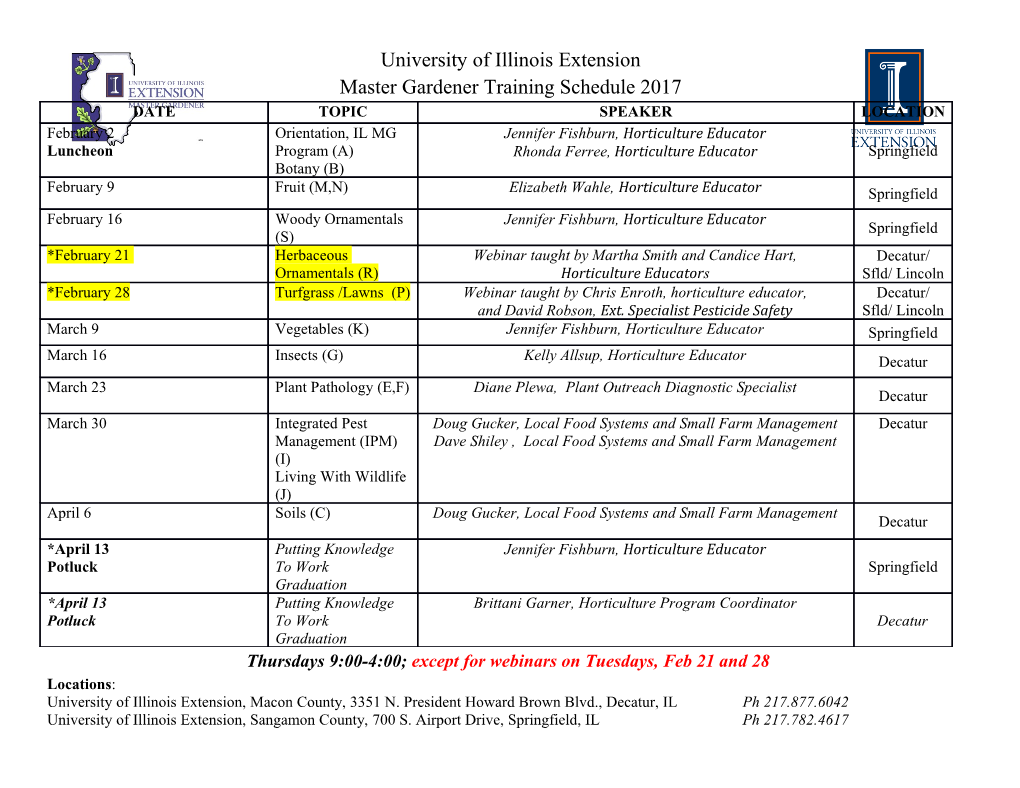
Bayesian Linear Model: Gory Details Pubh7440 Notes By Sudipto Banerjee n Let y = [yi]i=1 be an n × 1 vector of independent observations on a dependent variable (or response) from n experimental units. Associated with the yi, is a p × 1 vector of regressors, say xi, and lead to the linear regression model y = Xβ + , (1) T n T where X = [xi ]i=1 is the n × p matrix of regressors with i-th row being xi and is assumed fixed, n β is the slope vector of regression coefficients and = [i]i=1 is the vector of random variables representing “pure error” or measurement error in the dependent variable. For independent obser- 2 iid 2 vations, we assume ∼ MVN(0, σ In), viz. that each component i ∼ N(0, σ ). Furthermore, we will assume that the columns of the matrix X are linearly independent so that the rank of X is p. 1 The NIG conjugate prior family A popular Bayesian model builds upon the linear regression of y using conjugate priors by specifying 2 2 2 2 p(β, σ ) = p(β | σ )p(σ ) = N(µβ, σ Vβ) × IG(a, b) = NIG(µβ,Vβ, a, b) ba 1 a+p/2+1 1 1 = × exp − b + (β − µ )T V −1(β − µ ) p/2 1/2 2 2 β β β (2π) |Vβ| Γ(a) σ σ 2 1 a+p/2+1 1 1 ∝ × exp − b + (β − µ )T V −1(β − µ ) , (2) σ2 σ2 2 β β β where Γ(·) represents the Gamma function and the IG(a, b) prior density for σ2 is given by ba 1 a+1 b p(σ2) = exp − , σ2 > 0, Γ(a) σ2 σ2 where a, b > 0. We call this the Normal-Inverse-Gamma (NIG) prior and denote it as NIG(µβ,Vβ, a, b). The NIG probability distribution is a joint probability distribution of a vector β and a scalar σ2. If (β, σ2) ∼ NIG(µ, V, a, b), then an interesting analytic form results from integrating out σ2 1 from the joint density: Z ba Z 1 a+1 1 1 NIG(µ, V, a, b)dσ2 = exp − b + (β − µ)T V −1(β − µ) dσ2 (2π)p/2|V |1/2Γ(a) σ2 σ2 2 ba Z 1 1 = exp − b + (β − µ)T V −1(β − µ) dσ2 (2π)p/2|V |1/2Γ(a) σ2 2 a p −(a+ p ) b Γ a + 1 2 = 2 b + (β − µ)T V −1(β − µ) (2π)p/2|V |1/2Γ(a) 2 2a+p " −1 #−( 2 ) Γ a + p (β − µ)T b V (β − µ) = 2 1 + a . p/2 b 1/2 2a π |(2a) a V | Γ(a) This is a multivariate t density: ν+p − ν+p Γ (β − µ)T Σ−1(β − µ) 2 MV St (µ, Σ) = 2 1 + , (3) ν ν p/2 1/2 Γ 2 π |νΣ| ν b with ν = 2a and Σ = a V . 