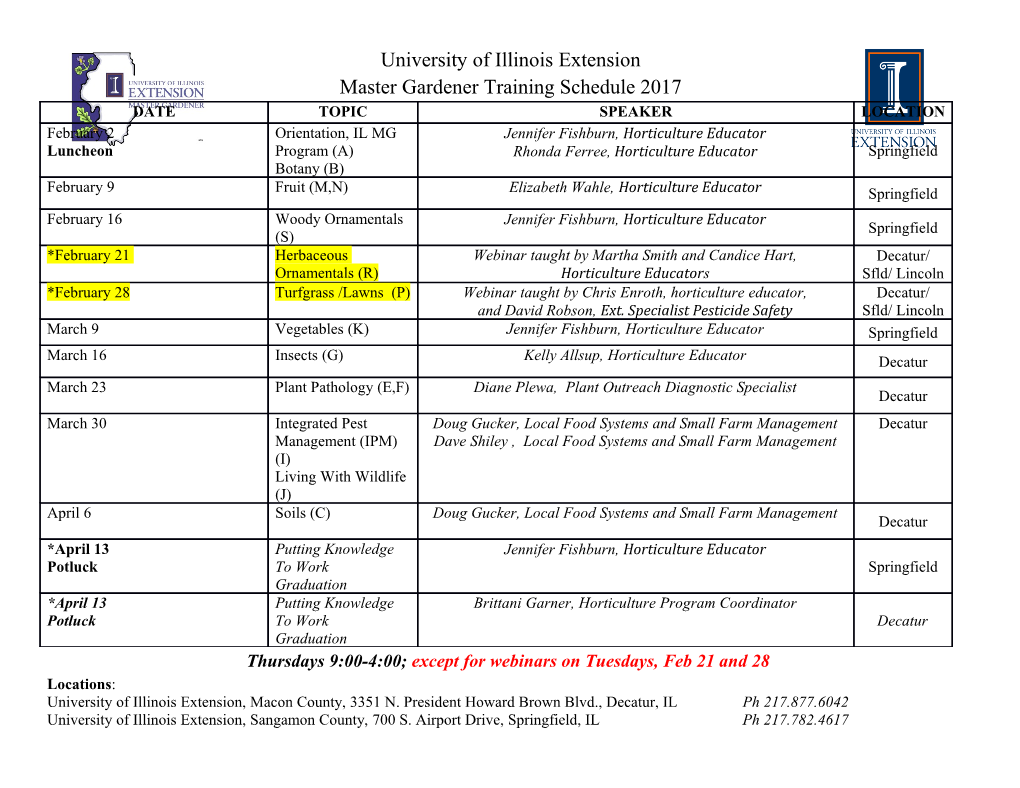
Introspective Perception: Learning to Predict Failures in Vision Systems Shreyansh Daftry, Sam Zeng, J. Andrew Bagnell and Martial Hebert Abstract— As robots aspire for long-term autonomous oper- ations in complex dynamic environments, the ability to reliably take mission-critical decisions in ambiguous situations becomes critical. This motivates the need to build systems that have I think I might fail. situational awareness to assess how qualified they are at that moment to make a decision. We call this self-evaluating capability as introspection. In this paper, we take a small step in this direction and propose a generic framework for introspective behavior in perception systems. Our goal is to learn a model to reliably predict failures in a given system, with respect to a task, directly from input sensor data. We present this in the context of vision-based autonomous MAV flight in outdoor natural environments, and show that it effectively handles uncertain situations. I. INTRODUCTION Perception is often the weak link in robotics systems. Fig. 1. Robot Introspection: Having a self-evaluating facility to know when While considerable progress has been made in the computer it doesn’t know will augment a robot’s ability to take reliable mission-critical vision community, the reliability of vision modules is often decisions in ambiguous situations. insufficient for long-term autonomous operation. Of course this is not surprising for two reasons. First, while vision algorithms are being systematically improved in particular hardcode ad-hoc test to reject such inputs, it is impossible to by using common benchmark datasets such as KITTI [1] anticipate all of the conditions encountered in a real world or PASCAL [2], their accuracy on these datasets does not environment. necessarily translate to the real-world situations encountered This presents a challenge as the real cost of failure can be by robotics systems. We advocate that for robotics applica- significant. For example, an autonomous drone that fails to tions, evaluating perceptions systems based on these stan- detect an obstacle can suffer disastrous consequences. They dard metrics is desirable but insufficient to comprehensively can cause catastrophic physical damage to the robot and its characterize system performance. The dimension missed is surroundings. We thus believe that it is essential to identify the robot’s ability to take action in ambiguous situations. as early as possible, i.e., based on sensor input, situation Second, even if a vision algorithm had perfect performance in which a trustable decision cannot be reached because of on these off-line datasets, it is bound to encounter inputs that degraded performance of the perception system. Crucially, it cannot handle. During long-term autonomous operations, and central to this paper, this requires the perception system robotics systems have to contend with vast amounts of to have the ability to evaluate its input based on a learned continually evolving, unstructured sensor data from which performance model of its internal algorithm. We call this information needs to be extracted. In particular, the problem capability introspection1, a precursor to action selection. aggravates for perception systems where the problems are In this paper, we explore the possibility of building often ambiguous, and algorithms are designed to work under introspective perception systems that can reliably predict strong assumptions that may not be valid in real-world their own failures and take remedial actions. We argue that scenario. Several factors - degraded image quality; uneven while minimizing failures has been the primary focus of the illumination; poor texture - can affect the quality of visual community, embracing and effectively dealing with failures input, and consequentially affect the robots’ action, possibly has been neglected. We propose a generic framework for resulting in catastrophic failure. For example, over- or under- introspective behavior in perception systems that enables a exposed images, images with massive motion blur would robot to know when it doesn’t know by measuring as to how confound a vision-based guidance system for a mobile robot. qualified the state of the system it is to make a decision. This While it is possible to anticipate some of these conditions and helps the system to mitigate the consequences of its failures by learning to predict the possibility of one in advance and S. Daftry, S. Zeng, J. A. Bagnell and M. Hebert are all members of take preventive measures. the Robotics Institute, Carnegie Mellon University, Pittsburgh, PA, 15213, USA. This work was supported by the ONR MURI grant for ‘Provably- Stable Vision-based Control of High-speed Flights through Forest and Urban 1Introspection. The act or process of self-awareness; contemplation of Environments’. Email: fdaftry, slzeng, dbagnell, [email protected] one’s own thoughts or feelings, and in the case of a robot, its current state. We explore these ideas in the context of autonomous itself in order to assess the system’s reliability. The main flight, where mission-critical decisions equate to safety- motivation