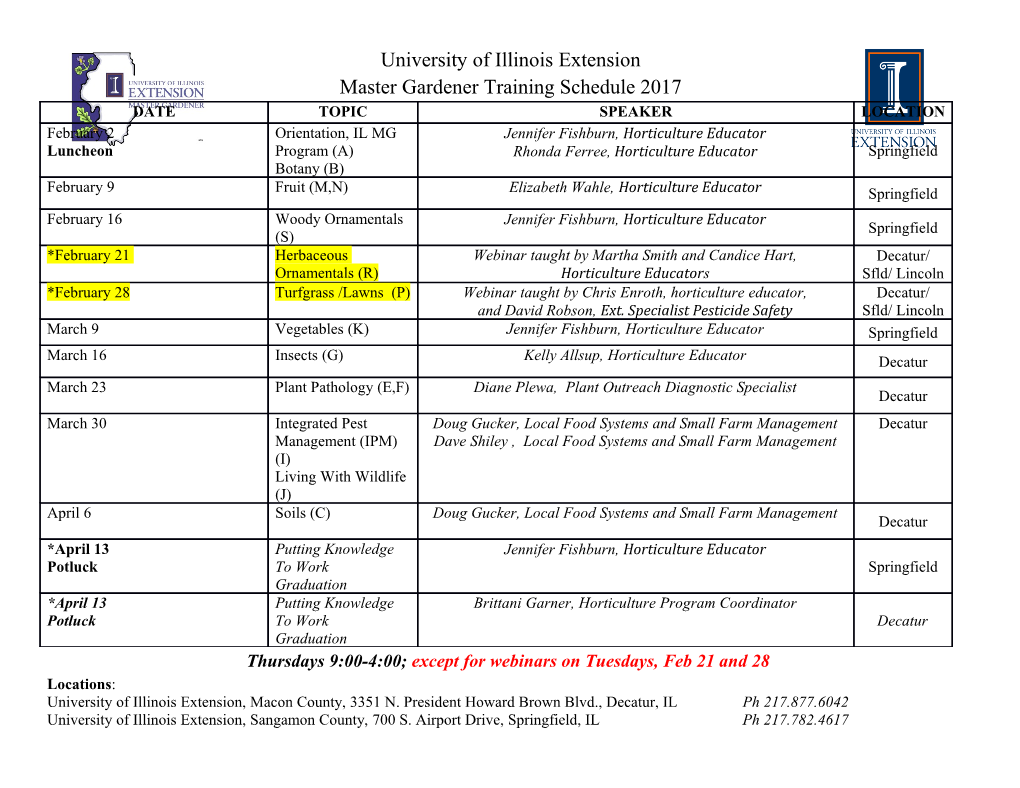
Donsker and Glivenko-Cantelli theorems for a class of processes generalizing the empirical process Davit Varron To cite this version: Davit Varron. Donsker and Glivenko-Cantelli theorems for a class of processes generalizing the empiri- cal process. Electronic Journal of Statistics , Shaker Heights, OH : Institute of Mathematical Statistics, 2014, pp.2301-2325. hal-01158050 HAL Id: hal-01158050 https://hal.archives-ouvertes.fr/hal-01158050 Submitted on 31 Aug 2016 HAL is a multi-disciplinary open access L’archive ouverte pluridisciplinaire HAL, est archive for the deposit and dissemination of sci- destinée au dépôt et à la diffusion de documents entific research documents, whether they are pub- scientifiques de niveau recherche, publiés ou non, lished or not. The documents may come from émanant des établissements d’enseignement et de teaching and research institutions in France or recherche français ou étrangers, des laboratoires abroad, or from public or private research centers. publics ou privés. Electronic Journal of Statistics ISSN: 1935-7524 arXiv: math.PR/0000000 Donsker and Glivenko-Cantelli theorems for a class of processes generalizing the empirical process Davit Varron Laboratoire de mathématiques de Besançon, UMR CNRS 6623, Université de Franche-Comté e-mail: [email protected] Abstract: We establish a Glivenko-Cantelli and a Donsker theorem for a class of random discrete measures which generalize the empirical measure, under conditions on uniform entropy numbers that are common in empirical pro- cesses theory. Some illustrative applications in nonparametric Bayesian theory and randomly sized sampling are provided. Primary 60F17, 60G57, 62F15. Keywords and phrases: Empirical processes, Random measures, Bayesian non- parametrics. Contents 1 Introduction.................................1 2 Main results.................................3 3 Some applications of Theorems1 and2.................6 3.1 Randomly sized sampling with increasing trust..........7 3.2 Application to sequences of normalized homogenous completely random measures...........................9 3.3 Stick breaking random measures.................. 12 3.4 A Bernstein-von Mises phenomenon under for sequences of Dirichlet processes priors.....................jj · jjF 16 3.5 A discussion on Gibbs-type priors................. 17 4 Proofs..................................... 18 References.................................... 24 1. Introduction The asymptotic properties of empirical processes indexed by functions have been intensively studied during the past decades (see, e.g., Van der Vaart and Wellner[1996] or Dudley[1999] for self-contained, comprehensive books on the topic). Among those results, one of the most used in statistical applications is that a class admiting a finite uniform entropy integral and a square inte- grable envelope is Donsker (Koltchinskii[1981]). In this note, we will show that 1 imsart-ejs ver. 2007/12/10 file: "Convex EJS final".tex date: October 28, 2014 D. Varron/Limit theorems for a class generalizing the empirical process 2 this structural condition is strong enough to carry a Donsker and a