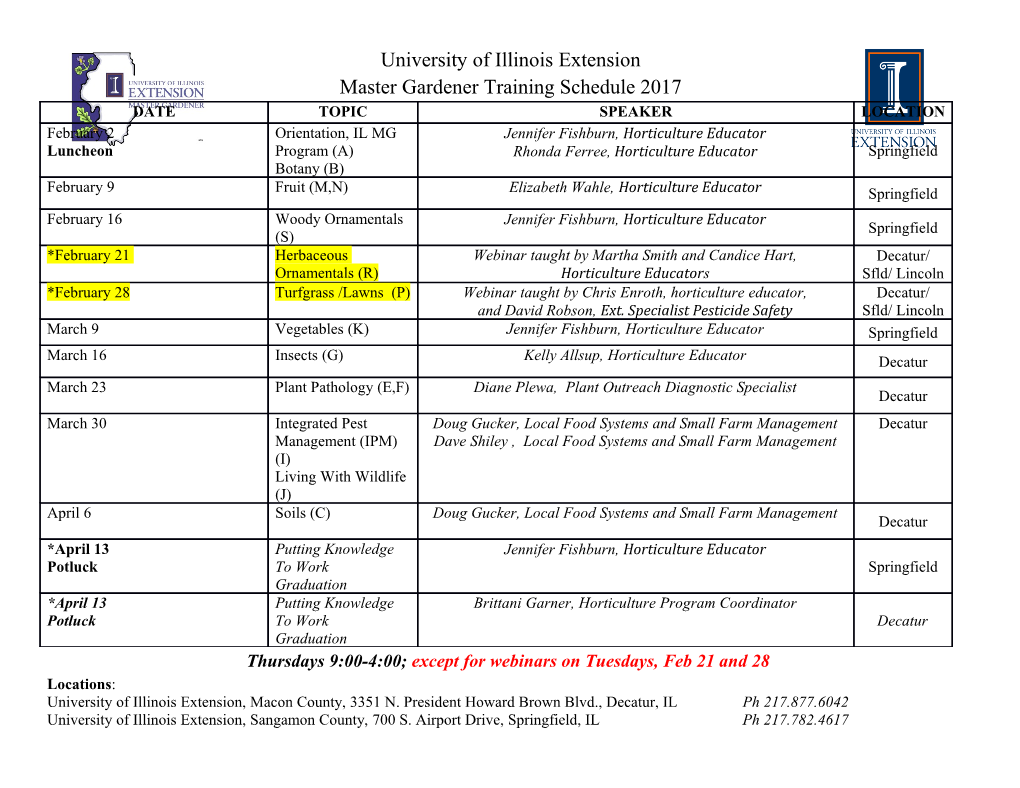
R E S E A R C H A R T I C L E ABSTRACT: Contemporary losses of early-successional young forest and shrubland habitat have resulted in range-wide population declines of numerous wildlife species, establishing shrubland conservation as a high priority in the eastern United States. However, the extent and spatial distribution of shrubland habitat is lacking for many locations. The Landsat program is an important resource for quantifying vegetation and land cover, but recent applications indicate that divergent structural characteristics between certain feature classes, such as vegetation height, are not readily captured by spectral response alone. • The fusion of Landsat imagery with light detection and ranging (LiDAR) data at the pixel level into existing supervised classification schemes may offset this discrepancy. We used multispectral images from Enhancing Forest Landsat 8’s Operational Land Imager (OLI) to compare Landsat OLI and Landsat OLI-LiDAR fusion data in the classification of 10 vegetation and land cover types across a ~5000 km2 area in southeastern Ohio at the native 30-m Landsat resolution. Fusion data produced a 12% increase in overall classifica- and Shrubland tion accuracy from the Landsat OLI model and met minimum mapping accuracy standards in remote sensing. Importantly, LiDAR textural bands in the fusion model improved discrimination of forest and shrubland classes with the most dramatic difference manifesting as a 75% increase in shrubland user’s Mapping in a accuracy. We demonstrate that Landsat OLI–LiDAR fusions are valuable for accurate shrubland mapping in forestlands. Additionally, we show that vegetation and land cover modeling can be easily integrated Managed Forest into existing wildlife monitoring programs by simultaneously sampling vegetation classes and wildlife communities from similar locations. Landscape with Index terms: forest management, LiDAR–Landsat data fusion, mapping accuracy, remote sensing, Landsat–LiDAR shrubland cover Data Fusion INTRODUCTION structure and growth stage, particularly shrubland extent, to address these crucial Many forests in the eastern United States conservation efforts. Bryce T. Adams1,3 are recovering from heavy exploitation in the 19th and early 20th centuries under Remotely sensed imagery provides a altered natural and anthropogenic distur- 1 comprehensive, consistent, and relatively School of Environment and bance regimes (Askins 2001; Lorimer cost-effective platform for the quanti- Natural Resources 2001; King and Schlossberg 2014). As fication of vegetation and land cover The Ohio State University a result, many forestlands are rapidly across broad geographic extents (Rogan Columbus, OH 43210 transitioning to intermediate stages of and Chen 2004; Fassnacht et al. 2006). development in absence of the disturbance Spaceborne imagery from the Landsat processes that historically maintained large program (a partnership between the United Stephen N. Matthews1,2 extents of early-successional young forests States Geological Survey [USGS] and the 2Northern Research Station and shrublands. Subsequently, these hab- National Aeronautics and Space Admin- US Department of Agriculture itats are becoming increasingly rare. For istration [NASA]) is one of the primary Forest Service example, Ohio experienced a 78% loss sources for concurrent globally available Delaware, OH 43015 in forestland <20 y in age, while forest- remote sensing data (Turner et al. 2015) land >60 y old increased by 68% during and is the foundation for the widely used 2007–2011 (Widmann et al. 2014). Long- National Land Cover Database (NLCD; • term trend data from the North American Danielson et al. 2015). Its long-running Breeding Bird Survey indicates that many data record and free and open-access plat- shrubland-dependent species, such as the form make Landsat data highly attractive yellow-breasted chat (Icteria virens L.) and to natural areas mapping (Turner et al. prairie warbler (Setophaga discolor Vie- 2015). Landsat-8, launched in 2013, is 3 Corresponding author: illot), have experienced concomitant and the latest operating satellite and includes [email protected]; (614) 247-1889 profound range-wide population declines a pushbroom multispectral instrument, the (Askins 1993; Ziolkowski et al. 2010). Operational Land Imager (OLI). While the The landscape-scale maintenance of forest Landsat program continues to experience a condition in both early- and later-growth growing spectrum of use cases, including stages has reached a high conservation the mapping of forest productivity (Bois- Associate editor: James Farmer priority in the eastern United States (King venue et al. 2016), stand volume (Chrysafis and Schlossberg 2014). Practitioners and et al. 2017), and disturbance-induced Natural Areas Journal 38:402–418 researchers require