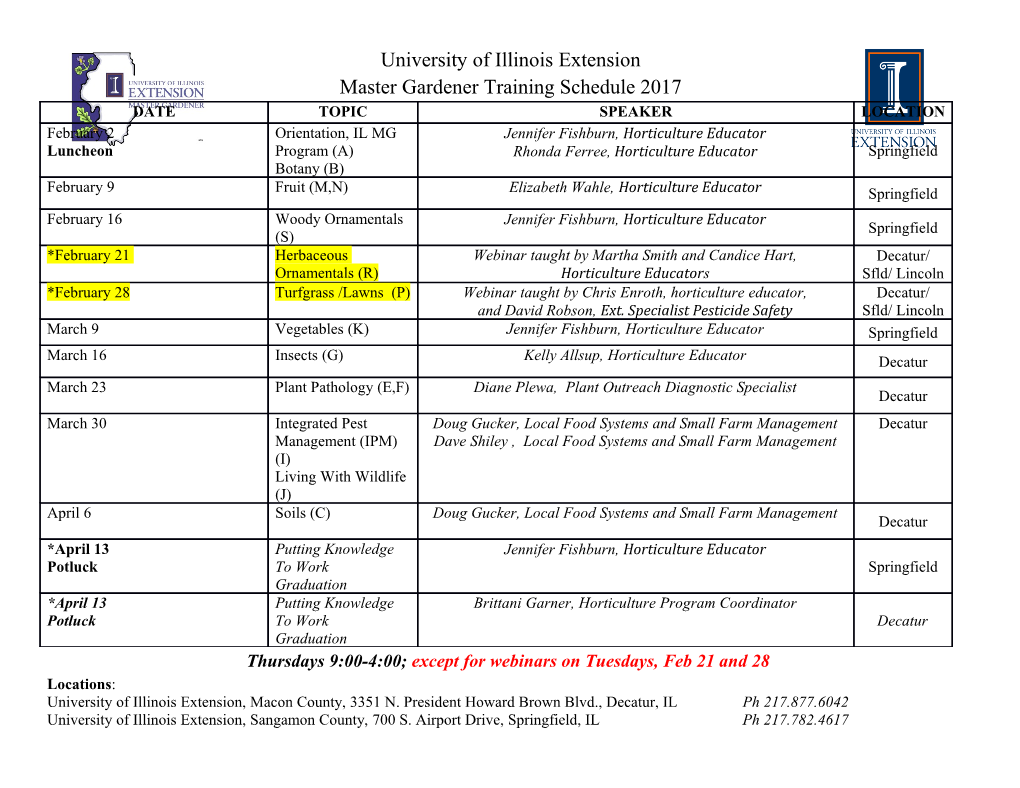
Climatology of Severe Convective Wind Gusts in Australia Alessio Cosmo Spassiani M.Sc., B.Sc. (Hons.) A thesis submitted for the degree of Doctor of Philosophy at The University of Queensland in 2020 School of Civil Engineering Abstract Severe convective wind storms are responsible for billions of dollars in damage to global infrastructure each year. This suggests there are systematic deficiencies in the way that structures are designed to withstand this type of event and that there is a need to better understand the hazard convective wind storms pose to infrastructure. In order to quantify this risk, it is necessary to have a reliable and spatially complete climatology of their occurrence. Given no such climatology exists for Australia, this research sought to develop such a climatology based on recent observational records, as well as examining how climate change may impact the severe convective wind storm climate into the future. Severe weather records (including wind gusts) in the Australian Bureau of Meteorology’s Severe Storm Archive are spatially and temporally incomplete and are therefore inadequate for developing a reliable climatology. In contrast, approximately 600 Automatic Weather Stations (AWS) around the country now produce 1-minute data records for several atmospheric variables (including wind speed and direction). These records offer a source of data that can be reliably analysed. However, analysis of these data presents challenges, primarily including, a) identifying the weather mode (e.g., convective, frontal, strong pressure gradients, pressure systems) responsible for each extreme gust, and b) overcoming the incomplete spatial coverage of the network. To overcome these challenges, this work utilises Self-Organizing Maps (SOM) as an automated method for classifying the mode of each observed gust, and then applies hierarchical Bayesian statistics to extend the analysis to regions where no AWS records exist. With the increasing use and acceptance of SOM algorithms for classifying atmospheric data, this machine learning technique was used to objectively identify severe wind storms from 1- minutre AWS data. The SOM algorithm was applied to a small subset of AWS stations so that the SOMs could be trained, and their performance verified. Given the large number of free parameters built into the SOM algorithm, it was first essential to conduct a proper sensitivity analysis to determine the set up for the SOM. Upon selecting the best combination of free parameters to run the SOMs, different combinations of atmospheric variables were explored, including: wind speed, change in wind direction, temperature, mean sea-level pressure, precipitation and equivalent potential temperature. Various statistical tools were used to determine how well the SOM algorithm was able to identify convective events compared to a manual identification of events. It was found that by considering wind speed alone, the SOM was able to perform well compared to methods that combine other variables such as temperature, pressure, and change in wind direction. ii To extend this station-based analysis and facilitate the development of a spatially complete convective wind storm climatology across the Australian continent, observational and global reanalysis data are coupled to determine the probability of severe wind storms occurring in different parts of Australia, even where there is no observational data available. A Bayesian hierarchical framework was used to develop the relationship between the SOM identified AWS convective events and severe weather indices calculated from ERA-Interim reanalysis data, while minimizing the impact of the spatial and temporal biases inherit to the AWS data. Using this model, the expected number of severe wind storms occurring in all parts of Australia was estimated. The Bayesian model was run using data between 2005-2015 and showed that there are significantly more severe convective wind storms occurring in northern Western Australia, southern Northern Territory and western Queensland than observational datasets show. Resampling techniques minimised the effects of the short observational period and helped determine the index or indices that best relates the observational and reanalysis data. These relationships were then used to extend the length of the observed dataset over the entire ERA- Interim reanalysis period (1979-2015). The flexibility of the Bayesian Hierarchal model allows the ERA-Interim reanalysis data to be replaced by other global datasets, including global climate models. Here, the Bayesian model is run with CMIP5 data to estimate how the climatology of severe convective wind storms might change under different climate scenarios. The BOM-CSIRO ACCESS-CM 1.3 under the RCP8.5 scenario was used. Mean severe weather indices were