2 The likelihood The likelihood for the model is defined, up to proportionality, as the joint probability of observing the data given the parameters. Since X is fixed, the likelihood is given by 1 n/2 1 p(y | β, σ2) = N(Xβ, σ2I) = exp − (y − Xβ)T (y − Xβ) . (4) 2πσ2 2σ2 3 The posterior distribution from the NIG prior Inference will proceed from the posterior distribution p(β, σ2)p(y | β, σ2) p(β, σ2 | y) = , p(y) where p(y) = R p(β, σ2)p(y | β, σ2)dβdσ2 is the marginal distribution of the data. The key to deriving the joint posterior distribution is the following easily verified multivariate completion of squares or ellipsoidal rectification identity: uT Au − 2αT u = (u − A−1α)T A(u − A−1α) − αT A−1α, (5) 2 where A is a symmetric positive definite (hence invertible) matrix. An application of this identity immediately reveals, 1 1 1 1 b + (β − µ )T V (β − µ ) + (y − Xβ)T (y − Xβ) = b∗ + (β − µ∗)T V ∗−1(β − µ∗) , σ2 2 β β β σ2 2 using which we can write the posterior as 1 a+(n+p)/2+1 1 1 p(β, σ2 | y) ∝ × exp − b∗ + (β − µ∗)T V ∗−1(β − µ∗) , (6) σ2 σ2 2 where ∗ −1 T −1 −1 T µ = (Vβ + X X) (Vβ µβ + X y), V ∗ = (V −1 + XT X)−1, a∗ = a + n/2, 1 b∗ = b + [µT V −1µ + yT y − µ∗T V ∗−1µ∗]. 2 β β β This posterior distribution is easily identified as a NIG(µ∗,V ∗, a∗, b∗) proving it to be a conjugate family for the linear regression model. Note that the marginal posterior distribution of σ2 is immediately seen to be an IG(a∗, b∗) whose density is given by: ∗ b∗a∗ 1 a +1 b∗ p(σ2 | y) = exp − . (7) Γ(a∗) σ2 σ2 The marginal posterior distribution of β is obtained by integrating out σ2 from the NIG joint posterior as follows: Z Z p(β|y) = p(β, σ2 | y)dσ2 = NIG(µ∗,V ∗, a∗, b∗)dσ2 ∗ Z 1 a +1 1 1 ∝ exp − b∗ + (β − µ∗)T V ∗−1(β − µ∗) dσ2 σ2 σ2 2 ∗ (β − µ∗)T V ∗−1(β − µ∗)−(a +p/2) ∝ 1 + . 2b∗ This is a multivariate t density: ∗ ∗ ν +p − ν +p Γ ∗ T ∗−1 ∗ 2 ∗ ∗ 2 (β − µ ) Σ (β − µ ) MV St ∗ (µ , Σ ) = 1 + , (8) ν ν∗ p/2 ∗ ∗ 1/2 ∗ Γ 2 π |ν Σ | ν ∗ ∗ ∗ b∗ ∗ with ν = 2a and Σ = a∗ V . 3 4 A useful expression for the NIG scale parameter Here we will prove: 1 −1 b∗ = b + y − Xµ T I + XV XT (y − Xµ ) (9) 2 β β β On account of the expression for b∗ derived in the preceding section, it suffices to prove that T T −1 ∗ ∗−1 ∗ T T −1 y y + µβ V µβ − µ V µ = y − Xµβ I + XVβX (y − Xµβ) ∗ ∗ −1 T Substituting µ = V (V µβ + X y) in the left hand side above we obtain: T T −1 ∗ ∗−1 ∗ T T −1 −1 T ∗ −1 T y y + µβ Vβ µβ − µ V µ = y y + µβ Vβ µβ − (Vβ µβ + X y)V (Vβ µβ + X y) T ∗ T T ∗ −1 T −1 −1 ∗ −1 = y (I − XVβ X )y − 2y XV V µβ + µβ (Vβ − Vβ V Vβ )µ. (10) Further development of the proof will employ two tricky identities. The first is