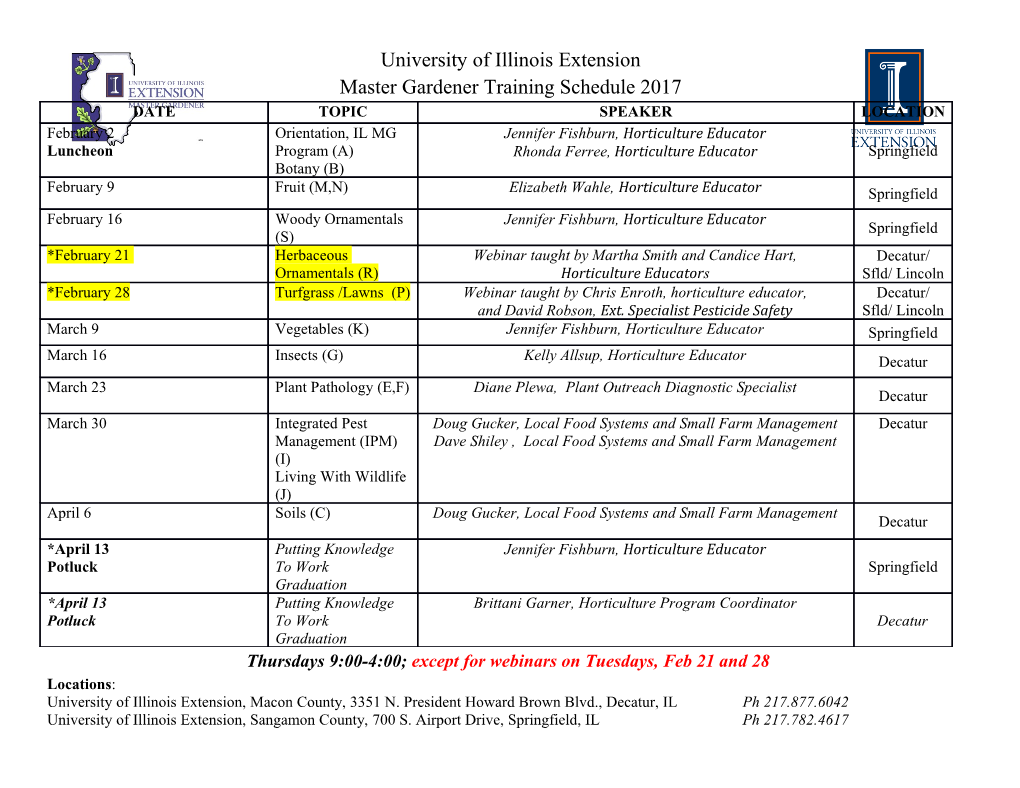
Two-Stage Least Squares Estimation of Average Causal Effectsin Models WithVariable TreatmentIntensity Joshua D. ANGRISTand Guido W. IMBENS* Two-stageleast squares (TSLS) is widelyused in econometricsto estimate parameters in systemsof linear simultaneous equations andto solve problems of omitted-var.ables biasin single-equationestimation. We showhere that TSLS canalso be usedto estimate theaverage causal effect of variable treatments such as drugdosage, hours of exam preparation, cigarette smoking, and yearsof schooling.The average causal effect in whichwe areinterested is a conditionalexpectation of the difference between the outcomes of thetreated and whatthese outcomes would have been in theabsence of treatment.Given mild regularity assumptions, the probabilitylimit of TSLS is a weightedaverage of per-unit average causal effects along the length of an appropriatelydefined causal responsefunction. The weightingfunction is illustratedin an empiricalexample based on therelationship between schooling and earnings. KEY WORDS: Instrumentalvariables; Rubin causal model; Schooling; Wages. 1. INTRODUCTION IV andTSLS estimators.Finally, the analysis used by Robins (1989,sec. 18) andRobins and Tsiatis (1991) tocorrect for Econometriciansand statisticians have developed a variety noncompliancein clinicaltrials is an applicationof instru- oftechniques for the estimation of systems of linear simul- mentalvariables to experimentaldata. taneousequations. The simplestand mostcommonly used Evaluationresearch in econometricsand applicationsof ofthese techniques isthe class of instrumental variables (IV) IV andTSLS inother fields typically rely on regressionmod- estimators,of which two-stage least squares (TSLS) is the els withconstant coefficients. The regressionapproach to mostimportant special case. IV and TSLS weredeveloped evaluationpostulates a hypothetical linear response function. in earlyresearch on simultaneousequations estimation (by In contrast,the statistical literature on evaluationhas been Wright[1928] and Theil[1958], among others), and both stronglyinfluenced by Rubin's (1974, 1977) model for causal estimatorsare now described in every econometrics textbook inferenceusing counterfactual outcomes. (The ideasbehind (e.g.,Theil 1971). These techniques are typically introduced thisframework date back to Neymanand Fisher; see Rubin as solutionsto the problem of "simultaneous equations bias." 1990for historical details.) In a methodologicalpaper related Moregenerally, however, IV providesa powerful and flexible to thisone (Imbensand Angrist1994), we discussthe use estimationstrategy that can be usedto tacklethe problem of IV to estimateaverage causal effects in Rubin'scausal ofomitted-variables bias in a widerange of single-equation model for binary treatments, as well as howto estimatethe regressionapplications, such as modelswith mismeasured sampling variance of IV estimatesof treatmenteffects. In regressors(Durbin 1954) and theestimation of treatment another recent paper (Angrist, Imbens, and Rubin1995), effectsin manpowertraining programs (Heckman and Hotz we presenta detailedanalysis of the conceptual issues and 1989;Heckman and Robb 1985). problemsthat arise