for such an approach is that while the perception critical consequences [3]. Consider the task of autonomously system may not be able to reliably predict the correct output navigating a dense cluttered environment with a MAV using for a certain input, predicting the difficulty or ambiguity of monocular vision. This requires the MAV to successfully the input may still be feasible. Moreover, this is applicable to estimate a local map of its surrounding in real-time, at any vision system because the reliability estimate is agnostic minimum the depth of objects relative to the drone, and to the actual algorithm itself and based on the input alone. based on this map to select an optical trajectory to reach its In a similar spirit, Jammalamadaka et al. [16] recently destination, while avoiding any obstacle. It is crucial to note introduced evaluator algorithms to predict failures for human that this kind of a system, like most other real-world robotics pose estimators. They used features specific to this applica- applications, involves modular pipelines, where the output tion; Welinder et al. [17] and Aghazadeh and Carslsson [18] of one module is fed into another as input. In such cases, analyzing statistics of the training and test data to predict decisions based on overconfident output from one subsystem, the global performance of a classifier on a corpus of test in this case a perception module, will lead to catastrophic instances. Another line of research involves the domain of failure. In contrast, anticipation of a possible failure based on ‘rejection’ [19]. This is related to systems that identify an previous experience allows for remedial action to be taken in instance to belong to ’none of the above’ class, as compared the subsequent layers. Providing this more relevant prediction to the one’s during training, and thereby refuse to make a is the introspective quality that we seek. decision on that instance . Zhang et al. [20] learn a SVM The rest of the paper is organized as follows: In Section II, model to predict failures from visual input. Bansal et al. [21] we review the related work on introspection. In Section III, learn a semantic characterization of failure modes. we introduce our generic introspective perception framework. The biometrics community has used image quality mea- Section IV outlines our autonomous monocular navigation sures as predictors for matching performance [22]. The approach. Quantitative, qualitative and system performance concept of input ‘quality’, as used here, is implicitly tied evaluation with respect to related work is delineated in to the underlying perception system. Our work follows the Section V. same philosophy. Such methods, like ours, only analyze the input instance (e.g., fingerprint scan) to assess the expected II. RELATED WORK quality of performance (e.g. fingerprint match). Our method Our work addresses an issue that has received attention is complementary to all the previous approaches: it uses in various communities. The concept of introspection as generic image appearance features, provides an instance- introduced here can be closely related to active learning and specific reliability measure, and the domain we consider is incremental learning [4], [5], [6], where uncertainty estimates not only concerned with unfamiliar instances that arise from and model selection steps are used to guide data acquisition discrepancies between the training and test data distributions and selection. KWIK ‘Knows What It Knows’ frameworks but also addresses familiar but difficult instances. In addition, [7] allow for active exploration which is beneficial in re- we show the effectiveness of such predictions in a mission- inforcement learning problems. Further, reliably estimating critical decision making scenario such as robot navigation. the confidence of classifiers has received a lot of attention We advocate that a similar level of self-evaluation should in the pattern recognition community [8]. Applications such be part of all perception systems as they are involved in a as spam-filtering [9], natural language processing [10] and variety of real-world applications. even computer vision [11] have used these ideas. In robotics, the concept of introspection was first intro- duced by Morris et al. [12] and has recently been adopted III. INTROSPECTIVE PERCEPTION more specifically for perception systems by Grimmett et al. Introspective robot perception is the ability to predict and [13], [14] and Triebel et al. [15]. They explore the introspec- respond to unreliable operation of the perception module. In tive capacity of a classifier using a realistic assessment of the this section, we describe a generic framework for introspec- predictive variance. This is in contrast to other commonly tive robot perception, which we illustrate in the context of used classification frameworks which often only rely on vision-based control of MAVs in the next section. a one-shot (ML or MAP) solution. It is shown that the accountability of this introspection capacity is better for real A.
Details
-
File Typepdf
-
Upload Time-
-
Content LanguagesEnglish
-
Upload UserAnonymous/Not logged-in
-
File Pages8 Page
-
File Size-