the well-known Sherman-Woodbury-Morrison identity in matrix algebra: −1 (A + BDC)−1 = A−1 − A−1B D−1 + CA−1B CA−1, (11) where A and D are square matrices that are invertible and B and C are rectangular (square if A and D have the same dimensions) matrices such that the multiplications are well-defined. This identity is easily verified by multiplying the right hand side with A + BDC and simplifying to reduce it to the identity matrix. T −1 Applying (11) twice, once with A = Vβ and D = (X X) to get the second equality and then T −1 with A = (X X) and D = Vβ to get the third equality, we have −1 −1 ∗ −1 −1 −1 −1 T −1 −1 Vβ − Vβ V Vβ = Vβ − Vβ (Vβ + XX ) Vβ T −1 −1 = [Vβ + (X X) ] = XT X − XT X(XT X + V −1)−1XT X T ∗ T = X (In − XV X )X. (12) ∗ −1 T ∗ −1 ∗ T The next identity notes that since V (V + X X) = Ip, we have V V = Ip − V X X, so that ∗ −1 ∗ T ∗ T XV V = X − XV X X = (In − XV X )X. (13) 4 Substituting (12) and (13) in (10) we obtain T ∗ T T ∗ T T ∗ T y (In − XV X )y − 2y (In − XV X )µβ + µβ (In − XV X )µβ T ∗ T = (y − Xµβ) (In − XV X )(y − Xµβ) T T −1 = (y − Xµβ) (In + XVX ) (y − Xµβ), (14) where the last step is again a consequence of (11): T −1 −1 T −1 T ∗ T (In + XVX ) = In − X(V + X X) X = In − XV X . 5 Marginal distributions – the hard way To obtain the marginal distribution of y, we first compute the distribution p(y | σ2) by integrating out β and subsequently integrate out σ2 to obtain p(y). To be precise, we use the expression for b∗ derived in the preceding section, proceeding as below: Z Z 2 2 2 2 2 p(y | σ ) = p(y | β, σ )p(β | σ )dβ = N(Xβ, σ In) × N(µβ, σ Vβ)dβ Z 1 1 n T T −1 o = n+p exp − (y − Xβ) (y − Xβ) + (β − µβ) Vβ (β − µβ) dβ 2 1/2 2σ2 (2πσ ) 2 |Vβ| 1 = n+p 2 1/2 (2πσ ) 2 |Vβ| Z 1 × exp − (y − Xµ )T (I + XVXT )−1(y − Xµ ) + (β − µ∗)T V ∗−1(β − µ∗) dβ 2σ2 β β 1 1 T T −1 = n+p exp − (y − Xµβ) (I + XVβX ) (y − Xµβ) 2 1/2 2σ2 (2πσ ) 2 |Vβ| Z 1 × exp − (β − µ∗)T V ∗−1(β − µ∗) dβ 2σ2 ∗ 1/2 1 |V | 1 T T −1 = n exp − 2 (y − Xµβ) (I + XVβX ) (y − Xµβ) (2πσ2) 2 |Vβ| 2σ 1 1 T T −1 = n exp − (y − Xµβ) (I + XVβX ) (y − Xµβ) 2 T 1/2 2 (2πσ ) 2 |I + XVβX | 2σ 2 T = N(Xµβ, σ (I + XVβX )). (15) Here we have applied the matrix identity |A + BDC| = |A||D||D−1 + CA−1B| (16) 5 to obtain |V | |I + XV XT | = |V ||V −1 + XT X| = β .
Details
-
File Typepdf
-
Upload Time-
-
Content LanguagesEnglish
-
Upload UserAnonymous/Not logged-in
-
File Pages9 Page
-
File Size-