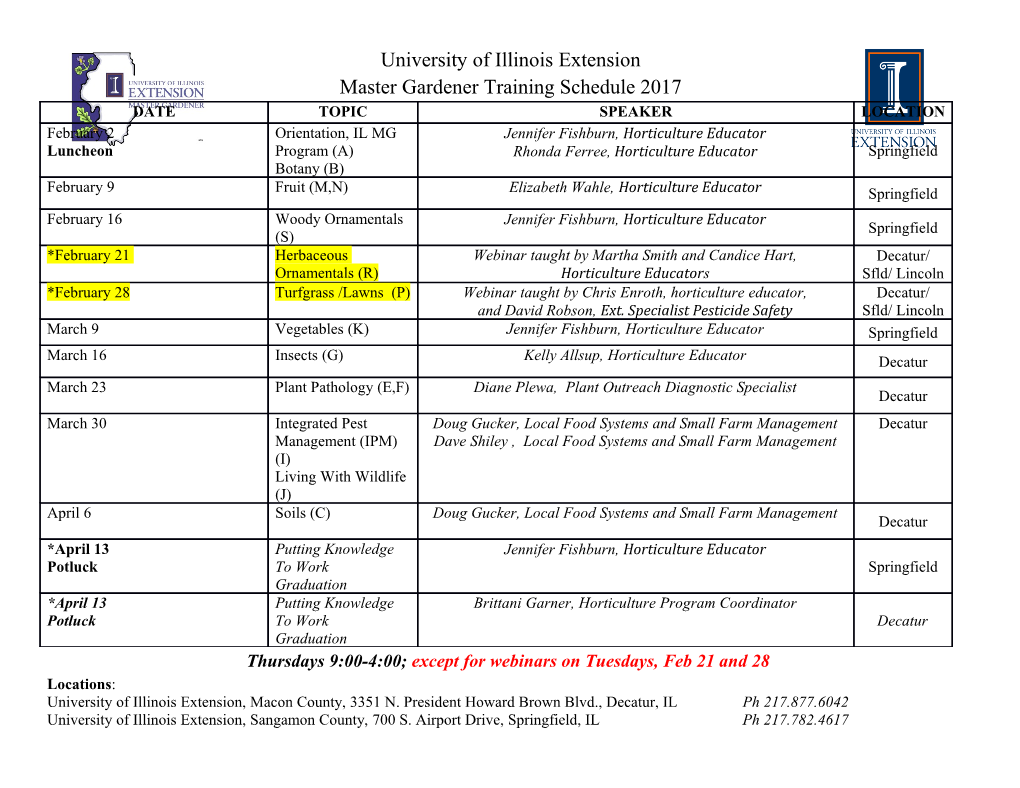
THE NOTION OF MIXING AND RANK ONE EXAMPLES WENYU CHEN Abstract. This paper discusses the relationships among mixing, lightly mix- ing, weakly mixing and ergodicity. Besides proving the hierarchy of these notions, we construct examples of rank one transformations that exhibit each of these behaviors to demonstrate the differences. Contents 1. Preliminaries 1 1.1. Convergence 1 1.2. Sets of Density Zero 2 1.3. Dynamical Systems 2 2. Ergodicity 3 3. Mixing and Weakly Mixing 5 4. Mixing and Lightly Mixing 9 5. Rank One Maps 10 5.1. The Dyadic Odometer 11 5.2. Chac´on'sTransformation 15 5.3. Just Lightly Mixing Map 19 6. Discussion 24 Acknowledgments 25 References 26 1. Preliminaries We assume knowledge of measure theory (for example, chapters 1 and 2 of [5] would suffice). We use the same notation as in standard measure theory. 1.1. Convergence. Definition 1.1. A bounded sequence ai may converge to a number a in at least three ways. f g (1) Convergence: lim ai = a: i !1 (2) Strong Ces`aroconvergence: n 1 1 X− lim ai a = 0: n n j − j !1 i=0 Date: August 27 2015. 1 2 WENYU CHEN (3) Ces`aroconvergence: n 1 1 X− lim ai = a: n n !1 i=0 Lemma 1.2. Let ai be a bounded sequence. Then convergence implies Strong Ces`aro convergence,f andg Strong Ces`aro convergence implies Ces`aro convergence. Proof. The result follows easily by applying the - δ definition of limits. 1.2. Sets of Density Zero. Definition 1.3. A set D of nonnegative integers is said to be of zero density if n 1 1 X− lim ID(i) = 0; n n !1 i=0 where ID is the characteristic function defined by ID(x) = 1 if x D and ID(x) = 0 if x = D. 2 2 Definition 1.4. Let a R. We say a sequence ai of real numbers converges 2 f g in density to a if there exists a zero density set D N such that for any > 0, there is an integer N such that if i > N and i = D, then⊂ a a < . 2 j i − j Lemma 1.5. If bi is a bounded sequence of nonnegative real numbers, then bi converges Ces`arof tog0 if and only if b converges in density to 0. f g f ig Proof. The result follows easily by applying the definitions of convergences. 