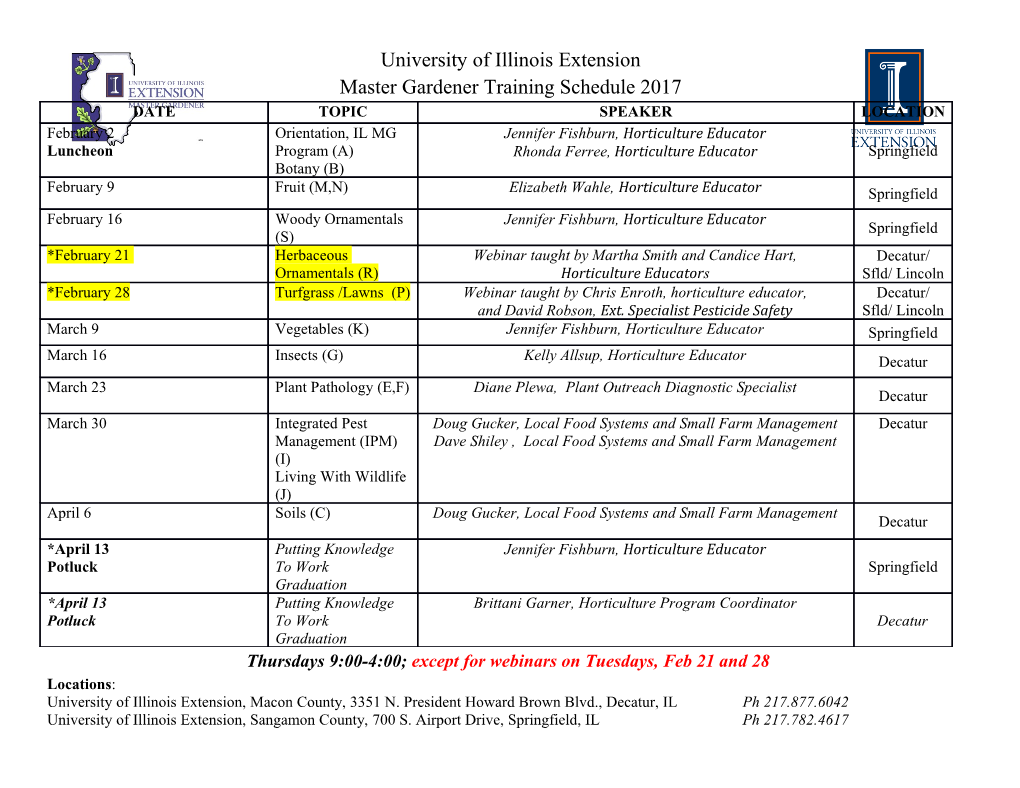
Volume-5, Issue-5, May - 2016 • ISSN No 2277 - 8160 IF : 3.62 | IC Value 70.36 Commerce Research Paper Statistics Inference in Nonlinear Regression Model With Heteroscedastic Errors B. Mahaboob Research Scholar, Department of Mathematics Dr. M. Ramesh Senior Data Scientist, Tech Mahindra, Hyderabad. Dr. Sk. Khadar Assistant Professor (Senior), Department of Mathematics, VIT, Vellore, Babu Tamilnadu. Academic Consultant, Department of CSE, SPMVV Engineering P. Srivyshnavi College, Tirupati. Prof. P. B.Sireesha Research Scholar, Department of Statistics, S.V.University, Tirupati. Balasiddamuni Rtd. Professor ABSTRACT An advent of modern computer science has made it possible for the applied mathematician to study the inferential aspects of an increasing number of nonlinear regression models in recent years. The inferential aspects for nonlinear regression models and the error assumptions are usually analogous to those made for linear regression models. The tests for the hypotheses on parameters of nonlinear regression models are usually based on nonlinear least squares estimates and the normal assumptions of error variables. A more common problem with data that are best fit by a nonlinear regression model than with data that can be adequately fit by the linear regression model is the problem of heteroscedasticity of errors. The problem of heteroscedasticity can be studied with reference to nonlinear regression models in a variety of ways. In the present study, an attempt has been made by developing inferential method for heteroscedastic nonlinear regression model. KEYWORDS : Intrinsically Nonlinear model; Violation of assumptions on Errors; Problem of Heteroscedasticity. I. INTRODUCTION In estimating the linear regression models, the least squares method of estimation is often applied to estimate the unknown parameters of the linear regression model and errors are usually assumed to be independent and identically distributed normal random variables with zero mean and unknown constant variance. The violations of these crucial assumptions on error variables can have several consequences on estimates of parameters and test statistics. The inferential aspects of nonlinear regression models and the error assumptions are usually analogous to those made for linear regression models. The tests for the hypotheses on parameters of nonlinear regression models are usually based on nonlinear least squares estimates and the normal assumptions of error variables. Among all assumptions, an important assumption of the regression model is that the errors need to have the constant or homoscedastic variance. Errors that do not have constant variances are known as heteroscedastic errors. The presence of heteroscedastic errors in the nonlinear regression model disturbs the optimal properties of the NLLS estimators of the parameters. These errors produce inefficient estimates of the parameters and invalid inferences concerning the true values of the parameters of the nonlinear regression model. The various inferential