when IV is usedto estimatethe average The use ofIV and TSLS to estimatetreatment effects is causaleffect of a binarytreatment inthe Rubin causal model, notlimited to econometrics. Permutt and Hebel (1989) used togetherwith a surveyof evaluation research in econometrics TSLS to estimatethe effect of maternalsmoking on birth and statistics. weight.Hearst, Newman, and Hulley's(1986) use of the In thisarticle, we show how TSLS canbe usedto estimate draftlottery to estimatethe effect of Vietnam-eramilitary averagecausal effects in a versionof Rubin's causal model serviceon civilianmortality is another epidemiological ap- thatallows for variable treatment intensity, multiple instru- plicationof IV. Angristand Krueger(1992) usedIV to es- ments,and covariates. In particular,we showthat TSLS ap- timatethe effect of children's age at schoolentry on their pliedto a causalmodel with variable treatment intensity and ultimateeducational attainment. The Powersand Swinton nonignorabletreatment assignment identifies a weighted av- (1984) "encouragementdesign" used to studythe effect of erageof per-unit treatment effects along the length of a causal testpreparation on graduaterecord examination (GRE) responsefunction. Our resultsdo nothinge on linearityof scores,discussed by Holland (1988), alsoleads naturally to therelationships between response variables, treatment in- tensities,and instruments. * JoshuaD. AngristisSenior Lecturer, Department ofEconomics, Hebrew A secondcontribution of thisarticle is to illustratethe University,Jerusalem 91095, Israel. Guido W. Imbens is Associate Professor, causal response weighting function in an empiricalexample Departmentof Economics,Harvard University, Cambridge, MA 02138. Theauthors thank Don Rubinfor raising the question that this article an- basedon thework by Angrist and Krueger (1991), usingIV swers,and seminar participants atthe Harvard-MIT Econometrics Workshop and TSLS to estimatethe effect of yearsof schoolingon andthe University ofWisconsin for helpful comments. Four referees and earnings.The weighting function in theapplication is ofin- an associateeditor also madehelpful comments. Financial support from theNational Science Foundation (SES-9122627) and the Nederlandse Or- ganisatieVoor Wetenschappelijk Onderzoek is alsogratefully acknowledged. ? 1995 AmericanStatistical Association Partof the article was written while the second author was visiting the Hebrew Journalof the AmericanStatistical Association UniversityDepartment ofEconomics. June 1995,Vol. 90, No. 430, Applicationsand Case Studies 431 432 Journal of the American Statistical Association, June 1995 terestbecause the relationship between schooling and earn- pulsoryattendance laws typically require students to enter ingsis one ofthe most important empirical regularities in schoolin thefall of the year in whichthey turn 6, butallow economics.More generally, the weighting formula can help studentsto dropout of school when they reach age 16.This researchersunderstand which observations are contributing induces a relationshipbetween quarter of birth and educa- to a particularestimate, and theformula provides a causal tionalattainment, because students born in thefirst quarter interpretationforsome of the simple