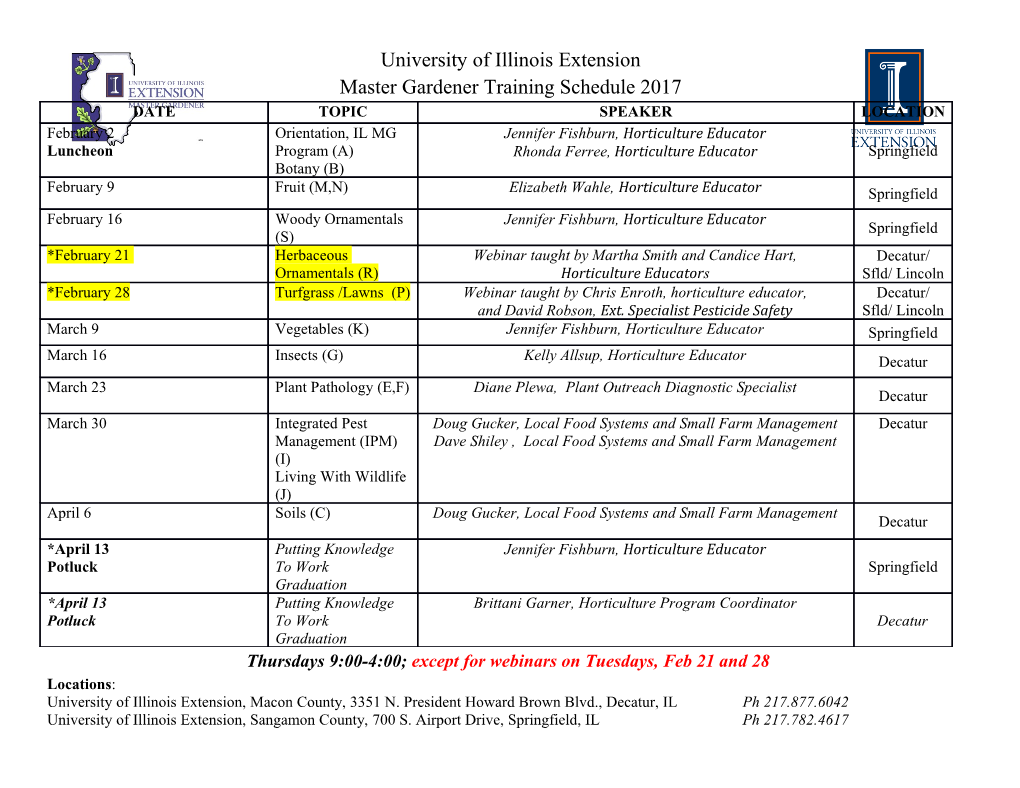
data Essay Towards a Contextual Approach to Data Quality Stefano Canali Institute of Philosophy, Leibniz University Hannover, Im Moore 21, 30167 Hannover, Germany; [email protected] Received: 31 July 2020; Accepted: 21 September 2020; Published: 25 September 2020 Abstract: In this commentary, I propose a framework for thinking about data quality in the context of scientific research. I start by analyzing conceptualizations of quality as a property of information, evidence and data and reviewing research in the philosophy of information, the philosophy of science and the philosophy of biomedicine. I identify a push for purpose dependency as one of the main results of this review. On this basis, I present a contextual approach to data quality in scientific research, whereby the quality of a dataset is dependent on the context of use of the dataset as much as the dataset itself. I exemplify the approach by discussing current critiques and debates of scientific quality, thus showcasing how data quality can be approached contextually. Keywords: research data management; scientific epistemology; data quality; FAIR; reproducibility crisis 1. Summary Determining the quality of scientific data is a task of key importance for any research project and involves considerations at conceptual, practical and methodological levels. The task has arguably become even more pressing in recent years, as a result of the ways in which the volume, variety, value, volatility, veracity and validity of scientific data have changed with the rise of data-intensive methods in the sciences [1]. At the start of the last decade, many commentators argued that these changes would bring dramatic shifts to the scientific method and would per se make science better, thanks to fully automated reasoning, more data-driven methods, less theorizing and more objectivity [2]. However, analyses of the use of data-intensive methods in the sciences have shown that the feasibility and benefits of these methods are not automatic results of these changes, but crucially rest upon the transparency, validity and quality of data practices [3]. As a consequence, there are currently various attempts at implementing guidelines to maintain and promote the quality of datasets, developing ways and tools to measure it and conceptualizing the notion of quality [4–6]. In this commentary, I want to focus on the latter line of research and discuss the following question: what are high-quality data? I propose a framework for data quality that suggests a contextual approach, whereby quality should be seen as a result of the context where a dataset is used and not only of the intrinsic features of the data. I develop this approach by integrating philosophical discussions on the quality of data, information and evidence. In Section2, I start by reviewing analyses of quality in different areas of philosophical research, particularly in the philosophy of information, the philosophy of science and philosophy of biomedicine. I identify and integrate shared results from this review and argue that these point towards a contextual approach, presenting the approach in Section3. I then discuss what the approach entails and how it can be used in practice, looking at current debates on quality in the scientific and philosophical literature (Section4). I conclude by summarizing the discussion of the commentary in Section5. Data 2020, 5, 90; doi:10.3390/data5040090 www.mdpi.com/journal/data Data 2020, 5, 90 2 of 10 2. Quality as a Property of Information, Evidence and Data Quality has been discussed in areas of philosophical work highly engaged with research practices and debates in the sciences [7]. In this context, I identify three main areas of research whose results are particularly significant for conceptualizations of quality and yet have only partially been applied to issues in data quality. I want to bring forth these results and their integration as important contributions for more general and interdisciplinary discussions on data quality. I identify and discuss research on quality as a property of three closely related notions: information, data and evidence. First, research on quality has traditionally focused on information quality, which became prominent in computer science in the 1990s. In this context, an influential line of research started to move beyond traditional interpretations of quality in terms of accuracy only, developing a multi-dimensional and purpose-dependent view whereby a piece of information is of high quality insofar as it is fit for a certain purpose [8]. This line of research has developed into two main approaches since the 1990s: by surveying opinions and definitions of academics and practices from an “empirical” point of view; and by studying the different dimensions of quality and interrelations between these from a theoretical and “ontological” perspective [9]. The empirical approach has expanded conceptualizations of information quality to include not only traditional dimensions such as accuracy, but also objectivity, completeness, relevance, security, access and