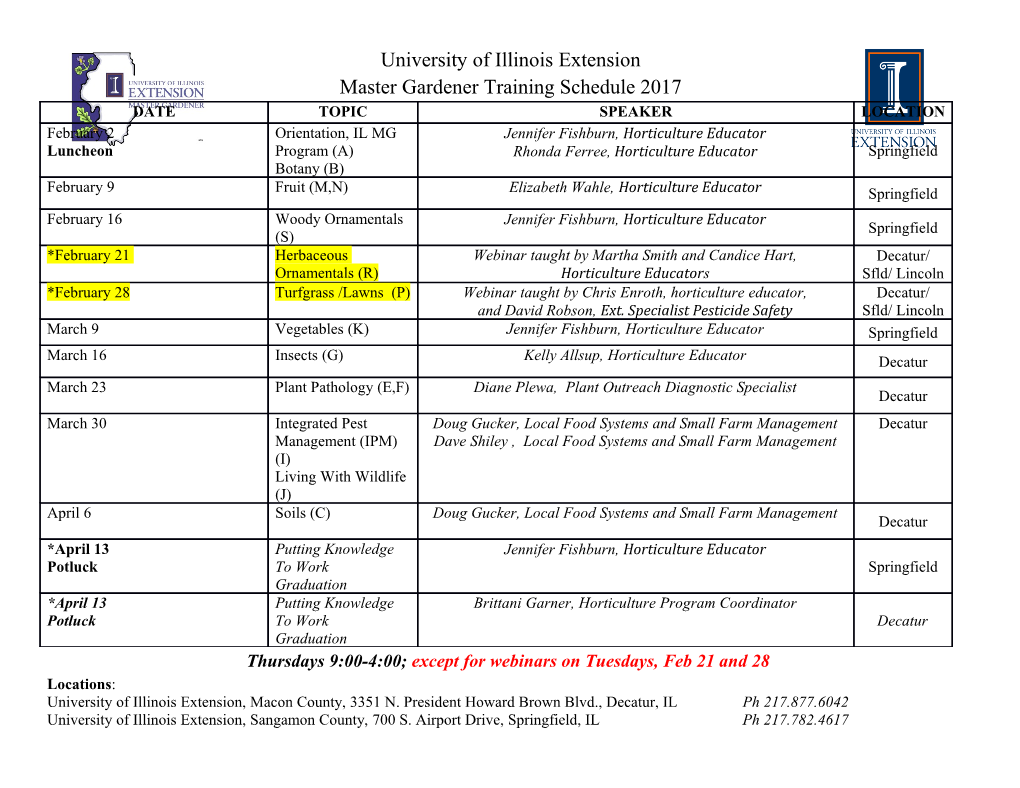
Journal of Surgical Research 126, 149–159 (2005) doi:10.1016/j.jss.2004.12.013 Statistical Power and Estimation of the Number of Required Subjects for a Study Based on the t-Test: A Surgeon’s Primer Edward H. Livingston, M.D.,*,1 and Laura Cassidy, Ph.D.† *Division of Gastrointestinal and Endocrine Surgery, University of Texas Southwestern School of Medicine and the Veterans Administration North Texas Health Care System, Dallas, Texas; and †Graduate School of Public Health, University of Pittsburgh, Pittsburgh, Pennsylvania Submitted for publication October 22, 2004 INTRODUCTION The underlying concepts for calculating the power of a statistical test elude most investigators. Under- standing them helps to know how the various factors Frequently asked these days is how many subjects contributing to statistical power factor into study de- are really needed for a study [1]. Calculations for sign when calculating the required number of subjects answering this question are not intuitively obvious mak- to enter into a study. Most journals and funding agen- ing the determination of adequate sample size a myste- cies now require a justification for the number of sub- rious process usually relegated to the local statistician. jects enrolled into a study and investigators must Most computerized statistical packages include sample present the principals of powers calculations used to size calculators allowing investigators to perform these justify these numbers. For these reasons, knowing assessments on their own. Because computers will pro- how statistical power is determined is essential for vide answers even if they are the wrong ones, it is researchers in the modern era. The number of subjects important for surgical investigators to understand the required for study entry, depends on the following basic tenets of sample size calculation so that they can four concepts: 1) The magnitude of the hypothesized ensure that computerized algorithms are appropriately effect (i.e., how far apart the two sample means are used. expected to differ by); 2) the underlying variability of Previous statistical reviews published in the Journal the outcomes measured (standard deviation); 3) the of Surgical Research summarized concepts necessary .the for the understanding of sample size determination (4 ;(0.05 ؍ ␣ ,.level of significance desired (e.g amount of power desired (typically 0.8). If the sample The basis for these calculations includes knowledge of standard deviations are small or the means are ex- data classification, measures of central tendency, the pected to be very different then smaller numbers of characterization of data sets [2], the fundamental con- subjects are required to ensure avoidance of type 1 cepts of group comparisons and determination of sta- and 2 errors. This review provides the derivation of tistically significant differences [3]. Statistics are all the sample size equation for continuous variables about probabilities, using a sample to make inferences when the statistical analysis will be the Student’s about a population and minimizing the risk of making t-test. We also provide graphical illustrations of how erroneous conclusions regarding that population. As and why these equations are derived. © 2005 Elsevier Inc. All such, there are several potential errors that must be rights reserved. avoided that are summarized in Table 1. Key Words: t-test; normal distribution; Z-statistic; Table 2 illustrates these relationships. Type 1 error study design occurs when a screening test returns a negative result when a patient has a disease. Type 2 error occurs when a screening test returns a positive result when a pa- tient does not have a disease. In designing experi- 1 To whom correspondence and reprint requests should be ad- dressed at Gastrointestinal and Endocrine Surgery, UT Southwest- ments, we attempt to minimize both types of errors but ern Medical Center, 5323 Harry Hines Blvd Room E7-126, Dallas, minimization of type 1 error is most important. The TX 75390-9156. E-mail: [email protected]. consequences of not establishing a diagnosis in a pa- 149 0022-4804/05 $30.00 © 2005 Elsevier Inc. All rights reserved. 150 JOURNAL OF SURGICAL RESEARCH: VOL. 126, NO. 2, JUNE 15, 2005 TABLE 1 Types of Statistical Error Symbol Defintion Alternate definition Implication ␣ Probability of rejecting H0 Probability of an observed difference resulting Type 1 error when it is true from chance alone  Probability of accepting H0 Probability of concluding that no difference Type 2 error when it is false exists when one is present 1Ϫ Probability of rejecting H0 Probability of detecting a statistically Power when it is false significant difference if one exists Note. Errors are the risk of falsely accepting or rejecting a null hypothesis when it is true or false. H0-the null hypothesis. tient with some disease are more significant than 0.9. This means that the statistical test has a 90% falsely believing a person has a disease that, in reality, probability of being correct if it concludes that there is they do not have. a difference between groups when a difference really