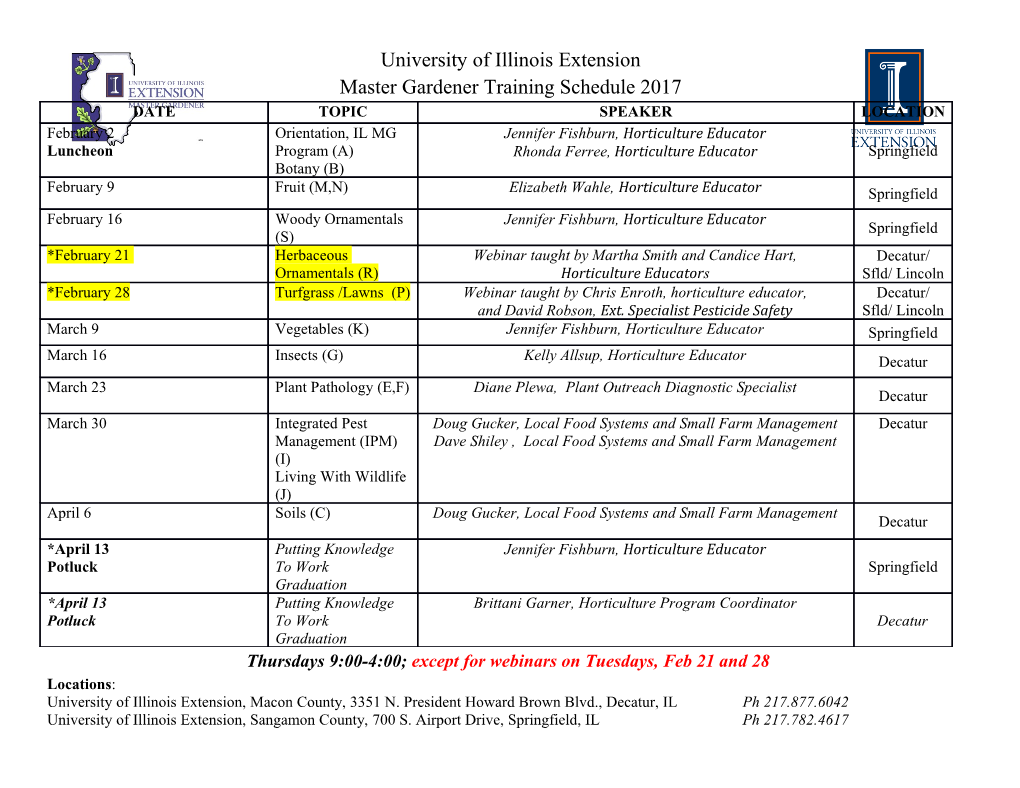
PUNCTUALITY FORECASTING AND BOTTLENECK IDENTIFICATION AT KLM AIRCRAFT SERVICES Paul Adriaansen S257389 April 2009 Thesis submitted in partial fulfillment of the requirements for the degree in Master of Science in Operations Research & Management Science Supervised by: Dr. J.P.C. Blanc, Department of Econometrics & Operations Research, Tilburg University, and: Ir. M. Bovenkerk, KLM Aircraft Services THESIS PAUL ADRIAANSEN –APRIL 2009 ii Pablo Ruiz y Picasso 1881 - 1973 THESIS PAUL ADRIAANSEN –APRIL 2009 iii THESIS PAUL ADRIAANSEN –APRIL 2009 iv MANAGEMENT SUMMARY Context and research goals KLM Aircraft Services (AS) is responsible for the turnarounds of aircrafts. A turnaround is the time in between two flights in which the aircraft is cleaned, fuelled, reloaded with catering supplies, reloaded with fresh water and prepared for the next flight. Since many aircrafts are handled at the same time and all turnarounds must be performed within limited time, AS needs to make the allocation of resources (equipment and staff) as efficient as possible. When the aircraft is not ready for departure at the moment it is supposed to leave according to the timetable, the aircraft is delayed. Punctuality is an important KPI for the KLM management, as well as the management of AS and its departments. But instead of measuring punctuality afterwards, KLM AS would rather have a tool for forecasting the consequences in punctuality of decisions in resource allocation and to facilitate scenario analysis for trading off costs and punctuality. The number of resources available during the day for aircraft handling is currently based on the workload during the day, based on the timetable. The Operational Check (OPC) is performed half a year in advance and tests the proposed timetable on feasibility for all AS processes. This test is performed by minimizing the number of resources needed to perform all jobs according to the timetable, with deterministic norms for the job durations. Since the timetable is assessed in a deterministic way, it is impossible to take punctuality forecasting into account in the OPC. Forecasting punctuality is especially interesting if the processes responsible for the delay can be identified. Altogether, the main goal of the project as assigned by KLM Aircraft Services is: 1. to evaluate definitions of punctuality in the context of KLM Aircraft Services, 2. to perform quantitative analysis on the measurement and forecasting of punctuality and the identification of bottlenecks in the platform operation, and 3. to find a way in which any form of punctuality forecasting can be included in the OPC. THESIS PAUL ADRIAANSEN –APRIL 2009 iii Simulation model The third goal as stated above implicitly incorporates the first two goals and was met by developing a simulation model (called Picasso), based on the inputs of the OPC. The Picasso simulation model was based on the existing OPiuM simulation model (programmed in Java with the DSOL simulation package). OPiuM is used for forecasting punctuality of flights by simulating deviations from the timetable, based on historical data of flight and ground time durations. The discrete-event simulation model that is used for this thesis combines the concept of job shop scheduling in a stochastic environment with the concept of a queuing network. These two concepts interact as follows: Aircrafts arrive and need different jobs (in prescribed order) before they can depart These jobs are performed by resources that are unique for the process the job belongs to When a resource is assigned to a certain job, it first needs to drive to the aircraft before it can start. Both driving and processing takes some stochastic period of time However, these resources are scarce which means jobs often need to queue within a process In order to minimize delays and waiting time (idle time of resources which eventually leads to delays as well) the system uses intelligent tools to allocate resources in an efficient way and give priority to jobs that are about to cause delays As described, the OPC minimizes the number of resources needed to be able to perform all platform operations according to the (deterministic) timetable. Picasso, on the other hand, does exactly the opposite: it minimizes delays (defined as lateness of departure) subject to performing all platform operations with a given number of resources. The arrival moments of aircrafts are subject to uncertainty (simulated by OPiuM) and the job durations are stochastic as well, based on historical data. The number of resources available for each process during the day in the Picasso model is taken equal to the output of the OPC trajectory: the minimal number of resources needed to perform a deterministic timetable (at 100% punctuality) with deterministic job durations. The lateness of a