Glivenko- Cantelli theorem over a wider class of processes which we shall describe at N once. For p = (pi)i 1 R and r > 0, we shall write the (possibly infinite) value ≥ 2 X 1=r r p r:= pi : (1) jj jj j j i 1 ≥ Let (Ω; ; P) be a complete probability space (in the sense that every P- negligibleA set belongs to ), and let A n N o S := p = (pi)i 1 [0; + [ ; p 1< ; (2) ≥ 2 1 jj jj 1 be the cone of positive summable sequences, which will be endowed with the product Borel σ-algebra (denoted by Bor). Consider a sequence of S-indexed collections of probability measures Pn;p on a measurable space X; . n 1;p S ≥ 2 X For fixed n, consider a random variable β = (βi;n)i 1 from (Ω; ; P) to (S; Bor) n ≥ A and a sequence Yn = Yi;n of random variables from (Ω; ; P) to X; , for i 1 ≥ N A X which the conditional law given βn = p is Pn⊗;p (we also make the assumption that those conditional laws properly define a Markov kernel). We then define the random element X Prn := βi;nδYi;n ; (3) i 1 ≥ which is a random probability measure in the sense that Prn X 1 and Prn A ≡ is Borel measurable for each A . Obtaining asymptotic results (as n ) 2 X ! 1 for Prn is of interest for several reasons. The first of them is that Prn general- izes the usual empirical measure, and also related objects from the bootstrap theory, such as the empirical bootstrap, or more generally, the exchangeable bootstrap empirical measure (see [Van der Vaart and Wellner, 1996, Section 3.6.2]). Our main motivation to consider such a generalization comes from the second reason : these types of random measures play a role in the modeling of almost surely discrete priors, such as stick breaking priors (including the two parameter Poisson-Dirichlet process) or, more generally, species sampling priors, including the normalized homogenous completely random measures. For an overview of all the previously cited types of random measures, see, e.g., [Hjort et al., 2010, Chapter 3]. In addition, there are several situations where objects such as (3) do also appear in the posterior distributions or posterior expected values of some almost surely discrete priors (see §3 in the sequel). As usual in empirical processes theory, we shall write, for a probability measure P and an integrable function f : Z P( f ):= f dP; (4) X and we shall adopt the same notation Pr( f ) when Pr is a random probability measure, in which case Pr( f ) has to be understood as a random variable. As imsart-ejs ver. 2007/12/10 file: "Convex EJS final".tex date: October 28, 2014 D. Varron/Limit theorems for a class generalizing the empirical process 3 mentioned earlier, we will state a Glivenko-Cantelli and a Donsker result for empirical-like processes indexed by a class of (Borel) functions . When is not uniformly bounded, the definition of such objects requiresF additionalF care on the structure of . Throughout this article, we will assume that is F F pointwise separable relatively to a countable subclass 0 (see, e.g., Van der Vaart and Wellner[1996, p. 110]). We will denote by ( ) theF space of real bounded functions on that are continuous with respectB F to the topology spanned by n F o the maps f f (x); x . We shall also denote the usual sup norm on ( ) ! 