+( −) =−→pptp=+→=+'2 ⎝⎠2 2222jj++11 2 j replacing into (a) 1'tt 1 (3) [p ], [ n ]⎛⎞* t ' 2 p n dt ' ⎛⎞ , t 2 p n h [ n 2 p ] ϕϕjj+1 =+ ϕϕ⎜⎟( −) =+ ϕϕ ⎜⎟ ( −) =− ∫ 22⎝⎠22 ⎝⎠ thus (b) becomes []phnpn [ 2] [] ϕϕjj+1 =∑ − n Proof: decomposition (2) qui • Coming back to the projection coefficients +∞ a [ p] f , f , h[n 2 p] f h[n 2 p] * dt j+1 = ϕ j+1,p = ∑ − ϕ j,n = ∫ ∑ − ϕ j,n = n −∞ n +∞ h[n 2 p] f (t) * (t)dt h[n 2 p] f , h[n 2 p]a [n] = ∑ − ∫ ϕ j,n = ∑ − ϕ j,n = ∑ − j → n −∞ n n a j+1[ p] = a j ∗h[2 p] • Similarly, one can prove the relations for both the details and the reconstruction formula Proof: decomposition (3) • Details WV , ψψψϕϕjp++1,∈⊂→ j 1 j jp + 1,=∑ jn + 1, jnjn , , n ttp' =22− j −→ 1 ⎛⎞t (3bis) ψϕjn+1,,,22 jn , = ψ⎜⎟ ϕ(tn−+= p) gn[ −→ p] 2 ⎝⎠2 gn2 p ψϕjp+1,=∑ [ −→] jn , n fgnpf,2, ψϕjn+1,=∑ [ −] jn , → n dp gnpan2 jj+1 [ ] =∑ [ −] [ ] n Proof: Reconstruction Since Wj+1 is the orthonormal complement of Vj+1 in Vj, the union of the two respective basis is a basis for Vj. Hence VV W ,, jj=++11,⊕→ jϕϕϕϕϕψψ jp=∑∑ jpjnjn ,1,1,,1,1, ++ + jpjnjn ++ nn but (see (3) and (3bis), the analogous one for g) ϕϕjp,1,,[2] j+ n =hp− n ϕψjp,1,,[2] j+ n =gp− n thus hp[2] n gp [2] n ϕϕjp,1,1,=∑∑− j++ n+− ψ j n nn Taking the scalar product with f at both sides: +∞ +∞ a j[ p] = ∑ h[ p − 2n]a j+1[n] + ∑ g[ p − 2n]d j+1[n] =a j+1 ∗ h[n] + d j+1∗ g[n] CVD n=−∞ n=−∞ ⎧x[ p] n = 2 p x[n] = ⎨ ⎩ 0 n = 2 p +1 Graphically a j [p] = ∑h[p − 2n]a j+1 [n] = ∑a j+1 [n]h[p − 2n] n n a j [0] = ∑h[−2n]a j+1 [n] = ∑a j+1 [n]h[−2n] n n Let's assume that h is symmetric a j [0] = ∑a j+1 [n]h[2n] n aj+1[n] 0 1 2 n h[n] 0 1 2 n Graphically a j+1 [n] 0 1 2 n h[n] 0 1 2 n a j [0] = ∑a j+1 [n]h[2n] = ∑a j+1 [n]h[n] n n Summary a j+1[ p] = a j ∗h[2 p] • The coefficients aj+1 and dj+1 are computed by taking every other sample of the convolution of aj with h and g respectively. • The filter h removes the higher frequencies of the inner product sequence aj , whereas g is a high-pass filter that collects the remaining highest frequencies. • The reconstruction is an interpolation that inserts zeroes to expand aj+1 and dj+1 and filters these signals, as shown in Figure. Filterbank implementation _ a2 h ↓2 _ a1 h ↓2 _ a0 g ↓2 _ d2 g ↓2 d1 a2 ↑2 h a1 + ↑2 h ă0 ↑2 g + d2 ↑2 g d1 Fast DWT • Theorem 7.10 proves that aj+1 and dj+1 are computed by taking every other sample of the convolution on aj with h and g respectively • The filter h removes the higher frequencies of the inner product and the filter g is a band- pass filter that collects such residual frequencies • An orthonormal wavelet representation is composed of wavelet coefficients at scales 12≤≤jJ 2 plus the remaining approximation at scale 2J ⎡⎤ dajJ, ⎣⎦⎢⎥{ }1≤≤jJ Summary Analysis or decomposition Synthesis or reconstruction h ↓2 a j +1 ↑2 h a j a j g ↓2 d j +1 ↑2 g Teorem 7.2 (Mallat&Meyer) and Theorem 7.3 [Mallat&Meyer] 2 2 ∀ω ∈ , hˆ(ω) + hˆ(ω + π ) = 2 and hˆ(0) = 2 gehˆ()ωωπ=+−−jnω