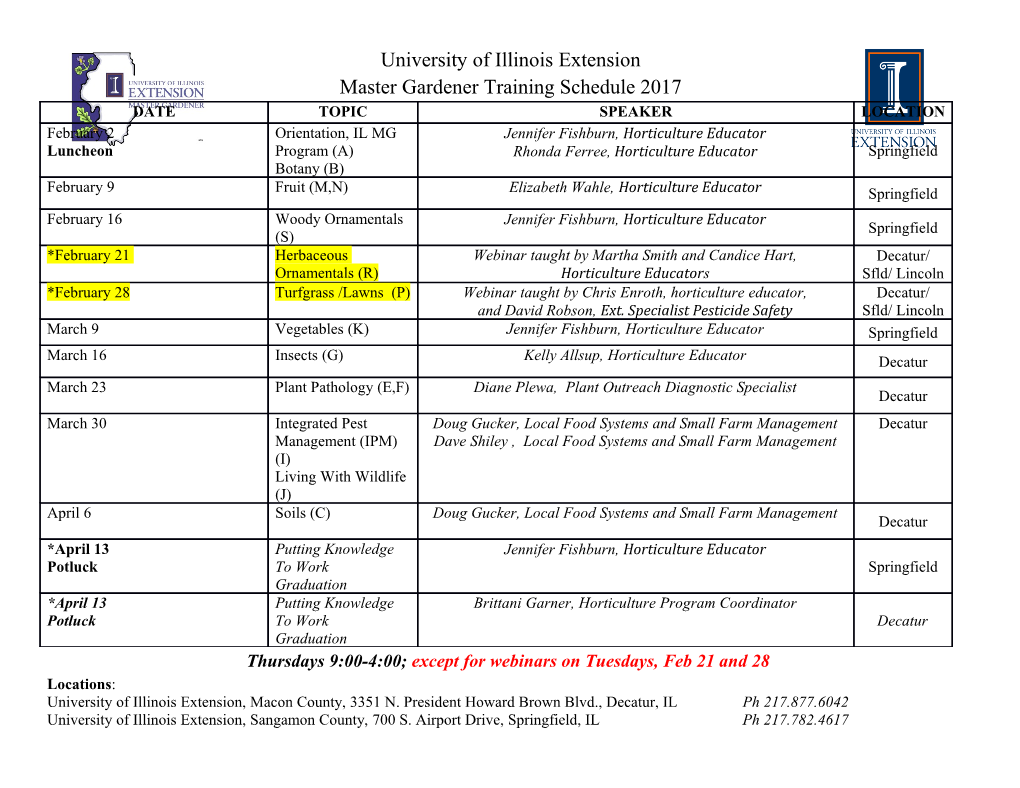
Resource Optimal-Transport Analysis of Single-Cell Gene Expression Identifies Developmental Trajectories in Reprogramming Graphical Abstract Authors Geoffrey Schiebinger, Jian Shu, Marcin Tabaka, ..., Rudolf Jaenisch, Aviv Regev, Eric S. Lander Correspondence [email protected] (J.S.), [email protected] (A.R.), [email protected] (E.S.L.) In Brief Application of a new analytical approach to examine developmental trajectories of single cells offers insight into how paracrine interactions shape reprogramming. Highlights d Optimal transport analysis recovers trajectories from 315,000 scRNA-seq profiles d Induced pluripotent stem cell reprogramming produces diverse developmental programs d Regulatory analysis identifies a series of TFs predictive of specific cell fates d Transcription factor Obox6 and cytokine GDF9 increase reprogramming efficiency Schiebinger et al., 2019, Cell 176, 928–943 February 7, 2019 ª 2019 Elsevier Inc. https://doi.org/10.1016/j.cell.2019.01.006 Resource Optimal-Transport Analysis of Single-Cell Gene Expression Identifies Developmental Trajectories in Reprogramming Geoffrey Schiebinger,1,11,16 Jian Shu,1,2,16,* Marcin Tabaka,1,16 Brian Cleary,1,3,16 Vidya Subramanian,1 Aryeh Solomon,1,17 Joshua Gould,1 Siyan Liu,1,15 Stacie Lin,1,6 Peter Berube,1 Lia Lee,1 Jenny Chen,1,4 Justin Brumbaugh,5,7,8,9,10 Philippe Rigollet,11,12 Konrad Hochedlinger,7,8,9,13 Rudolf Jaenisch,2,3 Aviv Regev,1,6,13,* and Eric S. Lander1,6,14,18,* 1Klarman Cell Observatory, Broad Institute of MIT and Harvard, Cambridge, MA 02142, USA 2Whitehead Institute for Biomedical Research, Cambridge, MA 02142, USA 3Computational and Systems Biology Program, MIT, Cambridge, MA 02142, USA 4Harvard-MIT Division of Health Sciences and Technology, Cambridge, MA 02139, USA 5Cancer Center, Massachusetts General Hospital, Boston, MA 02114, USA 6Department of Biology, Massachusetts Institute of Technology, Cambridge, MA 02139, USA 7Department of Molecular Biology, Center for Regenerative Medicine and Cancer Center, Massachusetts General Hospital, Boston, MA 02114, USA 8Department of Stem Cell and Regenerative Biology, Harvard University, Cambridge, MA 02138, USA 9Harvard Stem Cell Institute, Cambridge, MA 02138, USA 10Harvard Medical School, Boston, MA 02115, USA 11MIT Center for Statistics, Massachusetts Institute of Technology, Cambridge, MA 02139, USA 12Department of Mathematics, Massachusetts Institute of Technology, Cambridge, MA 02139, USA 13Howard Hughes Medical Institute, Chevy Chase, MD 20815, USA 14Department of Systems Biology Harvard Medical School, Boston, MA 02125, USA 15Biochemistry Program, Wellesley College, Wellesley, MA 02481, USA 16These authors contributed equally 17Present address: Weizmann Institute of Science, Rehovot, Israel 18Lead Contact *Correspondence: [email protected] (J.S.), [email protected] (A.R.), [email protected] (E.S.L.) https://doi.org/10.1016/j.cell.2019.01.006 SUMMARY ramming and provides a framework applicable to diverse temporal processes in biology. Understanding the molecular programs that guide differentiation during development is a major chal- lenge. Here, we introduce Waddington-OT, an INTRODUCTION approach for studying developmental