problems on nonlinear regression models involving heteroscedastic errors have been studied by Gallant and Goebel (1976), Carroll and Ruppert (1982a, 1982b), Cook and Weisberg (1983), Baljet (1986), Beal and Sheiner (1988), Welsh, Carroll and Ruppert (1994), Smyth (2002), Fox and Wiesberg (2010), Potocky and Stehlik (2010) and others. II. NONLINEAR STUDENTIZED RESIDUALS Residuals have vital role in many testing procedures designed to examine various types of disagreement between data and an assumed nonlinear regression model. In testing nonlinear hypotheses and procedures for detecting the problem of heteroscedasticity, several transformations of the Nonlinear least squares (NLLS) residuals have been suggested to overcome partially some of their shortcomings. GJRA - GLOBAL JOURNAL FOR RESEARCH ANALYSIS X 184 IF : 3.62 | IC Value 70.36 Volume-5, Issue-5, May - 2016 • ISSN No 2277 - 8160 Consider the standard nonlinear regression model with usual assumptions in vector notation as Yfn1 n1 n1 ...(2.1) and is p1 vector of unknown parameters. Suppose that ˆ is the nonlinear least squares estimator of . For large samples, the NLLS residuals vector is given by ˆ ˆ e YY Yf …(2.2) 1 where ˆ FF F …(2.3) and F F f X, …(2.4) i j np th Here, f X,i is the (i,j) element of np matrix F . j An approximate relationship between e and is given by 1 e M, where M I F FF F 1 or eI , where ij F FF F is symmetric idempotent matrix known as ‘HAT’ matrix. or in scalar form, n ei i ij j , i=1,2,….,n j1 …(2.5) Since, is symmetric idempotent matrix, it follows that trace( ) = rank ( ) = p n 2 and ij ii , i=1,2,…,n j1 If follows N O, 2 I then e follows a singular normal distribution with zero mean vector and variance 2I. Here controls the variation in e. 2 Since, the variance of each ei is a function of both and ii , i=1,2,…,n; the NLLS residuals have a probability distribution that is scale dependent. The nonlinear studentized residuals do not depend on either of these quantities and they have probability distribution that is free of the nuisance scale parameters. One can make a further distinction between internal studentization and external studentization. (A) INTERNALLY NONLINEAR STUDENTIZED RESIDUALS In NLLS regression, the internally nonlinear studentized residuals are defined by, * ei ei , i 1,2,...,n …(2.6) ˆ 1 ii GJRA - GLOBAL JOURNAL FOR RESEARCH ANALYSIS X 185 Volume-5, Issue-5, May - 2016 • ISSN No 2277 - 8160 IF : 3.62 | IC Value 70.36 n e2 ^ ee i where 2 i1 …(2.7) np np 2 e* asy 1 n p1 Here, i ~ Beta distribution with parameters and . np 2 2 It follows that Ee i 0and Var ei 1, i 1,2,...,n ** ij Also, Cov eij ,e 1 , ,i j 1,2...,n 2 11ii jj (B) EXTERNALLY NONLINEAR STUNDENTIZED RESIDUALS The externally nonlinear studentized residuals are defined by, ** ei ei 1 , i 1,2,...,n …(2.8) 2 ˆi 1 ii ^ 2 2 e np i ^ 2 1 ii where i n p1 2 ^^npe* or 22 i i n p1 ^ 2 Under normality i and ei are independent. ** Here, e~i Student’s t- distribution with n p1degrees of freedom. A relationship between internally and externally nonlinear studentized residuals is given by 1 2 ** * n