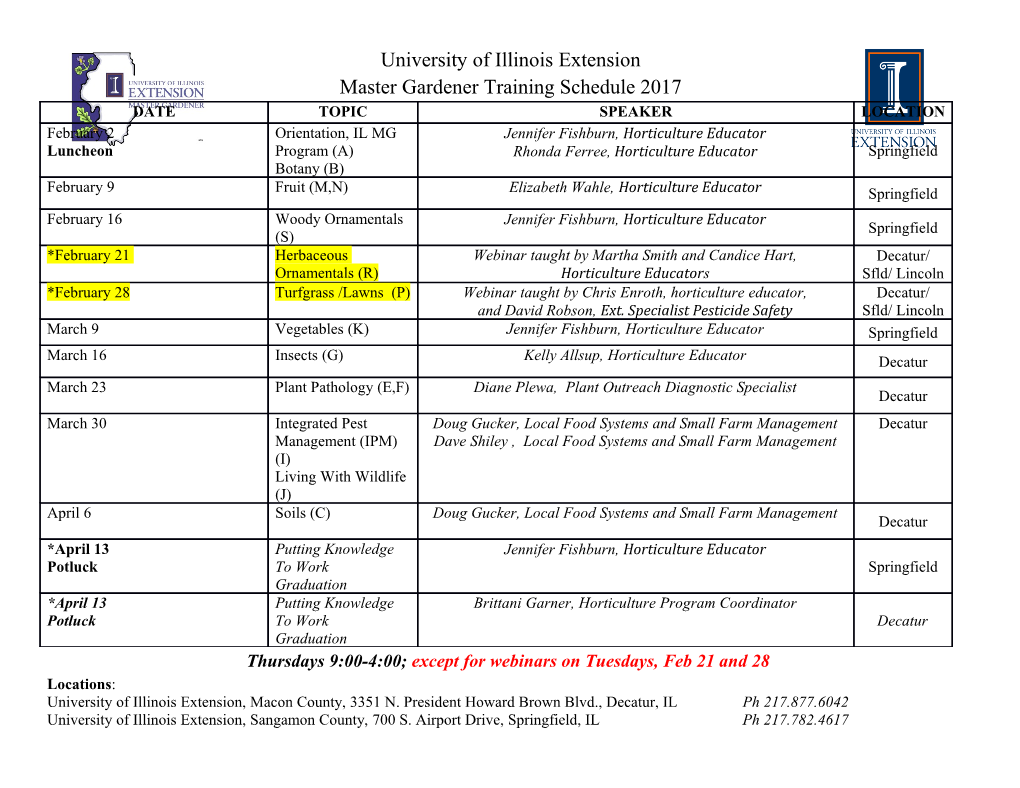
IEEE TRANSACTIONS ON INFORMATION THEORY, VOL. 56, NO. 8, AUGUST 2010 3721 Rényi Information Dimension: Fundamental Limits of Almost Lossless Analog Compression Yihong Wu, Student Member, IEEE, and Sergio Verdú, Fellow, IEEE Abstract—In Shannon theory, lossless source coding deals with • The central problem is to determine how many compressed the optimal compression of discrete sources. Compressed sensing measurements are sufficient/necessary for recovery with is a lossless coding strategy for analog sources by means of mul- vanishing block error probability as blocklength tends to tiplication by real-valued matrices. In this paper we study almost lossless analog compression for analog memoryless sources in an infinity [2]–[4]. information-theoretic framework, in which the compressor or de- • Random coding is employed to show the existence of compressor is constrained by various regularity conditions, in par- “good” linear encoders. In particular, when the random ticular linearity of the compressor and Lipschitz continuity of the projection matrices follow certain distribution (e.g., stan- decompressor. The fundamental limit is shown to be the informa- dard Gaussian), the restricted isometry property (RIP) is tion dimension proposed by Rényi in 1959. satisfied with overwhelming probability and guarantees Index Terms—Analog compression, compressed sensing, infor- exact recovery. mation measures, Rényi information dimension, Shannon theory, On the other hand, there are also significantly different ingre- source coding. dients in compressed sensing in comparison with information theoretic setups. I. INTRODUCTION • Sources are not modeled probabilistically, and the funda- mental limits are on a worst case basis rather than on av- A. Motivations From Compressed Sensing erage. Moreover, block error probability is with respect to the distribution of the encoding random matrices. HE “Bit” is the universal currency in lossless source • Real-valued sparse vectors are encoded by real numbers T coding theory [1], where Shannon entropy is the fun- instead of bits. damental limit of compression rate for discrete memoryless • The encoder is confined to be linear while generally in sources (DMS). Sources are modeled by stochastic processes information-theoretical problems such as lossless source and redundancy is exploited as probability is concentrated coding we have the freedom to choose the best possible on a set of exponentially small cardinality as blocklength coding scheme. grows. Therefore, by encoding this subset, data compression Departing from the compressed sensing literature, we study fun- is achieved if we tolerate a positive, though arbitrarily small, damental limits of lossless source coding for real-valued mem- block error probability. oryless sources within an information theoretic setup. Compressed sensing [2], [3] has recently emerged as an ap- • Sources are modeled by random processes. This method proach to lossless encoding of analog sources by real numbers is more flexible to describe source redundancy which en- rather than bits. It deals with efficient recovery of an unknown compasses, but is not limited to, sparsity. For example, a