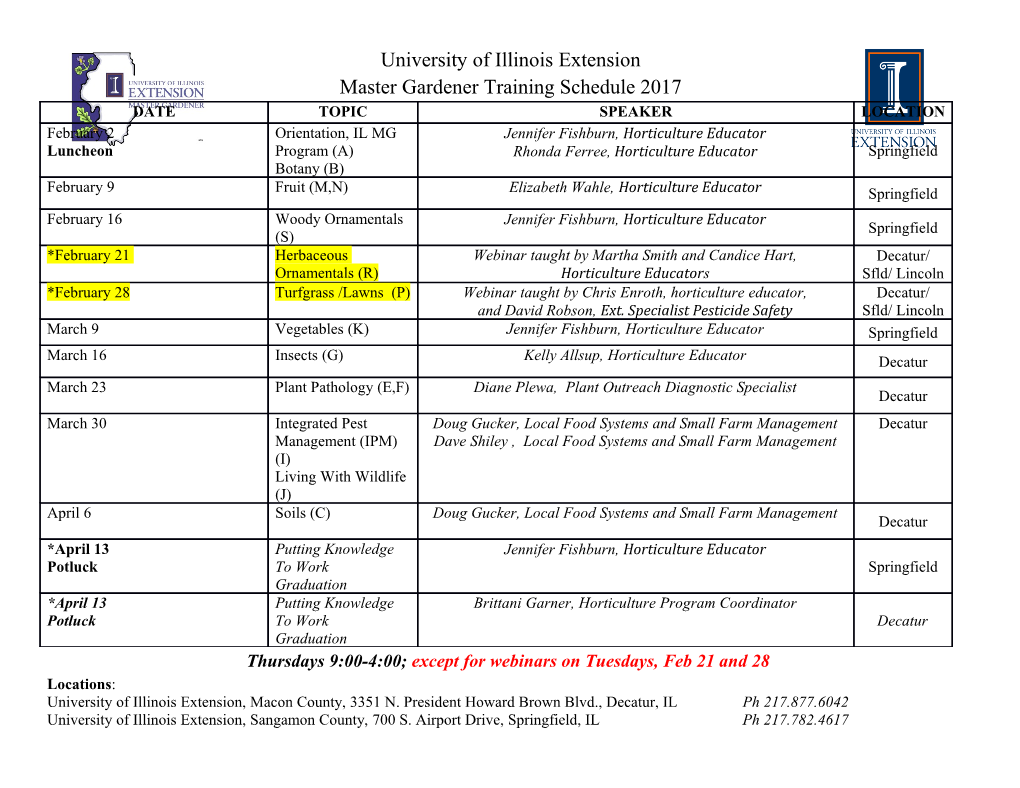
Adaptive Evaluation of Non-Strict Programs Robert Jonathan Ennals King’s College Dissertation submitted for the degree of Doctor of Philosophy University of Cambridge July 2, 2004 Declaration This dissertation is not substantially the same as any I have submitted for a degree or diploma or any other qualification at any other university. Further, no part of the dissertation has already been or is being currently submitted for any such degree, diploma or other qualification. This dissertation does not exceed 60,000 words. This total includes tables and footnotes, but excludes appendices, bibliography, and diagrams. Material from Chapters 2, 3 and 4 has been presented in a paper I co-authored with my supervisor [EP03b]. Chapter 5 is largely the same as a paper that I co-authored with my super- visor and with Alan Mycroft [EPM03]. Chapter 11 is largely identical to another paper that I co-authored with my supervisor [EP03a]. This dissertation is my own work and contains noth- ing which is the outcome of work done in collaboration with others, except as specified in the text. Robert Ennals, July 2, 2004 i Abstract Most popular programming languages are strict. In a strict language, the binding of a variable to an expression coincides with the evaluation of the expression. Non-strict languages attempt to make life easier for programmers by decoupling expression binding and expression evaluation. In a non-strict language, a variable can be bound to an unevaluated expression, and such expressions can be passed around just like values in a strict language. This separation allows the programmer to declare a variable at the point that makes most logical sense, rather than at the point at which its value is known to be needed. Non-strict languages are usually evaluated using a technique called Lazy Evaluation. Lazy Evaluation will only evaluate an expression when its value is known to be needed. While Lazy Evaluation minimises the total number of expressions evaluated, it imposes a considerable bookkeeping overhead, and has unpredictable space behaviour. In this thesis, we present a new evaluation strategy which we call Optimistic Evaluation. Optimistic Evaluation blends lazy and eager evaluation under the guidance of an online pro- filer. The online profiler observes the running program and decides which expressions should be evaluated lazily, and which should be evaluated eagerly. We show that the worst case per- formance of Optimistic Evaluation relative to Lazy Evaluation can be bounded with an upper bound chosen by the user. Increasing this upper bound allows the profiler to take greater risks and potentially achieve better average performance. This thesis describes both the theory and practice of Optimistic Evaluation. We start by giving an overview of Optimistic Evaluation. We go on to present a formal model, which we use to justify our design. We then detail how we have implemented Optimistic Evaluation as part of an industrial-strength compiler. Finally, we provide experimental results to back up our claims. ii Acknowledgments First of all, I would like to thank my supervisor, Simon Peyton Jones, who has provided in- valuable support, inspiration, and advice. Enormous thanks must go to Alan Mycroft, who has acted as my lab supervisor. I am also extremely grateful for the support of Microsoft Research, who have provided me not only with funding and equipment, but also with a supervisor, and a building full of interesting people. I would also like to thank Jan-Willem Maessen and Dave Scott, who provided suggestions on this thesis, and Manuel Chakravarty, Karl-Filip Faxen,´ Fergus Henderson, Neil Johnson, Alan Lawrence, Simon Marlow, Greg Morisett, Nick Nethercote, Nenrik Nilsson, Andy Pitts, Norman Ramsey, John Reppy, Richard Sharp, and Jeremy Singer, who have provided useful suggestions during the course of this work. This work builds on top of years of work that many people have put into the Glasgow Haskell Compiler. I would like to thank all the people at Glasgow, at Microsoft, and elsewhere, who have worked on GHC over the years. Special thanks must go to Simon Marlow, who was always able to help me when I ran into deep dark corners in the GHC runtime system. iii Contents 1 Introduction 1 1.1 Lazy Evaluation . 