estimators commonly of theyear enter school at an olderage thando students usedin appliedresearch. bornin later quarters. Students who enter school at an older agethus are allowed to drop out after having completed less 2. APPLICATION:THE EFFECT OF COMPULSORY schoolingthan students who enter school at a youngerage. SCHOOL ATTENDANCEON EARNINGS Ifstudents' quarter of birth is correlatedwith earnings solely becauseit is correlatedwith schooling, then it is an instru- Thetheory of human capital (Becker 1964) says that years mentfor schooling in an earningsequation. of schoolingcan be treatedmuch the same way as an in- One wayto convertthis idea intoan estimationstrategy vestmentin physicalcapital, yielding a rate of return some- is to comparethe education and earningsof people born in thinglike an interestrate. The empirical counterpart ofthis thefirst quarter to the education and earnings of people born theoryis the"human capital earnings function," a semilog- in a laterquarter, say the fourth. This leads to thesimplest arithmicregression of earnings on schoolingand otherco- possibleIV estimator:Wald's method of fitting straight lines variates.An exampleis thefollowing model for microdata: (Durbin1954). As an example,calculations underlying Wald Y = o + Xy+pS+,(+1 ) estimatesbased on a firstquarter/fourth quarter comparison formen are laid outin Table 1. PanelA ofthe table shows whereY is thelog of weekly earnings, yo is a constant,X1 is resultstabulated from data on thewages and earningsof a rowvector of covariates, yj a vectorof coefficients,S is menin the 1970 Census, and Panel B showsresults tabulated yearsof schooling, and thecoefficient p is theapproximate using data fromthe 1980Census. In bothdata sets,men percentagereturn to a yearof schooling.Equation ( 1) is bornin thefirst quarter earn slightly less and haveslightly sometimesaugmented with an equationdescribing how lessschooling than men born in the fourth quarter. The ratio schoolingis relatedto thecovariates, X1, and additional co- ofdifferences inearnings to differences inschooling generates variates,X2: a Waldestimate of the return to schoolingof 5.3% using the 1970Census and 8.9%using the 1980 Census. S= 60 +Xlbl +X262 +q7 (2) Durbin(1954) showedthat under the null hypothesis that Econometricianstypically assume that a semilogarithmicthe OLS estimateis consistent,the sampling variance of the responsefunction for earnings is a reasonablygood approx- imationto the true earnings function. Ordinary least squares (OLS) appliedto ( 1), however,may lead to biased estimates Table1. CompulsorySchool Attendance of if p even thetrue response function is linear.The reason (1) (2) (3) is thatschooling is determinedby individual choices under Bornin Bornin Difference constraints.For example,the literature on schoolingand 1stquarter 4thquarter (std.error) of of - earningshas devoted considerable attention to theproblem year year (1) (2) of "abilitybias" in estimatesof the economicreturn to PanelA: WaldEstimates for 1970 Census-Men Born1920-1929a schooling.This is a formof omitted-variables biasthat would In(weekly wage)
Details
-
File Typepdf
-
Upload Time-
-
Content LanguagesEnglish
-
Upload UserAnonymous/Not logged-in
-
File Pages12 Page
-
File Size-