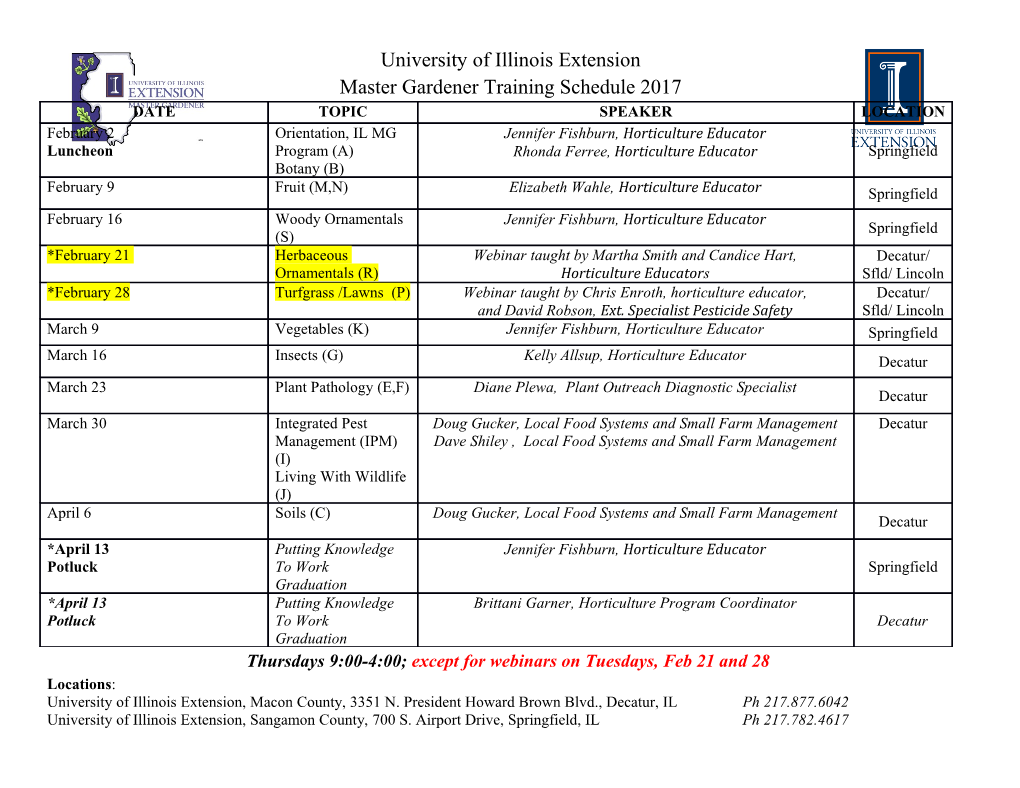
Causal Inference in Observational Studies with Complex Design: Multiple Arms, Complex Sampling and Intervention Effects DISSERTATION Presented in Partial Fulfillment of the Requirements for the Degree Doctor of Philosophy in the Graduate School of The Ohio State University By Giovanni Nattino, M.S. Graduate Program in Biostatistics The Ohio State University 2019 Dissertation Committee: Dr. Bo Lu, Advisor Dr. Stanley Lemeshow, Co-Advisor Dr. Eloise Kaizar © Copyright by Giovanni Nattino 2019 Abstract Observational studies are major data sources to infer causal relationships. When using observational data to estimate causal effects, researchers must consider appro- priate statistical methodology to account for the non-random allocation of the units to the treatment groups. Such methodology is well-established when the research question involves two treatment groups and results do not need to be generalized to the population from which the study sample has been selected. Relatively few studies have focused on research questions that do not fit into this framework. The goal of this work is to introduce statistical methods to perform causal inference in complex designs. First, I introduce a matching design for estimating treatment effects in the presence of multiple treatment groups. I devise a novel matching algorithm, generating samples that are well-balanced with respect to pre-treatment variables, and discuss the post-matching statistical analyses. Second, I focus on the generaliza- tion of causal effects to the population level, specifically when the sample selection is based on complex survey designs. I discuss the extension of the propensity score methodology to survey data, describe a weighted estimator for the common two-stage cluster sample and study its asymptotic properties. Third, I consider the estimation of population intervention effects, which evaluate the impact of realistic changes in the distribution of the treatment in a cohort. I describe estimators for upper and lower bounds of effects of this type, highlighting the implications for policy makers. For each of these three areas of causal inference, I use Monte Carlo simulations to ii assess the reliability of the proposed methods and compare them with competing approaches. The new methods are illustrated with real-data applications. Finally, I discuss limitations and aspects requiring further work. iii Acknowledgments First of all, I would like to express my sincere gratitude to my advisors. Thanks to Dr. Stan Lemeshow, who has been the catalyst of this incredible journey. Without you, I would not be where I am now. I am grateful for your unconditional help, which often went beyond the university walls, and countless advices. An equal thanks goes to Dr. Bo Lu, who introduced me to the world of causal inference. Thank you for your guidance and trust, which simultaneously directed me to the finish line and left me space to set my own pace. Thanks for all the pragmatic suggestions and for helping me navigating the statistical conferences I have been fortunate to attend. Thanks to all the staff of the Government Resource Center, in particular to Lorin Ranbom and Colin Odden, for the continuous support and