departure is measured in minutes, but in order to take the size of the aircraft into account, the delay is multiplied with the number of passengers in the aircraft, which results in the punctuality measure of Passenger Delay Minutes (PDM). By means of an allocation rule, based on “fairness principles”, the total PDM of a flight is assigned to one or more processes that are responsible for the delay. By adding up these PDM per process, bottlenecks in the operation (processes with too little resources) can be identified for every moment of the day. Results, conclusions and recommendations Since Picasso is only a prototype of a simulation model that could be used in the OPC, the emphasis of the process was not on the quality of the programming. Therefore some imperfections exist in producing multiple independent runs and replications which turns out to be troublesome when performing statistical analysis. However, by making some assumptions about independence based on the output data, it turned out to be possible to compare different scenario’s on their input parameters. Although absolute values in the output showed strong deviations from expected numbers, due to various reasons, the relative differences in the output numbers did provide useful results. A lot of significant results were found by using the Welch approximation for constructing Confidence Intervals with the two-sample t-test. Due to the elaborate nature of performing an OPC, KLM did not have the possibility to perform more than one OPC trajectory for the Picasso project. Without having multiple sets of capacity levels as the output of an OPC trajectory it was not possible to test different timetables on their performance. The one timetable that was examined (timetable of summer 2007) has been tested on several aspects to test its validity and to proof the usefulness of Picasso as a punctuality forecasting tool. The results of these tests include (apart from verification and validation): In general, the cleaning and security check processes (and to a lesser extent the fuelling process) are the main causes of delay according to Picasso THESIS PAUL ADRIAANSEN –APRIL 2009 iv The problems with these processes (bottlenecks in the operation) mainly occur during the wide morning peak between 7.00 and 9.00 (bottleneck: cleaning) and during the high evening peak between 18.30 and 19.30 (bottleneck: security check). Other peaks are much less problematic During the sensitivity analysis it became clear that there exists a strong positive correlation between delays in fuelling and pushback en between delays in cleaning and security check. This correlation means that a shortage of capacity in one process, can induce problems at another process as well During the early morning (5.00 to 6.00) many long delays are caused by multiple reasons. Scenario analysis with Picasso shows that a small investment in extra capacity can easily solve most delays in this early morning peak The second and fourth result presented above are in line with a more general notion in the conclusion of this thesis: The current analyses in the OPC pay too much attention to the height of a peak in the workload, while they should focus more on its domain during the day. In the day-to-day operation numerous sources of uncertainty shift workload back and forth in time, so that peaks are truly unpredictable. A workload forecasting tool that takes punctuality (and thus uncertainties) into account should be robust in its results instead of precise in its predictions. This last conclusion introduces the most important recommendation following from this thesis: it is possible to implement an improved and extended version of Picasso as a punctuality forecasting tool in the OPC trajectory. The fact that Picasso has not yet proved to be able to test different timetables on their punctuality performance is no impediment to that, since this thesis shows Picasso is capable of providing valid and significant results in comparing scenario’s. However, the most important notion to this recommendation is that the whimsicalities of the day-to-day operation should never be forgotten when trying to model uncertainties. Modeling uncertainties is necessary when forecasting punctuality, but the simulation model itself is not necessarily getting more realistic by it. THESIS PAUL ADRIAANSEN –APRIL 2009 v TABLE OF CONTENTS PREFACE AND ACKNOWLEDGEMENTS xi CHAPTER I –INTRODUCTION 1 1.1 Project description 1 1.2 Research question and requirements 2 1.3 Importance and use of the project 3 1.4 Outline research approach and content of chapters 4 CHAPTER II –DETAILED PROBLEM DESCRIPTION 6 2.1 The organization 6 2.1.1 KLM Royal Dutch Airlines 6 2.1.2 Ground Services 7 2.1.3 Aircraft
Details
-
File Typepdf
-
Upload Time-
-
Content LanguagesEnglish
-
Upload UserAnonymous/Not logged-in
-
File Pages170 Page
-
File Size-