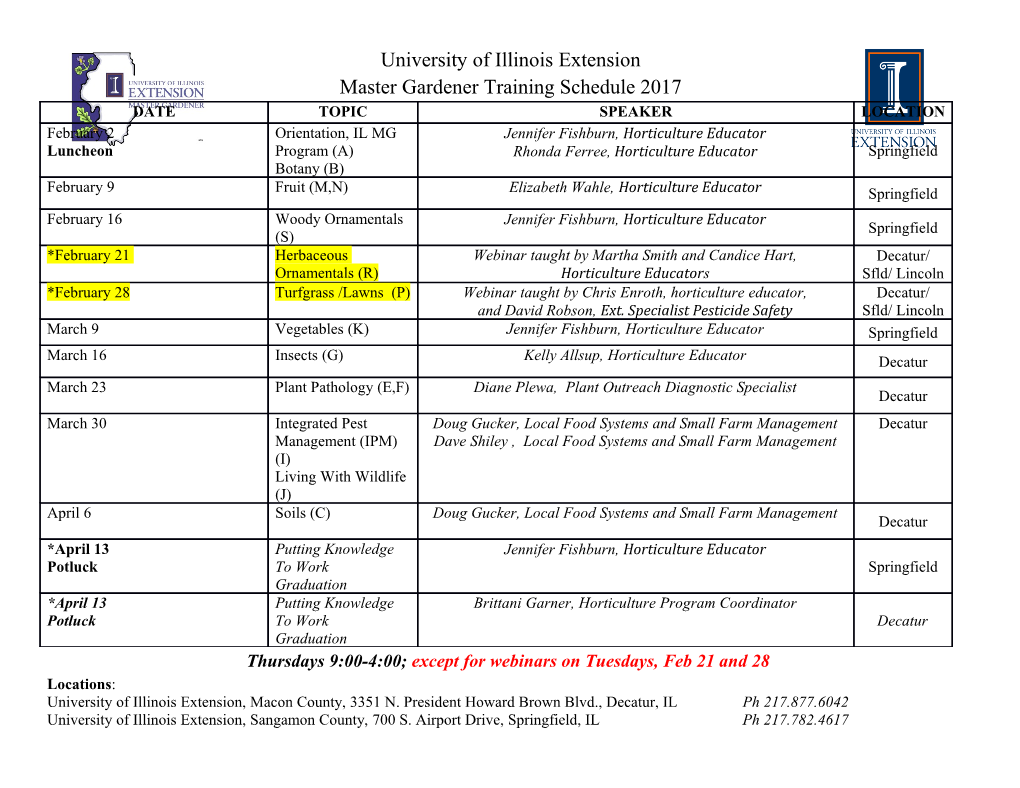
Chapter 8: Split-Plot Designs Split-plot designs were originally developed by Fisher (1925,Statistical Methods for Research Workers) for use in agricultural experiments where factors are differentiated with respect to the ease with which they can be changed from experimental run to experimental run. This may be due to the fact that a particular treatment is expensive or time-consuming to change or it may be due to the fact that the experiment is to be run in a large batches and batches can be subdivided later for additional treatments. A split-plot design may be considered as a special case of the two-factor randomized design where one wants to obtain more precise information about one factor and also about the interaction between the two factors. The second factor being of secondary importance to the experimenter. Example 1 Consider a study of the effects of four irrigation methods (A1, A2, A3, and A4) and three fertilizers (B1, B2, and B3). Two replicates are planned to carried out for each of the 12 combinations. A completely randomized design will randomly assign a combination of irrigation and fertilization methods to one of the 24 fields. This may be easy for fertilization but it is hard for irrigation where sprinklers are arranged in a certain way. What is usually done is to use just eight plots of land, chosen so that each can be irrigated at a set level without affecting the irrigation of the others. These large plots are called the whole plots. Randomly assign irrigation levels to each whole plot so that each irrigation level is assigned to exactly two plots. Irrigation is called the whole-plot factor. Divide each whole plot into three plots. Each plot is called a subplot. Within each whole plot, randomly assign the three fertilizers to the three subplots. Fertilizer is called the subplot factor. Note that, in this split-plot design, the first randomization assigns the four irrigation types to eight fields (whole plots), then, the second randomization is conducted to assign the three fertilizers to three subplots within each field. 1 whole plot factor A3 A1 A2 A4 A2 A1 A4 A3 subplot factor subplot B3 B2 B1 B1 B2 B3 B1 B2 B2 B1 B2 B3 B1 B2 B3 B3 B1 B3 B3 B2 B3 B1 B2 B1 Example 2 Box et al. (2005, Statistics for experimenters: Design, inovation, and Discovery, 2nd) described a prototypical split-plot experiment with one easy- to-change factor and one hard-to-change factor. The experiment was designed to study the corrosion resistance of steel bars treated with four coatings, C1, o o o C2, C3, and C4, at three furnance temperatures, 360 C, 370 C, and 380 C. Furnance temperature is the hard-to-change factor because of the time it takes to reset the furnance and reach new equilibrium temperature. Once the equilibrium temperature is reached, four steel bars with randomly assigned coatings C1, C2, C3, and C4 are randomly positioned in the furnance and heated. Here are the data. 2 Temperature Coatings Whole-plot (oC) (randomized order) 1 360 C2 C3 C1 C4 73 83 67 89 2 370 C1 C3 C4 C2 65 87 86 91 3 380 C3 C1 C2 C4 147 155 127 212 4 380 C4 C3 C2 C1 153 90 100 108 5 370 C4 C1 C3 C2 150 140 121 142 6 360 C1 C4 C2 C3 33 54 8 46 Split-Plot Experiments with CRD in Whole Plots (CRSP) Split-plot designs have three main characteristics: 1. The levels of all the factors are not randomly determined and reset for each experimental run. Usually the experimenter holds a factor at a particular setting and then run all the combinations of the other factors. 2. The size of the experimental unit is not the same for all experimental factors. Usually the experimenter applies one factor to a larger unit or group of units involving combinations of the other factors. 3. There is a restriction on the random assignment of the treatment combina- tions to the experimental units. Usually, there is something that prevents assigning the treatments to the units completely randomly. Example 3 Recipes for chocolate and orange cookies include exactly the same ingredients up to the point where the syrup was added to the batch. However, after the cookies were baked, the chocolate cookies had an appealing round and plump appearance, while the orange cookies spread during the baking process and became thin, flat, and unappealing. A factorial experiment was devised to determine if there was a way to change the process of making the orange cookies that would reduce the spreading during baking. The factors that were chosen to be varied were A: the amount of shortening in the dough batch (80% of what the recipe called for or 100%), B: the baking temperature (below, at or above the temperature called for by the recipe), and C: The temperature of the cookies sheet upon which the cookies were placed to be baked (hot out of the oven