time courses to infer ancestor-descendant fates and model Waddington introduced two metaphors that shaped biological thinking about cellular differentiation: first, trains moving along the regulatory programs that underlie them. We branching railroad tracks and, later, marbles rolling through a apply the method to reconstruct the landscape developmental landscape (Waddington, 1936, 1957). Studying of reprogramming from 315,000 single-cell RNA the actual landscapes, fates, and trajectories associated with sequencing (scRNA-seq) profiles, collected at cellular differentiation and de-differentiation—in development, half-day intervals across 18 days. The results physiological responses, and reprogramming—requires us to reveal a wider range of developmental programs answer questions such as: What classes of cells are present at than previously characterized. Cells gradually each stage? What was their origin at earlier stages? What are adopt either a terminal stromal state or a mesen- their likely fates at later stages? What regulatory programs con- chymal-to-epithelial transition state. The latter trol their dynamics? gives rise to populations related to pluripotent, Approaches based on bulk analysis of cell populations are not extra-embryonic, and neural cells, with each well suited to address these questions, because they do not pro- vide general solutions to two challenges: discovering cell classes harboring multiple finer subpopulations. The anal- in a population and tracing the development of each class. ysis predicts transcription factors and paracrine The first challenge has been largely solved by the advent of signals that affect fates and experiments vali- single-cell RNA sequencing (scRNA-seq) (Tanay and Regev, date that the TF Obox6 and the cytokine GDF9 2017). The second remains a work-in-progress. Because enhance reprogramming efficiency. Our approach scRNA-seq destroys cells in the course of recording their pro- sheds light on the process and outcome of reprog- files, one cannot follow expression of the same cell and its direct 928 Cell 176, 928–943, February 7, 2019 ª 2019 Elsevier Inc. descendants across time. While various approaches can record scape of differentiation trajectories and intermediate states information about cell lineage, they currently provide only very that give rise to these diverse fates, we describe a gradual tran- limited information about a cell’s state at earlier time points (Kes- sition to either stroma-like cells or a mesenchymal-to-epithelial ter and van Oudenaarden, 2018). transition (MET) state. Trajectories emerge from the MET state Comprehensive studies of cell trajectories thus rely heavily on to iPSCs, extraembryonic cells, and neural cells. Based on the computational approaches to connect discrete ‘‘snapshots’’ into trajectories, we infer TFs predictive of various fates and suggest continuous ‘‘movies.’’ Pioneering work to infer trajectories (Sae- paracrine interactions between the stromal cells and other cell lens et al., 2018) has shed light on various biological systems, types. We experimentally showed that two top predictions including whole-organism development (Farrell et al., 2018; indeed enhance reprogramming efficiency. Wagner et al., 2018), but many important challenges remain. First, with few exceptions, most methods do not explicitly RESULTS leverage temporal information (Table S6). Historically, most were designed to extract information about stationary pro- Reconstruction of Probabilistic Trajectories by Optimal cesses, such as adult stem cell differentiation, in which all stages Transport exist simultaneously. However, time courses are becoming Our goal is to learn the relationship between ancestor cells at one commonplace. Second, many methods model trajectories in time point and descendant cells at another time point: given that terms of graph theory, which imposes strong constraints on a cell has a specific expression profile at one time point, where the model, such as one-dimensional trajectories (‘‘edges’’) and will its descendants likely be at a later time point and where zero-dimensional branch points (‘‘nodes’’). Thus, gradual diver- are its likely ancestors at an earlier time point? We model a gence of fates is not captured well by these models. Third, few differentiating population of cells as a time-varying probability methods account for cellular growth and death during develop- distribution (i.e., stochastic process) on a high-dimensional ment (Table S6). expression space. By sampling this probability distribution Pt Here, we describe a conceptual framework, implemented in a at various time points t, we wish to infer how the differentiation method called Waddington-OT, that aims to capture the notion process evolves over time (Figure 1A). From a large number of that cells at any time are drawn from a probability distribution cells at a given time point (Figure 1B), we can approximate the in gene-expression space, and each cell has a distribution of distribution at that time point, but, because different cells are both probable origins and probable fates (Figure 1). It uses sampled independently at different time points, we lose the joint scRNA-seq data collected across a time course to infer how distribution of expression between pairs of time points, called these probability distributions evolve over time, by using the temporal coupling. Absent any constraint on cellular transitions, mathematical approach of optimal transport (OT). we cannot infer the temporal coupling, but if we assume that We apply this framework to the challenge of understanding cells move short distances over short time periods, then we cellular reprogramming following transient overexpression of a can infer the temporal coupling by using the mathematical tech- set of transcription factors (TFs) (Takahashi and Yamanaka, nique of optimal transport (Figure 1A; Methods S1). 2016). We aim to address questions such as: What classes of Optimal transport was originally developed to redistribute cells arise in reprogramming? What are the developmental paths earth for the purpose of building fortifications with minimal that lead to reprogramming and to any alternative fates? Which work (Monge, 1781) and soon applied by Napoleon in Egypt. cell intrinsic factors and cell-cell interactions drive progress
Details
-
File Typepdf
-
Upload Time-
-
Content LanguagesEnglish
-
Upload UserAnonymous/Not logged-in
-
File Pages39 Page
-
File Size-