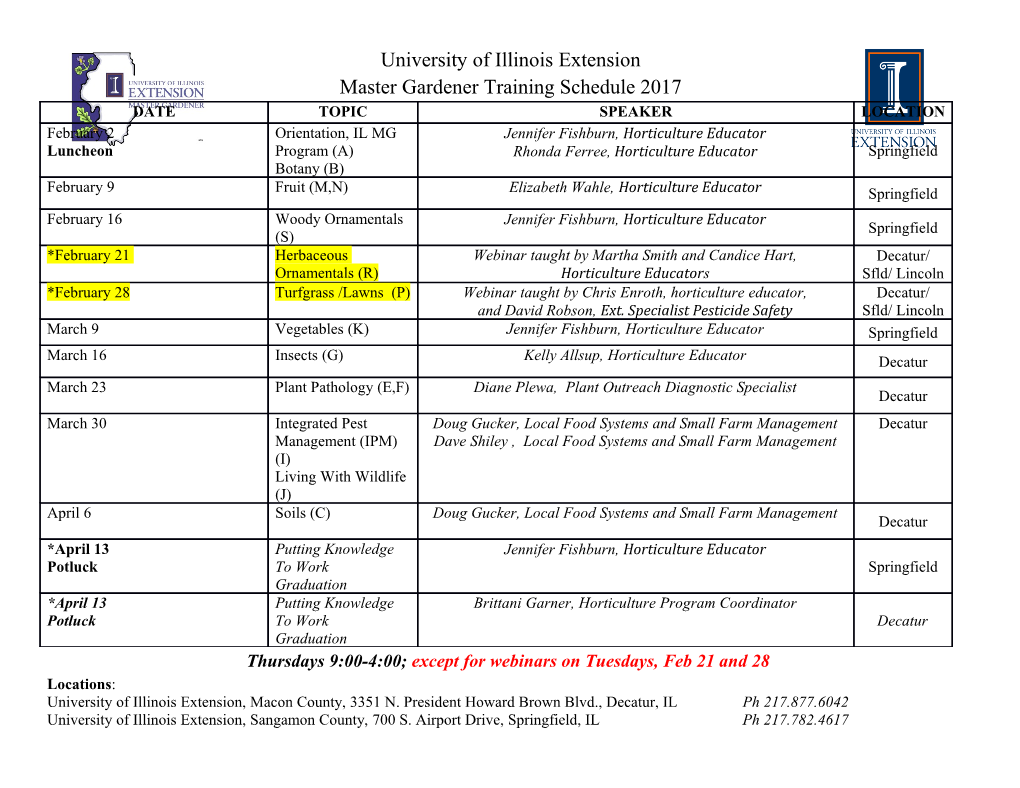
BAYESIAN ASSEMBLY OF READS FROM HIGH THROUGHPUT SEQUENCING A DISSERTATION SUBMITTED TO THE DEPARTMENT OF COMPUTER SCIENCE AND THE COMMITTEE ON GRADUATE STUDIES OF STANFORD UNIVERSITY IN PARTIAL FULFILLMENT OF THE REQUIREMENTS FOR THE DEGREE OF DOCTOR OF PHILOSOPHY Jonathan Laserson March 2012 © 2012 by Jonathan Daniel Laserson. All Rights Reserved. Re-distributed by Stanford University under license with the author. This work is licensed under a Creative Commons Attribution- Noncommercial 3.0 United States License. http://creativecommons.org/licenses/by-nc/3.0/us/ This dissertation is online at: http://purl.stanford.edu/xp796hy4748 ii I certify that I have read this dissertation and that, in my opinion, it is fully adequate in scope and quality as a dissertation for the degree of Doctor of Philosophy. Daphne Koller, Primary Adviser I certify that I have read this dissertation and that, in my opinion, it is fully adequate in scope and quality as a dissertation for the degree of Doctor of Philosophy. Serafim Batzoglou I certify that I have read this dissertation and that, in my opinion, it is fully adequate in scope and quality as a dissertation for the degree of Doctor of Philosophy. Andrew Fire Approved for the Stanford University Committee on Graduate Studies. Patricia J. Gumport, Vice Provost Graduate Education This signature page was generated electronically upon submission of this dissertation in electronic format. An original signed hard copy of the signature page is on file in University Archives. iii iv Abstract The high-throughput sequencing revolution allows us to take millions of noisy short reads from the DNA in a sample, essentially taking a snapshot of the genomic material in the sample. To recover the true genomes, these reads are assembled by algorithms exploiting their high coverage and overlap. In this thesis, I focus on two scenarios for sequence assembly. In both cases I use the same principled Bayesian approach to design an algorithm that uncovers the composition of the genomic sequences that produced the reads. The first scenario is Metagenomics, a de novo assembly task where the reads come from an unknown and diverse population of genomes. Here the challenge stems from uncertainty over the sample composition, read coverage, and noise levels. We developed Genovo, an assembler driven by a probabilistic model of the process of read generation. A nonparametric prior accounts for the unknown number of genomes and their relative abundance in the sample. We compared our method to three other short read assembly programs, over real and synthetic datasets. The results show that our Bayesian approach provides assemblies that outperform the state of the art on various measures. The second scenario is lineage assembly, where the reads come from short but clonally related genomes only slightly mutated from each other, generated over a small time scale. We developed ImmuniTree, a tool that builds a phylogenetic tree representing the mutated genomes, where the input reads are partitioned among the tree’s nodes. Unlike traditional phylogenetic trees, our trees are not necessarily binary, and we allow reads in internal nodes. The underlying model compounds a sequencing noise model on top of a mutation model, allowing the user to tell apart disagreements v between the reads that are due to random noise, versus an actual mutation. We demonstrated ImmuniTree on reconstruction of B cell lineages. In a healthy immune system, the B cells are produced with an incredible diversity, in an essentially random process. When a B cell detects an invader it starts cloning itself through a process of hypermutation, with B cells that detect the invader better selected over those that do not. We collected samples from 10 organ donors, each providing four tissue samples. Reads taken from the samples cover each the variable region of the heavy chain in a single B cell receptor. To analyze this data, we clustered the reads into clones and built a tree for each one using ImmuniTree. Our findings show that many of the clones are shared by B cells from multiple tissues, which suggests a high degree of B cell migration. In addition, our results show other aspects of B cell production in which the four tissues differ. vi Acknowledgments First, I’d like to thank Daphne Koller, my advisor, who taught me about sharp and uncompromising research. Throughout my PhD I worked on a number of projects in different fields, and one of the things I most appreciate is that each and every one of those projects was addressing a central problem in its field. These projects opened doors to amazing opportunities and collaborations. I want to thank Daphne for her guidance, and her genuine interest in the success of all my projects. Vladimir Jojic, with whom I worked closely for more than a year, was also a great influence on my PhD