p1 eii e 2 , i 1,2,...,n …(2.9) * npei 2 ** ** Thus, ei is a monotonic transformation of ei . III. ESTIMATION OF PARAMETERS OF NONLINEAR REGRESSION MODEL WITH HETEROSCEDASTIC ERRORS BY USING NONLINEAR STUDENTIZED RESIDUALS Consider the standard nonlinear regression model Yiii f X , , i 1,2,...,n …(3.1) which may be written in matrix notation as Yfn1 n1 n1 …(3.2) th where Xi X i1 ,X i2 ,....,X ik is a k- component vector denotes the i observation on known k-explanatory variables; GJRA - GLOBAL JOURNAL FOR RESEARCH ANALYSIS X 186 IF : 3.62 | IC Value 70.36 Volume-5, Issue-5, May - 2016 • ISSN No 2277 - 8160 is a p1 vector of unknown parameters; f is a known twice continuously differentiable function of ; The usual assumptions of the nonlinear regression model are: i. E Yii X f X i , , i 1,2,....,n; ii. is estimable or identified; iii. Eii f X, 0 22 iv. Eij f X , , j 1,2,..,n a finite constant and Eij f X i , ,f Xj , , i, j 1,2,..,n 0 j i That is, the i 'sare conditional homoscedastic and nonautocorrelated random error 2 variables (or) EI n v. i 's are normally distributed. i.e., i.i.d 2 i ~ N O, , i 1,2,...,n By minimizing the residual sum of squares ˆˆˆ with respect to ˆ , for large samples, under iterative R Yf Yf process, an iterative nonlinear least squares (NLLS) estimator for is given by 1 ˆˆ F ˆˆ F F ˆ Yf ˆ …(3.3) n1 n n n n n ˆ f where Fn as the regressor matrix. ˆ n Here, all the terms on the R.H.S of (3.3) are evaluated at ˆ and Yf ˆ is the n n vector of nonlinear least squares residuals for an arbitrary value of . By violating the assumption of homoscedastic errors in the nonlinear model (3.1) one may assume that E 2 …(3.4) where or is symmetric positive definite matrix If the diagonal elements of dispersion matrix are not all identical and is free from autocorrelation then can be considered a diagonal matrix. 22 2 diag12 , ,...., n …(3.5) th 2 and with i diagonal element is given by i . Define the proposed iterative NLLS residual vector based on ˆ as e Yf ˆ . n nn Also, Iterative Nonlinear Internally Studentized Residuals are defined by * eni eni , i 1,2,...,n …(3.6) ˆ 1 nii GJRA - GLOBAL JOURNAL FOR RESEARCH ANALYSIS X 187 Volume-5, Issue-5, May - 2016 • ISSN No 2277 - 8160 IF : 3.62 | IC Value 70.36 1 where F ˆ FF ˆˆ F ˆ n nij n n n n …(3.7) is symmetric idempotent matrix known as ‘HAT’ matrix. n 2 ^ eni 2 eenn i1 …(3.8) np np 2 e* asy Here, ni ~ Beta distribution with parameters ½ and n p12 , np It follows that, Ee ni 0 and Var eni 1, i 1,2,...,n ** nij Also, cov eni ,e nj 1 , i j 1,2,....,n …(3.9) 2 11nii njj Consider an estimator for 22 2 ˆ * ** * n diag en1 ,e n2 ,.....e nn …(3.10) Now, an Iterative Estimated Nonlinear Generalized Least Squares (IENLGLS) estimator for is given by 1 1 ^ * * ** * * * n1 n F n n F n F n Yf n …(3.11) * f * Here F n as the regressor matrix and Yf n is the vector of ~ * n IENLGLS residuals for an arbitrary value of . 1 1 ^ * ** * Further Varn F nn F n …(3.12) IV.
Details
-
File Typepdf
-
Upload Time-
-
Content LanguagesEnglish
-
Upload UserAnonymous/Not logged-in
-
File Pages7 Page
-
File Size-