1.3. Dynamical Systems. Definition 1.6 (Dynamical System). A measurable dynamical system is a quadruple (X; ; µ, T ), where X is a space, a σ-algebra of subsets of X, µ a measure on Sand T : X X a measurableS transformation with respect to µ. Specifically, (SX; ; µ, T ) is a!Lebesgue measure-preserving dynamical system if (X; ; µ) is aS Lebesgue measurable space and T is a measure-preserving trans- formationS T : X X. We will refer to such system simply as measure-preserving dynamical system! in this paper. Though we are not going to study the \sameness" of dynamical systems, we define the notion of factor for technical reasons. A factor of a dynamical system carries some of its dynamical properties. Later we show if a dynamical system has an ergodic transformation, then so does its factor. Definition 1.7. Let (X; ; µ, T ) be a measurable dynamical system. A dynamical S system (X0; 0; µ0;T 0) is a factor of (X; ; µ, T ) if there exist measurable sets X S S 0 ⊂ X and X0 X0 of full measure with T (X ) X; T 0(X0 ) X0, and a factor map 0 ⊂ 0 ⊂ 0 ⊂ φ : X X0 such that: 0 ! 0 (1) φ is onto 1 (2) φ− (A) (X0) for all A X0 1 2 S 2 (3) µ(φ− (A)) = µ0(A) for all A 0(X0 ) 2 S 0 (4) φ(T (x)) = T 0(φ(x)). THE NOTION OF MIXING AND RANK ONE EXAMPLES 3 2. Ergodicity Definition 2.1 (Ergodicity). A measure-preserving transformation T is ergodic 1 if whenever A is a measurable set that is strictly T -invariant (i.e., T − (A) = A), then µ(A) is either 0 or 1. Theorem 2.2 (Birkhoff's ergodic theorem). Let (X; ; µ) be a probability space S and let T be a measure-preserving transformation on (X; ; µ). If f : X R is an integrable function, then S ! 1 Pn 1 i (1) limn − f(T (x)) exists for all x X N, for some null set N !1 n i=0 2 n depending on f. Denote this limit by f~. (2) f~(T x) = f~(x) a.e. (3) For any measurable set A that is T -invariant, Z Z fdµ = fdµ.~ A A In particular, if T is ergodic, then n 1 1 X− Z lim f(T i(x)) = fdµ a.e. n n !1 i=0 For the proof, see chapter 5 of [9]. Theorem 2.3. Let T be a finite measure-preserving transformation on a probability space (X; ; µ). T is ergodic if and only if for all measurable sets A and B, S n 1 1 X− i (2.4) lim µ(T − (A) B) = µ(A)µ(B): n n \ !1 i=0 Proof. First we prove the forward direction. Suppose T is ergodic and A, B mea- surable. Apply the Birkhoff ergodic theorem on T , A, B, and the characteristic map IA IB to get n 1 Z 1 X− i lim IA(T (x)) = IAdµ = µ(A) a.e. n n !1 i=0 Thus, n 1 1 X− i lim IA(T (x))IB(x) = µ(A)IB(x) a.e. n n !1 i=0 i Observe that IA(T (x))IB(x) 1 a:e:, which enables us to apply the dominated convergence theorem.j This givesj ≤ Z n 1 Z n 1 1 X− i 1 X− i lim IA(T (x))IB(x)dµ(x) = lim IA(T (x))IB(x)dµ(x) n n n n !1 i=0 !1 i=0 Z = µ(A)IB(x)dµ(x) = µ(A)µ(B): 4 WENYU CHEN Also, we know Z n 1 n 1 Z 1 X− i 1 