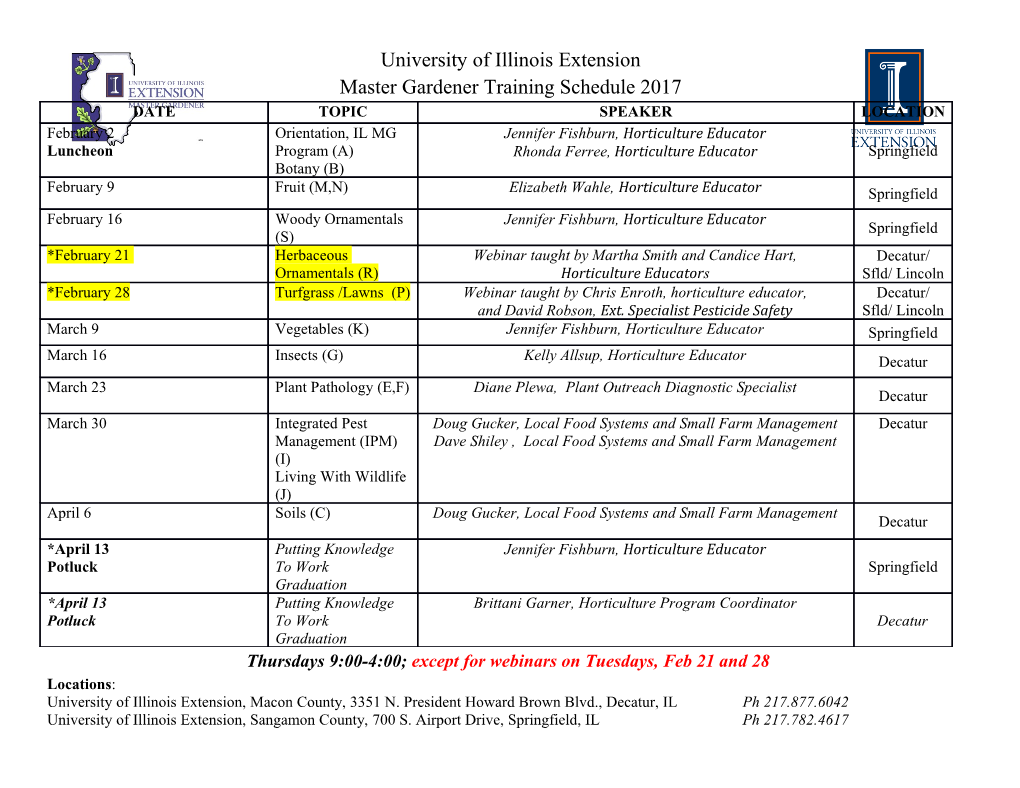
www.nature.com/scientificreports OPEN Evolution of disease transmission during the COVID‑19 pandemic: patterns and determinants Jie Zhu & Blanca Gallego* Epidemic models are being used by governments to inform public health strategies to reduce the spread of SARS‑CoV‑2. They simulate potential scenarios by manipulating model parameters that control processes of disease transmission and recovery. However, the validity of these parameters is challenged by the uncertainty of the impact of public health interventions on disease transmission, and the forecasting accuracy of these models is rarely investigated during an outbreak. We ftted a stochastic transmission model on reported cases, recoveries and deaths associated with SARS‑CoV‑2 infection across 101 countries. The dynamics of disease transmission was represented in terms of the daily efective reproduction number ( Rt ). The relationship between public health interventions and Rt was explored, frstly using a hierarchical clustering algorithm on initial Rt patterns, and secondly computing the time‑lagged cross correlation among the daily number of policies implemented, Rt , and daily incidence counts in subsequent months. The impact of updating Rt every time a prediction is made on the forecasting accuracy of the model was investigated. We identifed 5 groups of countries with distinct transmission patterns during the frst 6 months of the pandemic. Early adoption of social distancing measures and a shorter gap between interventions were associated with a reduction on the duration of outbreaks. The lagged correlation analysis revealed that increased policy volume was associated with lower future Rt (75 days lag), while a lower Rt was associated with lower future policy volume (102 days lag). Lastly, the outbreak prediction accuracy of the model using dynamically updated Rt produced an average AUROC of 0.72 (0.708, 0.723) compared to 0.56 (0.555, 0.568) when Rt was kept constant. Monitoring the evolution of Rt during an epidemic is an important complementary piece of information to reported daily counts, recoveries and deaths, since it provides an early signal of the efcacy of containment measures. Using updated Rt values produces signifcantly better predictions of future outbreaks. Our results found variation in the efect of early public health interventions on the evolution of Rt over time and across countries, which could not be explained solely by the timing and number of the adopted interventions. Mathematical and computational models of disease outbreaks are used to generate knowledge about the biologi- cal, behavioral and environmental processes of disease transmission, as well as to forecast disease progression. Public health responders rely on insights provided by these models to guide disease control strategies1. As an example, in mid March 2020, the British government changed its SARS-CoV-2 response policy following a brief on simulation results from Ferguson et al.2, which indicated an unacceptable forecasted number of deaths in the absence of more stringent control measures. Diferent models have been applied to model the spatial and temporal dynamics of SARS-CoV-2 transmis- sion (see the review study3). Tey range from simple deterministic population-based models4–6, that assume uniform mixing, to complex agent-based models2,7 in which individuals defned by diferent attributes related to their susceptibility, infectiousness and social interactions transmit the pathogen to each other, given rise to heterogeneous transmission patterns. Irrespective of their complexity, the accuracy of these models is constrained by the validity of the epidemio- logical parameters that underpin them. For example, the model parameters controlling the risk of infection together with the social contact between infectious and susceptible individuals determine the transmission rate, which in turns infuences the peak and duration of the epidemics. By manipulating these parameters, model- ers can represent the impact of public health measures such as social distancing (lowering the contact rate) or wearing protective masks (lowering the risk of infection). Centre for Big Data Research in Health, UNSW Sydney, Sydney, NSW 2052, Australia. *email: [email protected] Scientifc Reports | (2021) 11:11029 | https://doi.org/10.1038/s41598-021-90347-8 1 Vol.:(0123456789) www.nature.com/scientificreports/ Figure 1. Simulation model structure. Te population is divided into the following fve classes: susceptible, exposed (non-infectious and asymptomatic), infectious (asymptomatic and symptomatic), removed (i.e., isolated, recovered, or otherwise non-infectious) and dead. Te reported data is modeled with four classes: observed positive tests, reported infections, removed and deaths. Tis study evaluates the efectiveness of initial public