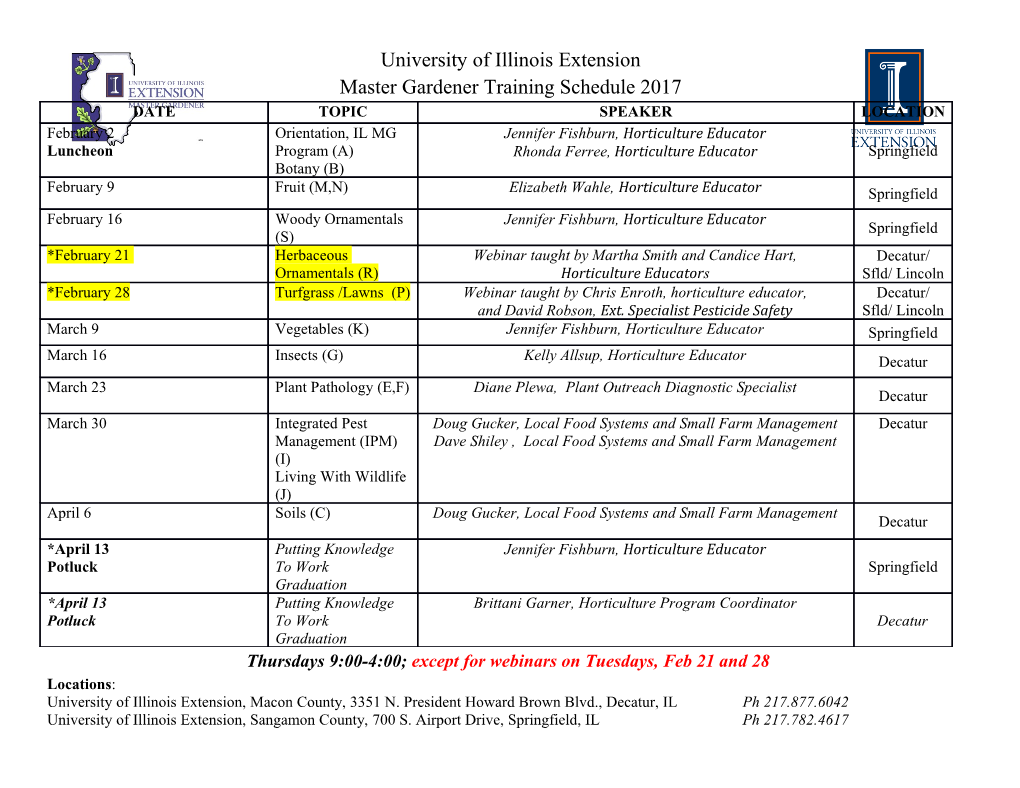
Open Knowledge Enrichment for Long-tail Entities Ermei Cao∗ Difeng Wang∗ State Key Laboratory for Novel Software Technology State Key Laboratory for Novel Software Technology Nanjing University, China Nanjing University, China [email protected] [email protected] Jiacheng Huang Wei Hu† State Key Laboratory for Novel Software Technology State Key Laboratory for Novel Software Technology Nanjing University, China National Institute of Healthcare Data Science [email protected] Nanjing University, China [email protected] ABSTRACT 10,000,000 Knowledge bases (KBs) have gradually become a valuable asset for 1,000,000 many AI applications. While many current KBs are quite large, they 100,000 are widely acknowledged as incomplete, especially lacking facts entities 10,000 of long-tail entities, e.g., less famous persons. Existing approaches of enrich KBs mainly on completing missing links or filling missing 1,000 values. However, they only tackle a part of the enrichment problem Number 100 # long-tail # popular and lack specific considerations regarding long-tail entities. In this 10 entities entities paper, we propose a full-fledged approach to knowledge enrichment, = 2.1M = 7,655 1 which predicts missing properties and infers true facts of long-tail 1 10 100 1,000 10,000 100,000 1,000,000 entities from the open Web. Prior knowledge from popular entities Number of triples per entity is leveraged to improve every enrichment step. Our experiments on Figure 1: Distribution of number of entities versus number the synthetic and real-world datasets and comparison with related of triples per entity (in log-log scale) work demonstrate the feasibility and superiority of the approach. places and award-winning works), but are overwhelmingly incom- KEYWORDS plete on less popular (e.g., long-tail) entities [7, 40, 52]. For instance, as shown in Figure 1, around 2.1 million entities in Freebase have Knowledge enrichment; long-tail entities; knowledge base augmen- less than 10 facts per entity, while 7,655 entities have more than tation; property prediction; fact verification; graph neural networks one thousand facts, following the so-called power-law distribution. ACM Reference Format: Among those long-tail entities, some just lack facts in KBs rather Ermei Cao, Difeng Wang, Jiacheng Huang, and Wei Hu. 2020. Open Knowl- than in the real world. The causes of the incompleteness are mani- edge Enrichment for Long-tail Entities. In Proceedings of The Web Conference fold. First, the construction of large KBs typically relies on soliciting 2020 (WWW ’20), April 20–24, 2020, Taipei, Taiwan. ACM, New York, NY, contributions from human volunteers or distilling knowledge from USA, 11 pages. https://doi.org/10.1145/3366423.3380123 “cherry-picked” sources like Wikipedia, which may yield a limited coverage on frequently-mentioned facts [7]. Second, some formerly 1 INTRODUCTION unimportant or unknown entities may rise to fame suddenly, due The last few years have witnessed that knowledge bases (KBs) have to the dynamics of this ever-changing world [18]. However, current become a valuable asset for many AI applications, such as semantic KBs may not be updated in time. Because the Web has become the search, question answering and recommender systems. Some exist- main source for people to access information nowadays, the goal arXiv:2002.06397v2 [cs.IR] 19 Feb 2020 ing KBs, e.g., Freebase, DBpedia, Wikidata and Probase, are very of this paper is to conjecture what facts about long-tail entities are large, containing millions of entities and billions of facts, where a missing, as well as extract and infer true facts from various Web fact is organized as a triple in the form of hentity;property;valuei. sources. We believe that enriching long-tail entities with uncovered However, it has been aware that the existing KBs are likely to have facts from the open Web is vital for building more complete KBs. high recall on the facts of popular entities (e.g., celebrities, famous State-of-the-art and limitations. As investigated in [38], the cost of curating a fact manually is much more expensive than that ∗Equal contributors †Corresponding author of automatic creation, by a factor of 15 to 250. Due to the vast scale of long-tail entities in KBs and accessible knowledge on the Web, This paper is published under the Creative Commons Attribution 4.0 International automation is inevitable. Existing approaches address this problem (CC-BY 4.0) license. Authors reserve their rights to disseminate the work on their personal and corporate Web sites with the appropriate attribution. from various angles [12, 37, 49], however, we argue that they may WWW ’20, April 20–24, 2020, Taipei, Taiwan have the following two limitations: © 2020 IW3C2 (International World Wide Web Conference Committee), published First, existing approaches