calculated for the projection period of 2090-2100 and the historical period from 1990-2000. Using this global model input for the Bayesian model, the change in the severe convective wind storm event counts from 1990-2000 to 2090-2100 were examined. To understand potential changes to convective wind storm hazard under an Intergovernmental Panel on Climate Change (IPCC) climate change scenario, large-scale global climate model environmental parameters (i.e. CAPE, Wind Shear) used in the stochastic model to estimate convective wind storm frequency were studied. Running the stochastic model with these “changed” environments showed that an increase in the number of severe convective wind storms can be expected during the spring, summer, and autumn, especially over northern Western Australia, and Queensland. This resulting data can be used to generate hazard maps and stochastic event sets to inform wind- resistant design standards and facilitate risk-based decision making by government and industry. iii Declaration by author This thesis is composed of my original work, and contains no material previously published or written by another person except where due reference has been made in the text. I have clearly stated the contribution by others to jointly-authored works that I have included in my thesis. I have clearly stated the contribution of others to my thesis as a whole, including statistical assistance, survey design, data analysis, significant technical procedures, professional editorial advice, financial support and any other original research work used or reported in my thesis. The content of my thesis is the result of work I have carried out since the commencement of my higher degree by research candidature and does not include a substantial part of work that has been submitted to qualify for the award of any other degree or diploma in any university or other tertiary institution. I have clearly stated which parts of my thesis, if any, have been submitted to qualify for another award. I acknowledge that an electronic copy of my thesis must be lodged with the University Library and, subject to the policy and procedures of The University of Queensland, the thesis be made available for research and study in accordance with the Copyright Act 1968 unless a period of embargo has been approved by the Dean of the Graduate School. I acknowledge that copyright of all material contained in my thesis resides with the copyright holder(s) of that material. Where appropriate I have obtained copyright permission from the copyright holder to reproduce material in this thesis and have sought permission from co- authors for any jointly authored works included in the thesis. Alessio Spassiani Doctor Matthew Mason January, 2020 January, 2020 iv Publications included in this thesis No publications included. v Submitted manuscripts included in this thesis No manuscripts submitted for publication. vi Other publications during candidature Conference Abstracts Spassiani, A.C., M. Mason, R.J. Krupar III, V. Cheng. “An Australian Climatology of Severe Convective Wind Storms Using Bayesian Hierarchical Modelling.” Oral presentation as part of the 29th Conference on Severe Local Storms, Stowe, VT., October 2018. Spassiani, A.C., M. Mason, R.J. Krupar III. “Bayesian statistical approach for developing a convective wind storm climatology of Australia.” Oral presentation as part of the AMOS 2018 Conference, Sydney, NSW., February 2018. Spassiani, A.C., M. Mason, R.J. Krupar III. “Estimating severe wind storm occurrence across Australia using Bayesian modelling.” Poster presentation as part of the ECSS 2017 Conference, Pula, Croatia., September 2017. Spassiani, A.C., M. Mason, R.J. Krupar III. “A probabilistic method for estimate severe weather event occurrence frequency using Bayesian statistics.” Oral presentation as part of the AMOS 2017 Conference, Canberra, ACT., February 2017. vii Contributions by others to the thesis This thesis is part of a larger project originally designed by Dr. Matthew Mason, titled “Characterising the hazard, structure and impacts of severe convective wind storms”. Statement of parts of the thesis submitted to qualify for the award of another degree No works submitted towards another degree have been included in this thesis. Research Involving Human or Animal Subjects No animal or human subjects were involved in this research. viii Acknowledgements First and foremost, I would like to thank my parents, Angelina and Alessandro Spassiani, for all their love and support over the years, especially during my academic years abroad. Without them none of this would have been possible. I would also like to thank my siblings and their partners for always being there, Gian-Paolo and
Details
-
File Typepdf
-
Upload Time-
-
Content LanguagesEnglish
-
Upload UserAnonymous/Not logged-in
-
File Pages250 Page
-
File Size-