path. He taught me a lot about MCMC and computational Biology. In fact, he is the reason I transitioned from computer vision to Biology, for better or worse. The collaboration with Scott Boyd and Andrew Fire was particularly enjoyable. They devoted a ton of their time discussing with me the different aspects of their data, and offered many research trajectories and help. I also want to thank their students, Katherine Jackson and Elyse Hope for streamlining the data from their side, and Clauss Niemann for collecting and sequencing the samples. I want to thank Serafim Batzoglou and his wonderful students for helpful discus- sions, and also for adopting me when I was all alone in that conference in Lisbon. I will never forget the day I gave a talk to your group. Through the PhD my funding that made this work possible came from the Stan- ford Graduate Fellowship, and also National Science Foundation Grant BDI-0345474. Many other people helped me through my time at Stanford, either directly to my research or simply by making the lab a happy place. I cannot possibly list here all of them. But I will name a few. Geremy Heitz was my first mentor in Daphne’s group, vii back when I was doing vision. I learned a lot from him about coding paradigms and running jobs on the cluster. Ben Packer and I were close friends from day one in Stanford, and soon after we became office-mates and colleagues in Daphne’s group. This makes him the person who had spent the most time in the same room with me in all of California, and we are still friends. Karen Sachs is both a good friend and one of those rare people who nativley knows both Computer Science and Biology. Almost every paper I wrote or presented went through her eyes first. My thanks go to all the other talented members of the DAGS group, especially Gal Elidan, Alexis Battle, Yoni Donner, Manfred Claassen, Such Saria, and Cristina Pop. Also thanks to my cousin, Uri Laserson, for many useful and stimulating conversations as somehow we ended up working in the same domain. During my nomadic search for purpose in my first year at Stanford, I enjoyed the interaction with many Stanford Professors, many in the Psychology department. I spent a few months in the groups of Vladlen Koltun, who also supported me finan- cially, and Lera Boroditsky. Even though I turned out to take a different academic route, I feel that what I learned in that first year profoundly shaped my interests and views. Many of the PhD students who joined the CS department the same year as I became great friends and companions. I want to thank Daniel Ramage, Siddhartha Chaudhuri, and Paul Heymann. Special thanks to Aleksandra Korolova for a true friendship that sadly did not last. For great friendship and good times that go beyond Stanford, I want to thank Orly Fuhrman, who became my housemate, and who was briefly my lab-mate as well, Leor Vartikovski, who quickly became the key ingredient of my social life, and also Shlomi, Roee, Inbal & Micha, Rachel, Eran, Arash, Maya, Rafit (and family), Joy, Noam, Nir, Inbal & Noy, Sivan, Pamela & Ophir, Natalie, Sarah, and Amy. Thanks also to my good old pals from Israel, Zvika, Eyal, Aviv, Gilad, Alex and Tair. Last, I want to thank my family. For my parents, Aliza and Avi, I think the PhD was the first time they ever saw me struggle, and they gave me tremendous and continuous support all the way from Israel, along with good advice and unconditional love. My sister Mia, my brother Itamar, his wife Ayelet, and all my beloved extended viii family, you all made me feel how fun it is to have the family near by, and this is one of the best reasons I can think of for coming back home. ix Contents Abstract v Acknowledgments vii 1 Introduction 1 1.1 Contributions ............................... 4 1.2 ThesisOutline............................... 5 1.3 Previously Published Work . 6 2 Preliminaries 7 2.1 TheJointDistribution .......................... 7 2.1.1 Independence ........................... 11 2.2 BayesianNetworks ............................ 12 2.2.1 PlateModels ........................... 14 2.2.2 Inference.............................. 15 2.2.3 Finding The MAP Assignment . 18 2.3 CommonDistributions . .. .. 19 2.3.1 DirichletDistribution. 19 2.4 MarkovChainMonteCarlo . 23 2.4.1 GibbsSampling.......................... 26 2.4.2 Using MCMC to Get the MAP Assignment . 26 2.4.3 MCMC With Collapsed Particles . 27 2.4.4 MCMC With Auxiliary Variables . 27 2.4.5 SliceSampling........................... 27 x 2.4.6 Example: Bayesian Clustering . 29 2.5 Non-ParametricModels . .. .. 32 2.5.1 Example: Bayesian Clustering - Continued . 32 2.5.2 The Chinese Restaurant Process . 34 2.5.3 TheDirichletProcess. 35 3 De novo Sequence Assembly 37 3.1 Background ...............................
Details
-
File Typepdf
-
Upload Time-
-
Content LanguagesEnglish
-
Upload UserAnonymous/Not logged-in
-
File Pages184 Page
-
File Size-