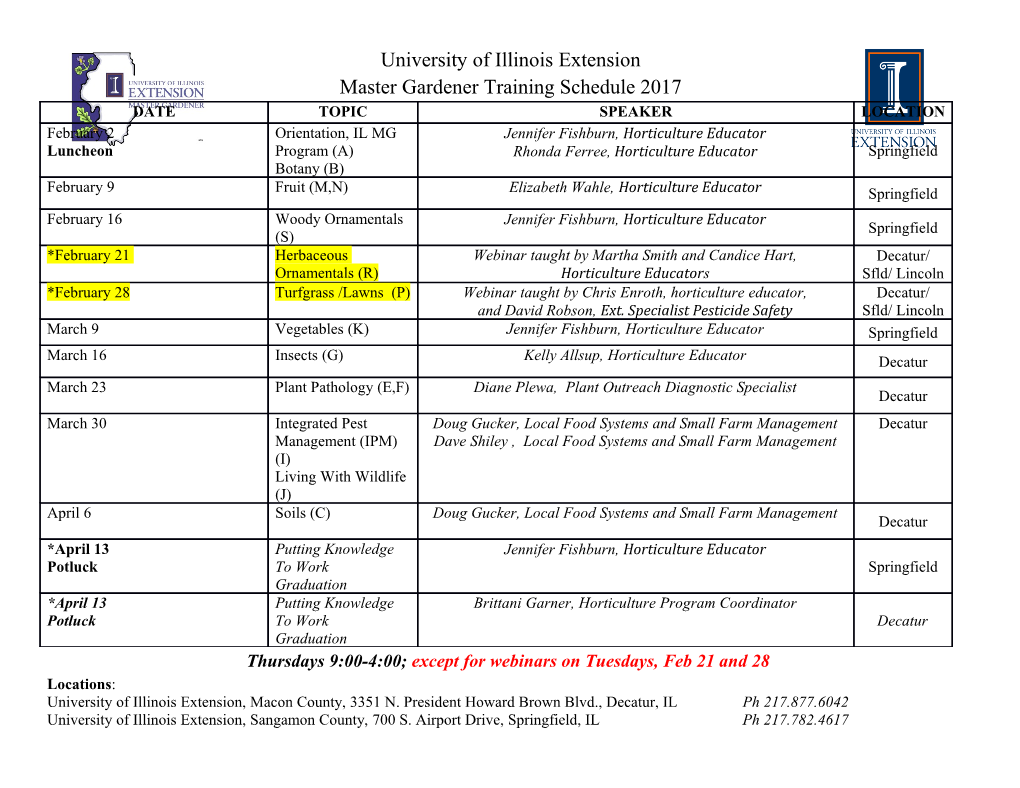
This article was downloaded by: [210.32.144.204] On: 27 January 2021, At: 17:31 Publisher: Institute for Operations Research and the Management Sciences (INFORMS) INFORMS is located in Maryland, USA Management Science Publication details, including instructions for authors and subscription information: http://pubsonline.informs.org Testing at the Source: Analytics-Enabled Risk-Based Sampling of Food Supply Chains in China Cangyu Jin, Retsef Levi, Qiao Liang, Nicholas Renegar, Stacy Springs, Jiehong Zhou, Weihua Zhou To cite this article: Cangyu Jin, Retsef Levi, Qiao Liang, Nicholas Renegar, Stacy Springs, Jiehong Zhou, Weihua Zhou (2021) Testing at the Source: Analytics-Enabled Risk-Based Sampling of Food Supply Chains in China. Management Science Published online in Articles in Advance 22 Jan 2021 . https://doi.org/10.1287/mnsc.2020.3839 Full terms and conditions of use: https://pubsonline.informs.org/Publications/Librarians-Portal/PubsOnLine-Terms-and- Conditions This article may be used only for the purposes of research, teaching, and/or private study. Commercial use or systematic downloading (by robots or other automatic processes) is prohibited without explicit Publisher approval, unless otherwise noted. For more information, contact [email protected]. The Publisher does not warrant or guarantee the article’s accuracy, completeness, merchantability, fitness for a particular purpose, or non-infringement. Descriptions of, or references to, products or publications, or inclusion of an advertisement in this article, neither constitutes nor implies a guarantee, endorsement, or support of claims made of that product, publication, or service. Copyright © 2021, INFORMS Please scroll down for article—it is on subsequent pages With 12,500 members from nearly 90 countries, INFORMS is the largest international association of operations research (O.R.) and analytics professionals and students. INFORMS provides unique networking and learning opportunities for individual professionals, and organizations of all types and sizes, to better understand and use O.R. and analytics tools and methods to transform strategic visions and achieve better outcomes. For more information on INFORMS, its publications, membership, or meetings visit http://www.informs.org MANAGEMENT SCIENCE Articles in Advance, pp. 1–12 http://pubsonline.informs.org/journal/mnsc ISSN 0025-1909 (print), ISSN 1526-5501 (online) Testing at the Source: Analytics-Enabled Risk-Based Sampling of Food Supply Chains in China Cangyu Jin,a Retsef Levi,b Qiao Liang,a Nicholas Renegar,c Stacy Springs,d Jiehong Zhou,a Weihua Zhoue a China Academy for Rural Development, School of Public Affairs, Zhejiang University, Hangzhou 310058, China; b Sloan School of Management, Massachusetts Institute of Technology, Cambridge, Massachusetts 02139; c Operations Research Center, Massachusetts Institute of Technology, Cambridge, Massachusetts 02139; d Center for Biomedical Innovation, Massachusetts Institute of Technology, Cambridge, Massachusetts 02139; e School of Management, Zhejiang University, Hangzhou 310058, China Contact: [email protected], https://orcid.org/0000-0002-9558-0428 (CJ); [email protected], https://orcid.org/0000-0002-1994-4875 (RL); [email protected], https://orcid.org/0000-0001-7473-7863 (QL); [email protected], https://orcid.org/0000-0002-5222-073X (NR); [email protected], https://orcid.org/0000-0003-2133-5689 (SS); [email protected], https://orcid.org/0000-0002-1967-780X (JZ); [email protected], https://orcid.org/0000-0002-1056-7612 (WZ) Received: March 27, 2020 Abstract. This paper illustrates how supply chain (SC) analytics could provide strategic Revised: July 14, 2020 and operational insights to evaluate the risk-based allocation of regulatory resources in Accepted: July 20, 2020 food SCs, for management of food safety and adulteration risks. This paper leverages data Published Online in Articles in Advance: on 89,970 tests of aquatic products extracted from a self-constructed data set of 2.6 million January 22, 2021 food safety tests conducted by the China Food and Drug Administration (CFDA) orga- https://doi.org/10.1287/mnsc.2020.3839 nizations. The integrated and structured data set is used to conduct innovative analysis that identifies the sources of adulteration risks in China’s food SCs and contrasts them with the Copyright: © 2021 INFORMS current test resource allocations of the CFDA. The analysis highlights multiple strategic insights. Particularly, it suggests potential gaps in the current CFDA testing allocation by SC location, which is heavily focused on retail and supermarkets. Instead, the analysis indicates that high-risk parts of the SC, such as wholesale and wet markets, are under- sampled. Additionally, the paper highlights the impact that SC analytics could have on policy-level operational