only deal with a part of the knowledge under Creative Commons CC-BY 4.0 License. ACM ISBN 978-1-4503-7023-3/20/04. enrichment problem, such as recommending properties to entities https://doi.org/10.1145/3366423.3380123 [23, 53], predicting missing links between entities [3, 6, 44, 45] and WWW ’20, April 20–24, 2020, Taipei, Taiwan Ermei Cao, Difeng Wang, Jiacheng Huang, and Wei Hu verifying the truths of facts [24]. Also, the KB population (KBP) or 1 slot filling approaches usually assume that the target properties Property prediction a long-tail predicted are given beforehand and extract values from free text [4, 46] or entity properties structured Web tables [22, 42, 52]. To the best of our knowledge, 2 Web KB none of them can accomplish open knowledge enrichment alone. popular Value Second, most approaches lack considerations for long-tail enti- entities extraction ties. Due to the lack of facts about long-tail entities, the link predic- facts about values from tion approaches may not learn good embeddings for them. Simi- the entity 3 the Web larly, the KBP approaches would be error-sensitive for incidentally- Fact verification appeared entities, as they cannot handle errors or exceptions well. We note that a few works have begun to study the long-tail phe- Figure 2: Overview of the approach nomenon in KBs, but they tackle different problems, e.g., linking 2 OVERVIEW OF THE APPROACH long-tail entities to KBs [8], extracting long-tail relations [54] and In this paper, we deal with RDF KBs such as Freebase, DBpedia and verifying facts for long-tail domains [24]. Wikidata. A KB is defined as a 5-tuple KB = ¹E; P;T ; L; Fº, where Our approach and contributions. To address the above limita- E; P;T; L; F denote the sets of entities, properties, classes, literals tions, we propose OKELE, a full-fledged approach to enrich long-tail and facts, respectively. A fact is a triple of the form f = he;p; xi 2 entities from the open Web. OKELE works based on the idea that E × P × ¹E [T [ Lº, e.g., hErika_Harlacher; profession; Voice_Actori. we can infer the missing knowledge of long-tail entities by com- Moreover, properties can be divided into relations and attributes. paring with similar popular entities. For instance, to find out what Usually, it is hard to strictly distinguish long-tail entities and non- a person lacks, we can see what other persons have. We argue that, long-tail entities. Here, we roughly say that an entity is a long-tail this may not be the best solution, but it is sufficiently intuitive and entity if it uses a small number of distinct properties (e.g., ≤ 5). very effective in practice. Given a long-tail entity e in a KB, open knowledge enrichment Specifically, given a long-tail entity, OKELE aims to search the aims at tapping into the masses of data over the Web to infer the Web to find a set of true facts about it. To achieve this, we deal with missing knowledge (e.g., properties and facts) of e and add it back several challenges: First, the candidate properties for a long-tail en- to the KB. A Web source, denoted by s, makes a set of claims, each tity can be vast. We construct an entity-property graph and propose of which is in the form of c = ¹f ;s;oº, where f ;s;o are a fact, a a graph neural network (GNN) based model to predict appropriate source and an observation, respectively. In practice, o often takes a properties for it. Second, the values of a long-tail entity are scat- confidence value about how confident f is present in s according tered all over the Web. We consider various types of Web sources to some extraction method. Also, f has a label l 2 fTrue; Falseg. and design corresponding extraction methods to retrieve them. f Figure 2 shows the workflow of our approach, which accepts a Third, the extracted facts from different sources may have conflicts. long-tail entity as input and conducts the following three steps: We propose a probabilistic graphical model to infer the true facts, which particularly considers the imbalance between small and large (1) Property prediction. Based on the observations that sim- sources. Note that, during the whole process, OKELE makes full ilar entities are likely to share overlapped properties and use of popular entities to improve the enrichment accuracy. popular entities have more properties, we resort to similar The main contributions of this paper are summarized as follows: popular entities. Our approach first creates an entity-property graph to model the interactions between entities and prop- • We propose a full-fledged approach for open knowledge erties. Then, it employs an attention-based GNN model to enrichment on long-tail entities. As far as we know, this is predict the properties of the long-tail entity.
Details
-
File Typepdf
-
Upload Time-
-
Content LanguagesEnglish
-
Upload UserAnonymous/Not logged-in
-
File Pages11 Page
-
File Size-