Statistical writings are replete with double nega- exists. Implicit in this is that if the test finds no differ- tives and confusing verbiage. Surgeons and other biol- ence between the mean values describing the groups ogists may be intimidated by statistical language re- properties, there is a 90% chance that there really is no sulting in a poor understanding of statistical concepts statistically significant difference between the groups. and tests. Particularly striking is the basic tenet of significance or hypothesis testing: The null hypothesis. THE LONG FORGOTTEN EPIC BATTLE BETWEEN It is represented by HO and is defined as the assump- STATISTICAL GIANTS tion that no statistically significant differences exist between important properties describing groups being A bitter, ferocious argument smoldered over the compared. Alternatively, H1, the alternative hypothe- course of decades early in the last century between sis represents the assumption that the measured enti- those responsible for developing the concepts of statis- ties characterizing the two groups are indeed different. tical significance testing and hypothesis evaluation [4]. Confusion results from the application of double neg- The story starts with Karl Pearson (1857–1936) con- atives. We seek to prove that the null hypothesis, i.e., sidered being one of the founders of statistics. He was that no statistically significant differences exist, is responsible for the Pearson correlation coefficient, the false. It is easier to state that we are seeking to find 2 test, linear regression and other fundamental con- differences between groups when they exist. That view cepts of statistics. He founded and headed the Depart- is more intuitively obvious and easier to reconcile. ment of Statistics at University College in London. However, it is important to recognize the null hypoth- That department was split into two to accommodate esis’s meaning given that it is ubiquitous in statistical two other giants in statistics, Egon Pearson (1895– writings. 1980), Karl’s son and Ron Fisher (1890–1962). Fisher When statistically significant differences are calcu- was most prolific, publishing on average one paper lated, an arbitrary ␣ value is set. Statistical convention sets this value at 0.05. In other words, there is a less TABLE 2 than 5% probability that observed differences between groups occur because of chance alone rather than a Further Examples of Error Types as they Pertain to true difference between the groups. The ␣ value estab- Diagnostic Tests lishes the risk of type 1 error, or the risk of falsely Actual situation (disease) concluding that differences between groups exist when in fact none do. Positive Negative Type 2, or  error, is the possibility of concluding that no statistically significant difference exists when, Screening test in fact, the groups being compared really are different. Positive Correct Type 2 error The statistical power of a test is defined by 1Ϫ or the Negative Type 1 error Correct probability that when a test concludes that there is a Note. Type 1 errors occur when a diagnostic test is negative but a difference between the groups being compared that the patient actually has a disease. This is considered to be more serious test result is correct. For example, if the  is 0.1 then than type 2 errors being that establishing the diagnosis of a medical there is a 10% chance that two groups really were problem may be missed. Type 2 error occur when a test is falsely positive, i.e., that a test is positive when the patient does not have a different when a statistical test suggests that the mean disease. Under these circumstances, the positive test will result in values for the properties describing the groups were further evaluation that will, hopefully, reveal that the disease was not different. 1Ϫ ϭ 0.9 such that the tests power is not actually present. LIVINGSTON AND CASSIDY: STATISTICAL POWER 151 every 2 months and was responsible for many concepts decisions regarding industrial processes. Fisher’s signif- guiding research today. Most importantly, he devel- icance testing was more theoretical and less practical oped the idea of significance testing and P values. and ignored the cost of performing an analysis. In this Fisher’s analytic approach was inductive being that he regard Fisher’s system was considered more applicable believed that experimental observations were repre- to scientific research than to industrial applications. sentative samples of a larger universe or population of Neyman and Pearson’s system was deductive, in con- observations for features that characterize groups be- trast to Fisher’s inductive analysis, in the sense that ing compared experimentally. Experimental data were data were produced under predefined circumstances considered as a sample from a larger population with with predetermined constraints for analyzing them. ␣ various assumptions being made regarding how repre- They introduced the concept of , the level of signifi- cance associated with type 1 error.
Details
-
File Typepdf
-
Upload Time-
-
Content LanguagesEnglish
-
Upload UserAnonymous/Not logged-in
-
File Pages11 Page
-
File Size-