health interventions globally by estimating the efective reproduction number (the expected number of secondary infections resulting from an infectious individual) on a daily basis and highlights the importance of using updated reproduction numbers for outbreak forecast. In the second section, we describe the “Methods”. In “Results”, we frst present the patterns of initial public health interventions and efective reproduction numbers for a fxed temporal window across countries. Ten, we look into the temporal patterns of number of public health interventions, efective reproduction number and inci- dence counts using a time-lagged cross correlation analysis. Lastly, future outbreaks are predicted to illustrate how the currency of epidemiological parameters can infuence the forecasting of expected infection counts. We end with a “Discussion”. Methods Data sources. By 15 May 2020, there were 188 countries with recorded SARS-COV-2 infections, and 101 of them had implemented at least one public health intervention to contain the spread of the virus and had at least one death8. We modeled the transmission dynamics of SARS-COV-2 between 22 Jan to 15 May 2020 by ft- ting a stochastic SEIRD model9 (illustrated in Fig. 1) to three available time series: (1) daily number of incident cases, (2) daily number of deaths, and (3) daily number of recoveries, as recorded for each country by the Johns Hopkins Coronavirus Resource Center10. To monitor public health interventions, we captured government stringent policies using the Oxford COVID- 19 government response tracker 8, which contains 17 policies organized into three groups: containment and closure policies, economic policies and health system policies. We explored 12 of these policies, namely those that have a more immediate and direct impact on individual’s lifestyle and well-being. Social distancing policies (including travel policies) were further classifed into two levels depending on whether the government took a recommended or required stance. International travel controls were divided into four levels: (1) screening arrivals, (2) quarantine arrivals from some or all regions, (3) ban arrivals from some regions, and (4) ban on all regions or total border closure. Epidemic simulation model and analysis of transmission patterns. We built an extended version of a SEIRD model that included transitions between reporting states and disease states in order to account for delays in reporting (Fig. 1). Te model incorporates uncertainty in reported counts by explicitly modeling a Poisson process of daily reported infection, recovered and death counts, as well as of tested individuals. Follow- ing previous literature11,12, the mean incubation period was assumed to be Erlang distributed with mean 5.2 days (standard deviation: 3.7)13, and the mean infectious period was assumed to be Erlang distributed with mean 2.9 days (2.1)12. A sensitivity analysis of these two parameters on the prediction performance of the SEIRD model was performed and can be found in the supplementary material S1. For each country k, the daily transmission rate ( βt,k ) and fatality rate ( ξt,k ) were modeled as a geometric random walk process, and sequential Monte Carlo simulation was used to infer the daily reproduction number, Rt,k (defned as the transmission rate over the assumed incubation period βt,k/γ ), as well as the daily fatality rate. Te remaining unknown parameters were estimated via grid search using the maximum likelihood method. In each country, we assumed the outbreak started from the frst infectious case and that the entire population was initially susceptible. Further details on the model can be found in the supplementary material S1. We summarized the patterns of estimated efective reproduction number using smoothed time series of Rt,k generated by the Savitzky-Golay fnite impulse response 14 (FIR) flter. We compared hierarchical clustering from three algorithms: the WPGMA (Weighted Pair Group Method with Arithmetic Mean), the centroid linkage algorithm and the Ward variance minimization method. Scientifc Reports | (2021) 11:11029 | https://doi.org/10.1038/s41598-021-90347-8 2 Vol:.(1234567890) www.nature.com/scientificreports/ Our study uses descriptive statistics to analyze the patterns of Rt,k and early public health interventions before 15 May 2020. We characterized the time series of Rt,k by the following metrics (we drop the country index k for simplicity): • Te days from the outbreak to the maximum of the estimated efective reproduction number curve (i.e. Rt peak) before 15 May 2020; • Rt peak duration, defned as the time it takes Rt to reduce to 50% of Rt peak; We related these metrics to three metrics captured over 8 social distancing
Details
-
File Typepdf
-
Upload Time-
-
Content LanguagesEnglish
-
Upload UserAnonymous/Not logged-in
-
File Pages9 Page
-
File Size-