2 X B F := sup ( f ) : jj jjF f j j 2F n o We will denote by F the envelope function x sup f (x); f 0 1. For ! 2 F _ > 0; r > 0 and a probability measure Q, we shall write N , ; Q r for the F jj · jj ; (possibly infinite) minimal number of balls with radius needed to cover , r F using the usual L (Q) norm. We shall also write `1( ) ( ) for the space of F ⊃ B F all real bounded functions on . For r > 0, we define the space ;r as follows : F EF a map Ψ : Ω ( ) belongs to ;r if and only if Ψ( f ) defines a Borel random !B F EF P r E r variable on (Ω; ; ) for each f , and if Ψ ;r:= Ψ < . Under A 2 F jjj jjjF jj jjF 1 the assumption that E F(Y n) < for all n, it is possible to define the process 1; 1 X Gn( ): f βi n f (Yi n) E f (Yi n) β · ! ; ; − ; j n i 1 ≥ as the limit, as k , of the truncated sequence ! 1 Xk k G ( ): f βi n f (Yi n) E f (Yi n) β n · ! ; ; − ; j n i=1 in the Banach space ;1; . Also note that this limit also holds in ;r EF jjj · jjjF EF r for each r 1 such that E F(Y1;n) < . Note that the measurability of the k ≥ 1 Gn is not completely immediate. The corresponding proof can be found at thejj beginningjjF of §4. 2. Main results We state here our first result, of Glivenko-Cantelli type. Theorem 1. Assume that βn 1= 1 almost surely for all n, and βn 2 0 in probability. Suppose that jj jj jj jj ! 1 lim lim E F(Y1;n) F(Y n) M = 0: (5) M n f 1; ≥ g !1 !1 imsart-ejs ver. 2007/12/10 file: "Convex EJS final".tex date: October 28, 2014 D. Varron/Limit theorems for a class generalizing the empirical process 4 Also assume that, for each > 0 and M > 0, we have, as n : ! 1 2 log N , M; P( Y ) 1 = oP βn 2− ; (6) F jj · jj βn; n ; jj jj n o where, M := f 1 F M ; f and F f ≤ g 2 F X N P(p; y):= piδy ; for p S; y X : (7) i 2 2 i 1 ≥ Then E Gn 0: (8) jj jjF ! Remark 2.1. 1 Choosing βi;n := n− when i n and 0 otherwise, leads to the usual Glivenko- Cantelli theorem under random≤ entropy conditions (see, e.g., Van der Vaart and Wellner[1996, p.123]), except for the almost sure counterpart of (8). Indeed, that almost sure convergence deeply relies on a reverse submartingale structure, which is not guaranteed under the general conditions of Theorem1. Our second result is a Donsker type Theorem. Since, for fixed n, the Yi;n are only conditionally independent, such a result will not involve the Gaussian analogues of the Gn, but mixtures Wn of the Pn;βn Brownian bridges by βn, for which a rigorous definition resuires additional care. To properly define them, p we proceed as follows : for p 1 and f := ( f1;:::; fp) and p S, writing f ≥ 2 F 2 Qn;p for the centered Gaussian distribution with variance covariance matrix f h i Σn;p := Pn;p ( fj Pn;p( fj))( fj0 Pn;p( fj0 )) ; (j;j ) 1;:::;p 2 − − 0 2f g we set, for each Borel set A Rp : ⊂ Qf (A):= E Qf (A) : n n;βn Kolmogorov’s extension theorem ensures the existence of a probability measure Pn0 on Ω0 := RF , endowed with its (Pn0 -completed) product Borel σ-algebra 0, n f p o X which is compatible with the system Qn; f ; p 1 .
Details
-
File Typepdf
-
Upload Time-
-
Content LanguagesEnglish
-
Upload UserAnonymous/Not logged-in
-
File Pages27 Page
-
File Size-