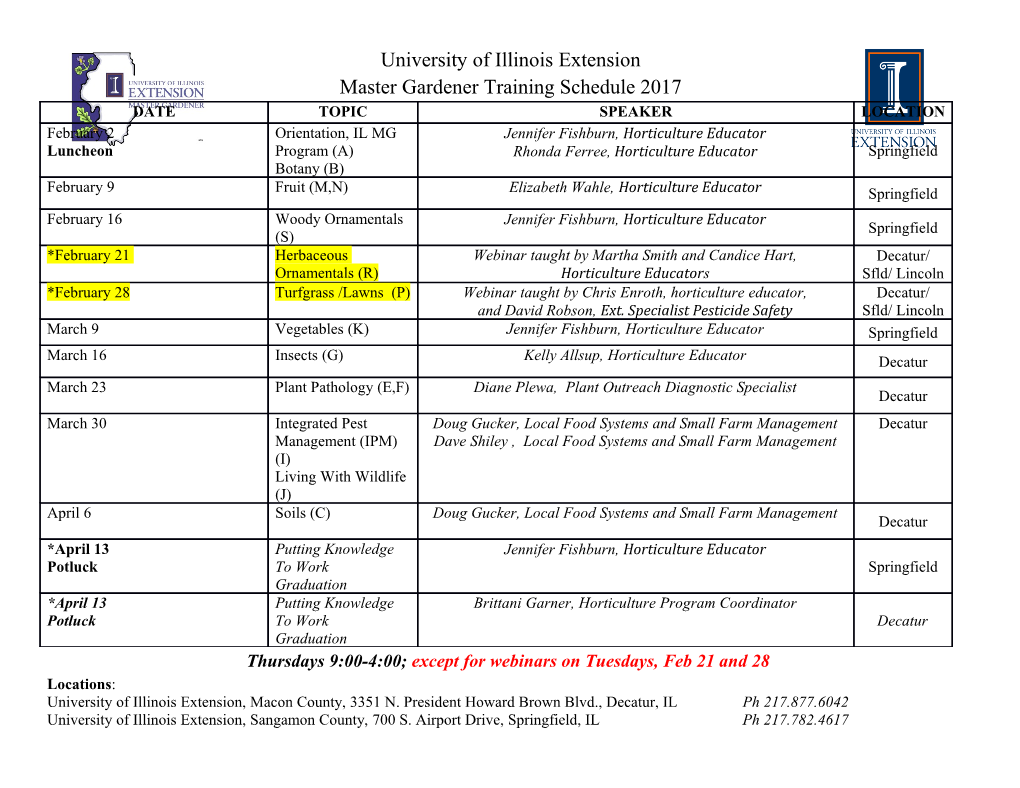
Improving the Prediction of Readmissions Amongst Medicare Patients in a California Hospital Nhan Huynh, Dylan Robbins-Kelley, Holly Fallah Faculty Advisors: Ian Duncan, Janet Duncan, Wade Herndon Dept. of Statistics and Applied Probability, University of California Santa Barbara Introduction Methodology Results Purpose and Motivation Logistic Regression Models Centers for Medicare and Medicaid Services A regression model where the data set has a Three models were created: (CMS) reduced Medicare payments for binary response or a multinomial response . LACE model hospitals with excess readmissions (within 30 and several predictors . General Model days of discharge) for following health We are interested in predicting the . Age 65+ model with CMS penalty conditions: probability a patient is readmitted to the conditions . Heart Attack, Heart Failure, Pneumonia, hospitals within 30 days after discharge Hip/Knee Replacement, Chronic based on characteristics such as: Obstructive Pulmonary Disease. age, gender, length of stay during Readmissions can lead to longer stays, and admission, diagnoses, admission from put patients at additional risk of hospital- emergency department, number of acquired infections and complications. emergency visits, etc… Development of LACE Logistic regression links the binary outcomes Table to compare predicted and actual re- Currently the LACE index is a widely used of readmission status with a combination of admissions using the age 65+ model: readmission model in the United States, due the linear predictors. Let p=probability the patient is readmitted to its simplicity and moderate predictive power. within 30 days after discharge LACE scores every patient on the risk of Let b0=intercept readmission upon discharge based on the Let bp=coefficient of variable following parameters: Let Xp=variable . Length of stay . Acuity of admission . Comorbidity . Emergency department visits in the previous 6 months. Validation Conclusions LACE scores range from 0-19 Logistic regression is built on 80% of the data Sensitivity values in logistic regression models . Low Risk 0-4 set. The remaining 20% of the data set is used are higher than the value in the LACE model. Moderate Risk 5-9 for internal validation. Specificity is higher in LACE, however the . High Risk 10-19 A confusion matrix was examined to slightly lower specificity values in the compare the sensitivity (true positive rate), regression models are worth the specificity (false negative rate), positive compensation to gain sensitivity. Data Summary predicted value, and c-statistic. This indicates an improvement in predictive Data aquired from single hospital consisting A new cutoff value was created to power of regression models compared to of 76,538 patients in five years compromise the tradeoff between the true the LACE model. positive rate and false negative rate. When comparing both regression models, the general model is preferred because of its higher sensitivity, specificity, and AUC vales compares to the age and penalty specific model. References 1. Van Walraven C, Dhalla IA, Bell C, et al. Derivation and Validation of an Index to Predict Early Death or Unplanned Readmission After Discharge From Hospital to the Community. CMAJ 2010; 182: 551-557. 2. Spiva L, Hand M, VanBrackle L, et al. Validation of a Predictive Model to Identify Patients at High Risk for Hospital Readmission. JHQ 2014; 00(0): 1-7. 3. S, Farooq F, van Esbroeck A, et al. Predicting Readmission Risk with Institution-Specific Prediction Models. Artificial Intelligence in Medicine 2015; 65(2): 89-96. 4. Billings J, Dixon J, Mijanovich T, et al. Case finding for patients at risk of readmission to hospital: development of algorithm to identify high risk patients. BMJ 2006, doi:10.1136/bmj.38870.657917.AE. .
Details
-
File Typepdf
-
Upload Time-
-
Content LanguagesEnglish
-
Upload UserAnonymous/Not logged-in
-
File Pages1 Page
-
File Size-