ˆ*1 ( )↔ gnhn []= (1)[1]−− The fast orthogonal WT is implemented by a filterbank that is completely specified by the filter h, which is a CMF The filters are the same for every j Filter bank perspective _ h ↓2 ↑2 h a0 ă0 + _ g ↓2 ↑2 g Taking h[n] as reference (which amounts to choosing the synthesis low-pass filter) the following relations hold for an orthogonal filter bank: hn[]= h [− n ] gn[]= (1)−11−−nn h [1 − n ]= (1)− hn [ − 1] gn[]= g [− n ]= (1)−−−(1n ) h [(1 −− n )] neglecting the unitary shift, as usually done in applications gn[]= (1)−−−nn h [ − n ]= (1)− hn[] gn[]= g [− n ]= (1)[]−n hn Finite signals • Issue: signal extension at borders • Possible solutions: – Periodic extension § Works with any kind of wavelet § Generates large coefficients at the borders – Symmetryc/antisymmetric extension, depending on the wavelet symmetry § More difficult implementation § Haar filter is the only symmetric filter with compact support – Use different wavelets at boundary (boundary wavelets) – Implementation by lifting steps Wavelet graphs Orthogonal wavelet representation • An orthogonal wavelet representation of aL=< f ,ϕL,n> is composed of wavelet coefficients of f at scales 2L<2j<=2J , plus the remaining approximation at the largest scale 2J : • Initialization – Let b[n] be the discrete time input signal and let N-1 be the sampling period, such that the corresponding scale is 2L=N-1 – Then: N-1: discrete sample distance 2L= N-1 scale original continuous interpolation function time signal discrete time signal Initialization -1 following the definition: N : discrete sample distance 2L= N-1 scale 12⎛⎞tn− L ϕϕLn, = ⎜⎟L Basis for VL 2L ⎝⎠2 −−11 L 111/2 ⎛⎞⎛⎞tNn−− tNn 1 2 NN N =→==→ϕϕLn,,=⎜⎟⎜⎟−−11→ ϕ= ϕ Ln N 2L ⎝⎠⎝⎠NNN but +∞⎛⎞tNn− −1 1 +∞ ft bn bn t ( ) ==∑∑[ ]ϕϕ⎜⎟−1 [ ] Ln, ( ) nn=−∞⎝⎠N N =−∞ ⎛⎞tNn− −1 11 bn f,, f a n an f, [ ] ===ϕϕ⎜⎟−1 Ln, L[ ] LLn[ ] = ϕ , ⎝⎠N NN since +∞ ⎛⎞tNn− −1 a n= f t Nϕ dt by definition, then L [ ] ∫ ( ) ⎜⎟−1 −∞ ⎝⎠N if f is regular, the sampled values can be considered as a local average in the an NfNn−1 L [ ] ≈ ( ) neighborhood of f(N-1n) The filter bank perspective Perfect reconstruction FB • Dual perspective: given a filterbank consisting of 4 filters, we derive the perfect reconstruction conditions ~ h ↓2 a 1 ↑2 h a0 a0 g ~ ↓2 d 1 ↑2 g • Goal: determine the conditions on the filters ensuring that a0 ≡ a0 PR Filter banks • The decomposition of a discrete signal in a multirate filter bank is interpreted as an expansion in l2(Z) since al[] a * h 2 l a nh 2 l n a nhn 2 l 10==[ ] ∑∑ 0[ ] [ −] = 0[ ] [ −] nn then and the signal is recovered by the reconstruction filter dual family of vectors thus points to biorthogonal wavelets The two families are biorthogonal Thus, a PR FB projects a discrete time signals over a biorthogonal basis of l2(Z).
Details
-
File Typepdf
-
Upload Time-
-
Content LanguagesEnglish
-
Upload UserAnonymous/Not logged-in
-
File Pages53 Page
-
File Size-