timeliness; here, the goal has primarily been to categorize these dimensions, rather than to define them [10]. On the other hand, the goal of the ontological approach has been to understand how to connect different dimensions of information quality (such as those surveyed through the empirical approach [11]) and conceptualize and measure potential disconnections as errors [12]. These discussions have been picked up and analyzed in the area of research known as philosophy of information. According to Phyllis Illari and Luciano Floridi, computer science has not fully embraced the purpose-dependent approach to information quality in all of its implications and theoretical understandings of information quality are still in search of a way of applying the approach to concrete contexts [6] (p. 8). With these problems and goals in mind, Illari has suggested that information quality suffers from a rock-and-a-hard-place problem [13]. While information quality is defined as information that is fit for purpose, many still think that some aspects and dimensions of information quality should be independent of specific purposes (the rock). At the same time, there is a sense in which quality should make information fit for multiple—if not all—purposes: a piece of information that is fit for a specific purpose, but not for others, will not be considered of high quality (the hard place). As a way of going beyond the impasse, Illari has argued that we should classify information quality on the basis of a relational model, which links the different dimensions of quality to specific purposes and uses [13]. Therefore, Illari conceives of quality as a property of information that is highly dependent on its context, i.e., the specific uses, aims and purposes we want to employ a piece of information for. In other words, quality cannot be independent of fit for a specific purpose and cannot consist in a fit for any single purpose. I identify a similar push for the purpose-dependent and contextual approach in a second area of philosophical analyses, which have more specifically focused on the use of data in the context of scientific practice. The increasing volume and variety of data used in the sciences, with related and different levels of veracity, validity, volatility and value, have created a number of potential benefits as well as challenges for scientific epistemology [14]. Determining and assessing quality is one of the main challenges of data-intensive science because of the diversity of sources of data and integration practices, the often short “timespan” and relevance of data, the difficulties of providing quality assessments and evaluations in a timely manner and the overall lack of unified standards [4]. Partly as a result of these shifts, recently philosophers of science have expanded their focus on data as an important component of scientific epistemology [15]. In this context, some analyses have focused on the tools that are used to calibrate, standardize and assess the quality of data in the sciences. For instance, data quality assessment tools are often applied to clinical studies, in the form of scales or checklists about specific aspects of the study, with the goal of checking whether the study, e.g., makes use of Data 2020, 5, 90 3 of 10 specific statistical methods, sufficiently describes subject withdrawal, etc. According to Jacob Stegenga, there are two main issues affecting the use of these tools in the biomedical context: a poor level of inter-rating operability, i.e., different users of the tools achieve different instead of similar results; and a low level of inter-tool operability, i.e., different types of tools give different instead of similar results when assessing the same study [16]. Stegenga has argued that this can be conceptualized as a result of the underdetermination of the evidential significance of data: there is no uniquely correct way of estimating information quality and different results will always be obtained in relation to the context, users and type of study. I interpret these results in similar terms to the aforementioned analysis by Illari [13], as pointing to the crucial role that the context where data are analyzed and used plays in determination of its quality. Quality is not an intrinsic property of data that only depends on the characteristics of the data itself: quality will differ depending on contextual features, such as the tools used to assess quality, who uses them, their purposes, etc. Further support for this point comes from Sabina Leonelli’s studies of data practices—especially assessment methods—in the life sciences [17]. Leonelli has argued that existing approaches to data quality assessment mostly fail at delivering on their objectives or being actually used in standard practice, to the point that, currently, new and more recently developed technologies and techniques of data collection are used as unofficial markers for data quality. This leads to a problematic situation for the following reasons.
Details
-
File Typepdf
-
Upload Time-
-
Content LanguagesEnglish
-
Upload UserAnonymous/Not logged-in
-
File Pages10 Page
-
File Size-