1 1.2 Mixing Eager and Lazy Evaluation . 2 1.3 Optimistic Evaluation . 3 1.4 Structure of this Thesis . 5 1.5 Contributions . 5 I Overview 7 2 Optimistic Evaluation 8 2.1 Switchable Let Expressions . 9 2.2 Abortion . 10 2.3 Chunky Evaluation . 11 2.4 Probability of a Nested Speculation being Used . 12 2.5 Exceptions and Errors . 13 2.6 Unsafe Input/Output . 13 3 Online Profiling 16 3.1 The Need for Profiling . 16 3.2 Goodness . 17 3.3 Calculating Wasted Work . 18 3.4 Burst Profiling . 22 iv CONTENTS v II Theory 24 4 Semantics 25 4.1 A Simple Language . 26 4.2 Operational Semantics . 27 4.3 Denotational Semantics . 34 4.4 Soundness . 39 5 A Cost Model for Non-Strict Evaluation 41 5.1 Why We Need a Cost Model . 42 5.2 Cost Graphs . 44 5.3 Producing a Cost View for a Program . 53 5.4 Rules for Evaluation . 55 5.5 An Operational Semantics . 58 5.6 Denotational Meanings of Operational States . 63 6 Deriving an Online Profiler 67 6.1 Categorising Work . 68 6.2 Blame . 72 6.3 Bounding Worst Case Performance . 78 6.4 Burst Profiling . 81 III Implementation 87 7 The GHC Execution Model 88 7.1 The Heap . 89 7.2 The Stack . 92 7.3 Evaluating Expressions . 95 7.4 Implementing the Evaluation Rules . 97 7.5 Other Details . 104 8 Switchable Let Expressions 105 8.1 Speculative Evaluation of a Let . 106 8.2 Flat Speculation . 111 8.3 Semi-Tagging . 114 8.4 Problems with Lazy Blackholing . 117 vi CONTENTS 9 Online Profiling 120 9.1 Runtime State for Profiling . 120 9.2 Implementing the Evaluation Rules . 124 9.3 Heap Profiling . 129 9.4 Further Details . 131 10 Abortion 135 10.1 When to Abort . 135 10.2 How to Abort . 137 11 Debugging 141 11.1 How the Dark Side Do It . 142 11.2 Failing to Debug Lazy Programs . 143 11.3 Eliminating Tail Call Elimination . 143 11.4 Optimistic Evaluation . 144 11.5 HsDebug . 145 IV Conclusions 146 12 Results 147 12.1 How the Tests were Carried Out . 148 12.2 Performance . 149 12.3 Profiling . 151 12.4 Metrics . 159 12.5 Semi-Tagging . 164 12.6 Heap Usage . 166 12.7 Miscellanea . 170 13 Related Work 174 13.1 Static Hybrid Strategies . 175 13.2 Eager Haskell . 180 13.3 Speculative Evaluation for Multiprocessor Parallelism . 182 13.4 Speculation for Uniprocessor Parallelism . 184 13.5 Models of Cost . 186 13.6 Profiling . 189 13.7 Debuggers for Non-Strict Languages . 190 13.8 Reducing Space Usage . 192 13.9 Other Approaches to Evaluation . 193 CONTENTS vii 14 Conclusions 195 A Proof that Meaning is Preserved 198 A.1 Evaluation Preserves Meaning . 198 A.2 Abortion Preserves Meaning . 201 B Proof that Costed Meaning is Preserved 204 B.1 Lemmas . 204 B.2 Proof of Soundness for Evaluation Rules . 205 B.3 Proof of Soundness for Abortion Rules . 207 C Proof that programWork predicts workDone 209 C.1 Preliminaries . 209 C.2 Proof for Evaluation Transitions . 210 C.3 Proof for Abortion Transitions . 213 D Example HsDebug Debugging Logs 214 CHAPTER 1 Introduction Avoiding work is sometimes a waste of time. Non-strict languages are elegant, but they are also slow. Optimistic Evaluation is an imple- mentation technique that makes them faster. It does this by using a mixture of Lazy Evaluation and Eager Evaluation, with the exact blend decided by an online profiler. 1.1 Lazy Evaluation Lazy Evaluation is like leaving the washing-up until later. If you are never going to use your plates again then you can save considerable time by not washing them up. In the extreme case you may even avoid washing up a plate that is so dirty that it would have taken an infinite amount of time to get clean. However, if you are going to need to use your plates again, then leaving the washing up until later will waste time. By the time you eventually need to use your plates, the food on them will have stuck fast to the plate and will take longer to wash off. You will also have to make space in your kitchen to hold all the washing up that you have not yet done. Non-strict languages aim to make life easier for programmers by removing the need for a programmer to decide if and when an expression should be evaluated. If a programmer were to write the following: let x = E in E0 1 2 Chapter 1. Introduction then a strict language such as C.
Details
-
File Typepdf
-
Upload Time-
-
Content LanguagesEnglish
-
Upload UserAnonymous/Not logged-in
-
File Pages244 Page
-
File Size-