for the invaluable oppor- tunity of continuously working on the Infant Mortality Research Partnership project. A special thank you to all the researchers I was fortunate to meet within this project. Thank you \Task 4" members, especially to Dr. Pat and Steve Gabbe and Dr. Court- ney Hebert. Your enthusiasm and genuine devotion to impact on the well-being of our society have truly inspired me. I would also like to thank Dr. Henry Xiang and Dr. Junxin Shi, from Nationwide Children's Hospital, for their expert advice and the help with the trauma data, which motivated part of this work. Thanks to all the faculties and students I have met during my time at the Ohio State University. In particular, I would like to thank Dr. Elly Kaizar, for your iv valuable feedback on my work. I am grateful to Dr. Matt Pratola and Hengrui Luo and to Dr. Mike Pennell. Even though the results of our collaborations do not appear in these pages, working with you was a truly stimulating, refreshing and enjoyable experience. A special thanks also to Dr. Amy Ferketich and Dr. Mario Peruggia, for your friendly advice and for being my \Little Italy" in Columbus. I would like to thank the researchers of the Laboratory of Clinical Epidemiology at the Mario Negri Institute for Pharmacological Research, in Italy, where I developed my interests in research and in biostatistics. Thank you all, especially to Dr. Guido Bertolini, for helping me embarking on this journey. Thanks to all the friends who have been my Columbus family in these years. In particular, thank you Sebastian, Guilherme, Aziz, J´ulia,Armand, Shuyuan, Jason, Natalia, Jafar, Juli´an,Alejandro and Andreas. Thanks for all the dinners together, the Friday night gatherings, the endless barbecues, the bike rides, the rock climbing sessions, the racquetball and disc golf games. You will be missed. A special thanks to my parents, Daniela and Beppe, and my brothers, Francesco and Stefano. If I am where I am, this is because of your education, encouragement and love. Finally, a profound thank you to my fianc´ee,Melissa. You understood the impor- tance of this goal for me, despite the time together that I had to sacrifice along the way. Thanks for your patience and heartening words. I could not have asked for a better travel companion. v Vita 1987 . .Born in Lecco (LC), Italy Education 2009 . .B.S. Applied Mathematics, University of Milan, Milan, Italy 2011 . .M.S. Applied Mathematics, University of Milan, Milan, Italy 2014 . .Post-graduate certificate in Biomedical Research, Istituto di Ricerche Farma- cologiche Mario Negri IRCCS, Ranica (BG), Italy Professional Experience 2011-2015 . Research Associate, Laboratory of Clinical Epidemiology, Istituto di Ricerche Farmacologiche Mario Negri IRCCS, Ranica (BG), Italy 2016-2019 . Graduate Research Associate, Divi- sion of Biostatistics, College of Pub- lic Health, The Ohio State University, Columbus, Ohio 2017-2019 . Graduate Research Associate, Ohio Colleges of Medicine Government Re- source Center, The Ohio State Univer- sity Wexner Medical Center, Colum- bus, Ohio vi Publications 1. Giovanni Nattino, Michael L Pennell, and Stanley Lemeshow. Assessing the goodness of fit of logistic regression models in large samples: a modification of the Hosmer-Lemeshow test. Submitted to Biometrics, 2019. 