easy access to consis- assemblage change (Savage et al. 2017), tently acquired spatial information on forest the supervised classification of Landsat 402 Natural Areas Journal Volume 38 (5), 2018 Downloaded From: https://bioone.org/journals/Natural-Areas-Journal on 02 Oct 2020 Terms of Use: https://bioone.org/terms-of-use Access provided by United States Department of Agriculture National Agricultural Library (NAL) imagery in support of land use and land travel times of short-duration laser pulses unique vegetation types (e.g., floodplain cover (LULC) applications remains widely between the sensor and surface features. forest), which are important to regional popular (Schetter and Root 2011; Singh et The vast quantities of data are usually bird conservation yet lacking in standard al. 2012; Rose et al. 2015). Landsat-based summarized by transferring statistics of LULC classification systems (such as An- classifications of vegetation are highly the resulting “point cloud” to layered grids, derson et al. [1976] in which the NLCD is suited to forest management because of its where reflections at ground level (usually based), prompted us to pursue this study. spatial resolution being similar to the scale last returns or filtered ground points) are We classified multiband composite images of land management (Zhu and Liu 2014). used to create the DEM, and above-ground composed of either Landsat OLI imagery Indeed, Landsat imagery has been widely reflections (usually first or maximum height or Landsat OLI–LiDAR fusion data using used to map forest cover and type across returns) are used to construct the digital the classification-type Random Forests various landscapes (Foody and Hill 1996; surface model (DSM). The canopy height model. Map accuracy was assessed using Dorren et al. 2003; Zhu and Liu 2014). model (CHM), representing maximum total and three class-level accuracy metrics. vegetation height values (quantified by We predicted that added LiDAR textural However, image classification in support subtracting the DEM from the DSM), is bands in the fusion model would detect of LULC applications exhibits varying de- the most basic and commonly used mea- current forest and shrubland cover more grees of accuracy for certain feature classes. surement of LiDAR-derived vegetation accurately than spectral imagery alone. The Classification error arises most notably structure (Zimble et al. 2003). Perhaps the outcome of this study is also intended to where features of interest mix spatially or most straightforward approach to improve facilitate cross-disciplinary efforts between where compositional overlap between veg- quantification of forest and shrubland hab- studies of biodiversity and remote sensing etation assemblages produces similar spec- itats across forestlands is the integration (Pettorelli et al. 2014b, 2016; Vihervaara tral signatures (Irwin and Bockstael 2007; of data provided by these complementary et al. 2015). We integrated this study into Wickham et al. 2010). These errors have sensors into existing per-pixel classifica- our overall investigation of multi-scale been documented in a number of studies tion schemes. In this approach, the image bird response to forest management in using a variety of both satellite and airborne and LiDAR data are fused at the pixel southeastern Ohio. We demonstrate that imagery, particularly for differentiating level and processed together during model applications of remote sensing can be forest and vegetated open-canopy (some- calibration (Leckie et al. 2005). This is easily synchronized with existing field times explicitly shrubland-based) classes referred to as multi-sensor fusion (Hall and study (Pettorelli et al. 2014a). (Hill and Thompson 2005; Geerling et al. Llinas 1997), and the mixture of LiDAR 2007; Koetz et al. 2008; Verrelst et al. 2009; and optical remote sensing data have been METHODS Singh et al. 2012). For example, the NLCD explored in applications involving LULC often confuses forest-shrubland-grassland (Koetz et al. 2008; Singh et al. 2012) and cover types both spatially and temporally vegetation (Verrelst et al. 2009; Gu et al. Study Area and Sampling Design (Wickham et al. 2010, 2017). Forest- and 2015) mapping with promising results. Overview shrubland-based classes are often con- The added textural bands provided by fused during image classification due to LiDAR could provide improved mapping We defined a ~5000-km2 area as our intergrading species compositions (e.g., of shrubland cover within forestlands by mapping extent and study area (Figure 1). regenerating forest stands) that differ only providing height-above-ground informa- The study area is predominantly forested in vegetation height or canopy structure
Details
-
File Typepdf
-
Upload Time-
-
Content LanguagesEnglish
-
Upload UserAnonymous/Not logged-in
-
File Pages17 Page
-
File Size-