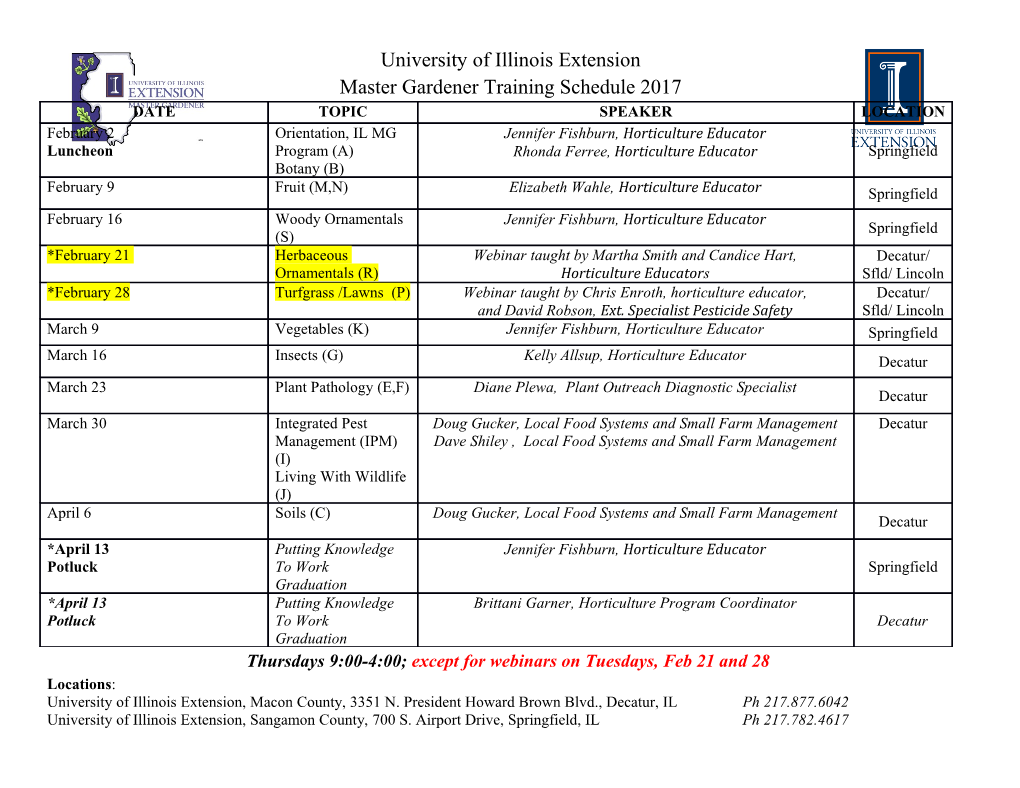
Pulm Ther (2016) 2:19–41 DOI 10.1007/s41030-016-0017-z REVIEW Identification of Asthma Subtypes Using Clustering Methodologies Matea Deliu . Matthew Sperrin . Danielle Belgrave . Adnan Custovic Received: April 28, 2016 / Published online: June 22, 2016 Ó The Author(s) 2016. This article is published with open access at Springerlink.com ABSTRACT stratification of patients, thus enabling more precise therapeutic and prevention approaches. Asthma is a heterogeneous disease comprising a number of subtypes which may be caused by different pathophysiologic mechanisms Keywords: Adult asthma; Asthma; Clustering; (sometimes referred to as endotypes) but may Endotypes; Paediatric asthma; Phenotypes share similar observed characteristics (phenotypes). The use of unsupervised clustering in adult and paediatric populations INTRODUCTION has identified subtypes of asthma based on Asthma is a heterogeneous disease, defined by observable characteristics such as symptoms, the most recent Global Initiative for Asthma lung function, atopy, eosinophilia, obesity, and (GINA) global strategy for asthma management age of onset. Here we describe different and prevention consensus as a condition clustering methods and demonstrate their characterised by the presence of respiratory contributions to our understanding of the symptoms such as wheeze, shortness of breath, spectrum of asthma syndrome. Precise chest tightness and cough that vary over time identification of asthma subtypes and their and in intensity, together with variable airflow pathophysiological mechanisms may lead to obstruction [1]. However, various definitions of Enhanced Content To view enhanced content for this asthma do not capture the heterogeneity of this article, go to http://www.medengine.com/Redeem/48D 4F06023BDDEA2. common complex condition. It is becoming increasingly clear that asthma is not a single M. Deliu (&) Á M. Sperrin disease, but a syndrome which consists of a Centre for Health Informatics, Institute of Population Health, University of Manchester, number of disease subtypes with similar Manchester, UK observable clinical characteristics [2]. These e-mail: [email protected] observable characteristics of the disease are D. Belgrave Á A. Custovic often referred to as asthma phenotypes. The Department of Paediatrics, Imperial College London, London, UK term ’asthma endotype’ is not synonymous 20 Pulm Ther (2016) 2:19–41 with phenotype, and it should be used to refer lung function and subsequent development of to the distinct disease entity under the umbrella asthma [11], their distinct underlying diagnosis of asthma, which has defined pathophysiological mechanisms have not been pathophysiological mechanisms that give rise elucidated or confirmed—they cannot be to clinical symptoms [3]. It should be considered as endotypes. emphasised that the same observable Based on expert opinion and consensus, characteristic (i.e. phenotype) can arise as a Lotvall et al. [4] suggested the existence of six consequence of different underlying asthma endotypes: aspirin-sensitive asthma, pathologies (i.e. endotypes), which is allergic bronchopulmonary mycosis, allergic consistent with observations showing that asthma, asthma predictive index-positive there are subtypes of asthma that share similar preschool wheezers, severe late-onset clinical symptoms but have differing hypereosinophilic asthma, and asthma in underlying pathophysiological mechanisms cross-country skiers. However, the well-defined [4]. There are numerous examples in other pathophysiological mechanisms and disease areas of a similar or identical clinical biomarkers which differentiate these proposed presentation arising as a consequence of endotypes have not been discovered, and there different pathology (e.g. fever in childhood is no universal agreement that these subtypes of can be caused by numerous different asthma represent true endotypes [12]. At this mechanisms). time, the endotype concept remains largely The traditional constructs of ‘asthma hypothetical, but may have a tangible value in phenotypes’ have been largely descriptive, helping us to formulate strategies to better with little uniformity, and usually informed by understand the mechanisms underlying subjective observations of single dimensions of different asthma-related diseases, and thus to the disease, such as triggering factors (e.g. identify more effective stratified treatment extrinsic and intrinsic asthma [5], strategies [13]. exercise-induced asthma [6]), patterns of In recent years, approaches to subtyping airway obstruction (e.g. reversible and asthma have evolved from subjective expert irreversible asthma [7]), or pathology (e.g. opinion to more data-driven methodologies eosinophilic and non-eosinophilic asthma [8]). such as machine learning [14, 15]. Statistical In paediatric