or cooled to room temperature). A response that could quantify the objective of the experiment was the diameter of the baked cookies. A completely randomized design for this 3 22 factorial experiment with- out replicates needs 12 experimental units. The× experimental units is a batch of cookie doughs. However, the cooking-making process consists of two steps: 3 mixing cookie dough batch and baking cookies. The amount of shortening was a hard-to-change factor because each time it was changed it required making a new batch of cookie dough, while the baking temperature and tray temperature were relative easier to vary. Therefore, an alternative way of designing the experiments would be to follow a two-step plan. First, plan to make four batches of cookie dough and randomly assign two batches to use 80% of the recipe recommended amount of shorten and two batches to recieve the full amount of shortening recommended by the recipe. This represents a completely randomized design in one factor and the experimental unit is a batch of cookie dough. This first step is called the whole plot design and the whole-plot factor is A: the amount of shortening. Next, bake six trays of cookies from each batch of dough and completely ran- domized the six combinations of bake temperature and tray temperature to the six trays of cookies within each batch. This is a randomized block 3 2 facto- rial within each batch of cookie dough and is called the sub-plot design× . The sub-plot block is the batch of cookies, the sub-plot experimental unit is a tray of cookies and the sub-plot factors are B: the bake temperature and C: the tray temperature. The combination of the whole-plot and sub-plot design is called a split-plot design with CRD in the whole plots or CRSP. By designing the experiment in this way only four batches of cookies are required in tead of 12 and there are replicate whole-plot and sub-plot experimental units from which variances of two experimental errors can be estimated. It will be showns that there is less power for testing the shortening effect than there would be with the 12 batch completely randomized designed, but there is actually more power for detecting the baking temperature, tray temperature, and interaction due to the blocking by batch. Here are more examples from Kowalski, S.M. and Potcner, K.J. (2003, How to recognize a split-plot experiment, Quality Progress, 36(11), 60-66). In the printing press process, blanket type is the hard-to-change factor. 4 The Printing Press In the experiment of water resistance property of wood, pretreatment type is the hard-to-change factor. 5 Water Resistance Property of Wood In the baking process of plastic strength, oven temperature is the hard-to-change factor. 6 Strength of Plastic in Baking Process Chapter 10. Response Surface Methodology Response surface methodology (RSM) is a collection of statistical and mathe- matical techniques useful for developing, improving, and optimizing processes. It is also important applications in the design, development, and formulation of new products, as well as in the improvement of existing product designs. Response surface methodology was developed by Box and Wilson (1951, On the experimental attainment of optimum conditions, JRSS-B, 13, 1-45) to aid the improvement of manufacturing processes in the chemical industry. The purpose was to optimize chemical reactions to obtain, for example, high yield and purity at low cost. This was accomplished through the use of sequential experimentation involving factors such as temperature, pressure, duration of reaction, and proportion of reactants. The same methodology can be used to model or optimize any response that is affected by the levels of one or more quantitative factors. Response surface methods generally refer to a complete package of statistical design and analysis tools that are used for the following steps. 1. Design and collection of data to fit an equation to approximate the rela- tionship between factors and responses 7 2. Regression analysis to fit a model to describe the data 3. Examination of the fitted relationship through graphical and numerical techniques. The most extensive applications of RSM are in the industrial world, par- ticularly in situations where several input variables potentially influence some performance measure or quality characteristic of the product or process. The general scenario is as follows. The response is a quantitative continuous vari- able (e.g., yield, purity, cost), and the mean response is a smooth but unknown function of the levels of p factors (e.g., temperature, pressure), and the levels are real-valued and accurately controllable.
Details
-
File Typepdf
-
Upload Time-
-
Content LanguagesEnglish
-
Upload UserAnonymous/Not logged-in
-
File Pages40 Page
-
File Size-