X− i IA(T (x))IB(x)dµ(x) = IA(T (x))IB(x)dµ(x) n n i=0 i=0 n 1 1 X− i = µ(T − (A) B): n \ i=0 We take the limit of both sides and obtain what we want to show. Now we prove the reverse direction. Suppose (2.4) holds for all sets A and B in X. Then it also holds for A = B. Let A be T -invariant. By the defini- 1 Pn 1 i tion of T -invariant, limn − µ(T − (A) A) = µ(A): Let A = B. We get !1 n i=0 1 Pn 1 i 2 \ 2 limn − µ(T − (A) A) = µ(A) : This gives µ(A) = µ(A) , which implies !1 n i=0 \ µ(A) is 0 or 1. Thus T is ergodic. Proposition 2.5. Let T be defined on a probability space (X; (X); µ), T T defined on (X X; (X) (X); µ µ). If T T is an ergodicS transformation,× then T is also ergodic.× S × S × × 1 Proof. Let A be a strictly T -invariant measurable set. Then (T T )− (A A) = 1 1 × × T − (A) T − (A) = A A, so A A is (T T )-invariant. The property of product measure× shows × × × (µ µ)(A A) = µ(A)µ(A): × × Since T T is ergodic, (µ µ)(A A) is 1 or 0. Therefore µ(A) is either 1 or 0. × × × Hence T is ergodic. Theorem 2.6. Let (Y; 0(Y ); ν; S) be a factor of (X; (X); µ, T ). If T is ergodic, so is S. S S Proof. Let φ : X Y be a factor map. If A is a strictly S-invariant set, then ! 1 1 1 1 1 T − (φ− (A)) = φ− (S− (A)) = φ− (A): (The first equality holds by Definition 1.7 and the second holds because A is S- 1 1 invariant.) Thus φ− (A) is a T -invariant set. Also by Definition 1.7, µ(φ− (A)) = ν(A). Since T is ergodic, ν(A) is 0 or 1, i.e., S is ergodic. Definition 2.7 (Eigenvalue and Eigenfunction). Let (X; ; µ) be a probability space and let T : X X be a measure-preserving transformation.S We say a ! number µ C is an eigenvalue of T if there exists a function f L2(X; ; µ) which is nonzero2 a.e. such that 2 S f(T (x)) = λf(x) µ-a.e. The function f is called an eigenfunction. Lemma 2.8. If λ is an eigenvalue for a measure-preserving transformation T , then λ = 1. j j Proof. Let T be a measure-preserving transformation and f be its eigenfunction. Let λ be its eigenvalue. Then λ 2 R f 2dµ = R λ 2 f 2dµ = R f T 2dµ = R f 2 j j j j j j j j j ◦ j j j ◦ T dµ = R f 2dµ. j j Lemma 2.9. If T is ergodic and f is an eigenfunction, then f is constant a.e. j j THE NOTION OF MIXING AND RANK ONE EXAMPLES 5 Proof. Let f be an eigenfunction of T with eigenvalue λ, i.e., f T = λf a.e. By Lemma 2.8, we know λ = 1.
Details
-
File Typepdf
-
Upload Time-
-
Content LanguagesEnglish
-
Upload UserAnonymous/Not logged-in
-
File Pages26 Page
-
File Size-