asthma, changes over time in machine-learning methods facilitate the symptoms such as wheeze have been used to efficient exploration of data for the define phenotypes of wheezing illness during identification and analysis of disease patterns. childhood [9]. For example, based on clinical These methods are able to draw upon the vast observation of changes in the temporal pattern array of data generated from birth and patient of wheezing illness during childhood, as cohorts in order to cluster, classify, regress, and confirmed in the birth cohort study (Tucson make predictions from data based on inherent Children’s Respiratory Study), Martinez et al. patterns within the large complex data set. This divided children into three groups (or is in contrast to the traditional methods based phenotypes) of wheezing: transient early on human observation and testing of wheezers, late-onset wheezers, and persistent hypotheses using prior knowledge. Within the wheezers [10]. Although these phenotypes are context of asthma subtyping, methods such as clinically meaningful in their association with unsupervised clustering approaches, factor Pulm Ther (2016) 2:19–41 21 analysis, and principal component analysis SELECTION OF VARIABLES/ come into wide use within the last decade. FEATURES AND DIMENSION These are hypothesis-generating, with the REDUCTION overarching notion that the inherent patterns within the data may be a reflection of different Cluster analysis lacks the ability to differentiate underlying aetiologies, genetic basis, and/or between clinically relevant and irrelevant immunopathophysiologies, and that identified variables; thus the choice of variables to input clusters may represent distinct asthma into the clustering algorithm is one of the most endotypes. If this assumption is correct, important considerations. Variable or feature clustering methodologies could facilitate better selection can be performed subjectively or understanding of the disease mechanisms, objectively. Subjective methods involve identification of novel therapeutic targets, and choosing relevant variables based on expert better clinical trial design incorporating advice and published work. In contrast, group-specific targeted treatment, all of which objective methods use data-driven approaches are essential steps towards delivery of stratified to variable/feature selection, the most common medicine in asthma. of which are stepwise methods (such as Here we present a review of the different backward and forward selection) and clustering methodologies—model-free and dimension reduction techniques (such as model-based—and their applications in asthma principal components analysis [PCA] and subtyping. We provide an overview of the major factor analysis [FA]). Forward selection studies and discuss the implications and progressively adds variables of greatest approaches used. significance (based on pre-set p values) to the model. Backward selection starts with all WHAT IS CLUSTERING? variables and progressively drops the least significant ones until all the remaining Cluster analysis is a popular unsupervised variables are statistically significant. machine-learning method that seeks to To reduce the large number of variables, the identify similar characteristics in subjects (or majority of studies we reviewed employed variables) and to group them together on that manual extraction based on expert advice. For basis. In selecting groups, the primary aim is to example, Moore et al. [16] manually reduced minimize intra-group variance while the number of variables from 600 to 34 by simultaneously maximizing inter-group excluding variables with missing data and those variance. Clustering ‘classifies’ data by that were either deemed redundant because labelling objects with cluster ‘labels’ or giving information was captured by another variable each object a probability of belonging to a (multicollinearity) or considered not clinically certain cluster. Cluster labels are not known a relevant. Other studies used dimension priori, and are derived solely from the data. This reduction techniques such as PCA and FA, is in contrast to supervised methods such as which reduce data by generating small subsets logistic regression and support vector machines, of generally uncorrelated variables from a large which seek to derive rules for classifying new data set of potentially correlated variables. It is objects based on a set of previously classified useful when we assume that there are objects. underlying latent (unobserved) constructs 22 Pulm Ther (2016) 2:19–41 Fig. 1 Overview of the difference between agglomerative and divisive hierarchical clustering (factors/components) in the data which cannot number of clusters to be
Details
-
File Typepdf
-
Upload Time-
-
Content LanguagesEnglish
-
Upload UserAnonymous/Not logged-in
-
File Pages23 Page
-
File Size-