decision making to regulate food SCs and manage food safety. The hope is that the paper will stimulate the interest of academics with expertise in these areas to conduct more work in this important application domain. History: Accepted by Charles Corbett, operations management. Funding: The work of C. Jin and N. Renegar was partially supported by the Walmart Foundation [Agreement dated 10/17/2016]. Supplemental Material: The data files and online appendix are available at https://doi.org/10.1287/mnsc.2020.3839. Keywords: food safety • supply chain • big data • analytics 1. Introduction melamine incident, which caused multiple deaths Food safety and food adulteration are widespread and more than 50,000 babies to be hospitalized with problems that challenge regulatory bodies, the food seriousinjuriesbecauseofbabyformulacontami- industry, and consumers around the world. Manag- nated with melamine through the dairy SC in China.1 ing food safety risks, such as foodborne illness out- This paper provides a first of its kind analysis to breaks, or intentional and economically motivated illustrate how data-driven SC analytics can evaluate adulteration (EMA) of food products is particularly risk-based monitoring of food SCs to potentially difficult because of the size, complexity, and opaque- identify gaps in the application of scarce regulatory ness of food supply chains (SCs). This challenge is resources. In particular, the paper focuses on China underscored by the relatively scarce resources avail- and leverages a unique, self-constructed data set of able to regulate and inspect these SCs. For example, 2.6 million food safety tests collected from 60,000 files each year the U.S. Food and Drug Administration (U.S. on 247 China Food and Drug Administration (CFDA) FDA) is able to sample only about 1%–2% of over websites. This includes 1.8 million tests between 2014 40 million imported food product lines (Racino 2011). and 2018, conducted in geographies where the data China also faces major challenges in regulating its set is thought to comprehensively cover all public food system and mitigating food safety and EMA postings of the CFDA. Data on 89,970 tests of aquatic risks, particularly because of extremely disaggregated products are extracted from this larger data set, in and complex food SCs. Indeed, China, like other order to provide both strategic and operational in- countries, is experiencing major incidents of food sights that could potentially inform risk-based sam- adulteration. Perhaps the most notable case is the pling of food SCs in China (see Definition 1). 1 Jin et al.: Analytics-Enabled Risk-Based Sampling of Food Supply Chains in China 2 Management Science, Articles in Advance, pp. 1–12, © 2021 INFORMS Definition 1. Risk-based sampling involves the use of category with many incidents of adulteration (Fox data (e.g., historical sampling results) to identify points et al. 2018). China is also the largest producer, ex- for testing (e.g., product categories, specific products, porter, and consumer of aquatic products worldwide SC locations, companies) that are more likely to expose (Xia 2019). Additionally, typical to China, this SC is problems and their respective source. also extremely complex and disaggregated, making This definition is analogous to risk-based approaches risk-based management more important. in healthcare, where qualitative and quantitative indi- TheSCrisksourceanalysisconsiders89,970CFDA cators are used to predict risk and considered as an input tests of aquatic products. Each failed test that detected fi for intervention decisions (Iezzoni 2012). This is also speci c adulterants is associated with the SC source consistent with the food sampling approach recently (farming, environment, manufacturing, circulation) proposed by the China State Council, which plans to that was most likely to introduce the adulterant. The allocate more resources to high-risk product cate- association is done based on expertise, technical and gories and companies (Xinhua News Agency 2019). economic analysis of the underlying motivation to This paper provides a detailed analysis of the SC add the adulterants to the food, and where it would sources introducing detected adulterants in tests con- be most “likely” added. The result is an aggregated ducted by the CFDA. This provides strategic insights analysis of the sources of risk in the SC. Although the as to what are the high-risk SC locations (e.g., manu- risk source analysis in this paper is focused on the facturers) that require more regulatory attention. This is freshwater aquatic SC in China, the insights are likely called risk-based sampling by SC location. Additionally, to hold more generally, and a framework
Details
-
File Typepdf
-
Upload Time-
-
Content LanguagesEnglish
-
Upload UserAnonymous/Not logged-in
-
File Pages13 Page
-
File Size-