2. Giovanni Nattino, Bo Lu, Junxin Shi, Stanley Lemeshow, and Henry Xiang. Triplet matching for estimating causal effects with three treatment arms: a comparative study of mortality by trauma center level. Submitted to Journal of the American Statistical Association, 2019. 3. Courtney L Hebert, Giovanni Nattino, Steven G Gabbe, Patricia T Gabbe, Jason Benedict, Gary Phillips, and Stanley Lemeshow. A predictive model for very preterm birth: developing a point of care tool. Submitted to American Journal of Obstetrics and Gynecology, 2019. 4. Erinn M Hade, Giovanni Nattino, Heather A Frey, and Bo Lu. Propensity Score Matching for Treatment Delay Effects with Observational Survival Data. Submitted to Statistical Methods in Medical Research, 2019. 5. Giovanni Nattino and Bo Lu. Model assisted sensitivity analyses for hidden bias with binary outcomes. Biometrics, 74: 1141{1149, 2018. 6. Stefano Skurzak, Greta Carrara, Carlotta Rossi, Giovanni Nattino, Daniele Crespi, Michele Giardino, and Guido Bertolini. Cirrhotic patients admitted to the icu for medical reasons: Analysis of 5506 patients admitted to 286 icus in 8years. Journal of Critical Care, 45: 220{228, 2018. 7. Guido Bertolini, Giovanni Nattino, Carlo Tascini, Daniele Poole, Bruno Viaggi, Greta Carrara, Carlotta Rossi, Daniele Crespi, Matteo Mondini, Martin Langer, Gian Maria Rossolini, and Paolo Malacarne. Mortality attributable to different kleb- siella susceptibility patterns and to the coverage of empirical antibiotic therapy: a cohort study on patients admitted to the ICU with infection. Intensive Care Medicine, 44(10): 1709{1719, 2018. 8. Giovanni Nattino, Stanley Lemeshow, Gary Phillips, Stefano Finazzi, and Guido Bertolini. Assessing the calibration of dichotomous outcome models with the calibra- tion belt. Stata Journal, 17(4): 1003{1014, 2017. 9. Daniele Poole, Stefano Finazzi, Giovanni Nattino, Danilo Radrizzani, Giuseppe Gristina, Paolo Malacarne, Sergio Livigni, and Guido Bertolini. The prognostic im- portance of chronic end-stage diseases in geriatric patients admitted to 163 italian ICUs. Minerva Anestesiologica, 83: 1283{1293, 2017. vii 10. Giovanni Nattino, Stefano Finazzi, and Guido Bertolini. A new test and graphical tool to assess the goodness of fit of logistic regression models. Statistics in Medicine, 35(5): 709{720, 2016. 11. Daniele Poole, Giovanni Nattino, and Guido Bertolini. Overoptimism in the interpretation of statistics. Intensive Care Medicine, 40(12): 1927{1929, 2014. 12. Giovanni Nattino, Stefano Finazzi, and Guido Bertolini. Comments on `Graphical assessment of internal and external calibration of logistic regression models by using loess smoothers' by Peter C. Austin and Ewout W. Steyerberg. Statistics in Medicine, 33(15): 2696{2698, 2014. 13. Giovanni Nattino, Stefano Finazzi, and Guido Bertolini. A new calibration test and a reappraisal of the calibration belt for the assessment of prediction models based on dichotomous outcomes. Statistics in Medicine, 33(14): 2390{2407, 2014. 14. Nicola Latronico, Giovanni Nattino, Bruno Guarneri,
Details
-
File Typepdf
-
Upload Time-
-
Content LanguagesEnglish
-
Upload UserAnonymous/Not logged-in
-
File Pages169 Page
-
File Size-