real vector from the information provided by linear measure- mixed discrete-continuous distribution is suitable for char- ments. The formulation of the problem is reminiscent of the tra- acterizing linearly sparse vectors [5], [6], i.e., those with a ditional lossless data compression in the following sense. number of nonzero components proportional to the block- • Sources are sparse in the sense that each vector is sup- length with high probability and whose nonzero compo- ported on a set much smaller than the blocklength. This nents are drawn from a given continuous distribution. kind of redundancy in terms of sparsity is exploited to • Block error probability is evaluated by averaging with re- achieve effective compression by taking fewer number of spect to the source. linear measurements. • While linear compression plays an important role in our de- • In contrast to lossy data compression, block error proba- velopment, our treatment encompasses weaker regularity bility, instead of distortion, is the performance benchmark. conditions. Methodologically, the relationship between our approach and Manuscript received March 02, 2009; revised April 30, 2010. Date of current compressed sensing is analogous to the relationship between version July 14, 2010. This work was supported in part by the National Science modern coding theory and classical coding theory: classical Foundation under Grants CCF-0635154 and CCF-0728445. The material in this coding theory adopts a worst case (Hamming) approach whose paper was presented in part at the IEEE International Symposium on Informa- tion Theory, Seoul, Korea, July 2009 [55]. goal is to obtain codes with a certain minimum distance, while The authors are with the Department of Electrical Engineering, Princeton modern coding theory adopts a statistical (Shannon) approach University, Princeton, NJ 08544 USA (e-mail: [email protected]; whose goal is to obtain codes with small probability of failure. [email protected]). Communicated by H. Yamamoto, Associate Editor for Shannon Theory. Likewise compressed sensing adopts a worst case model in Digital Object Identifier 10.1109/TIT.2010.2050803 which compressors work provided that the number of nonzero 0018-9448/$26.00 © 2010 IEEE Authorized licensed use limited to: Princeton University. Downloaded on July 20,2010 at 19:49:18 UTC from IEEE Xplore. Restrictions apply. 3722 IEEE TRANSACTIONS ON INFORMATION THEORY, VOL. 56, NO. 8, AUGUST 2010 components in the source does not exceed a certain threshold, version of the random vector. It characterizes the rate of growth while we adopt a statistical model in which compressors work of the information given by successively finer discretizations for most source realizations. In this sense, almost lossless of the space. Although a fundamental information measure, the analog compression can be viewed as an information theoretic Rényi dimension is far less well known than either the Shannon framework for compressed sensing. Probabilistic modeling entropy or the Rényi entropy. Rényi showed that under certain provides elegant results in terms of fundamental limit, as well conditions for an absolutely continuous -dimensional random as sheds light on constructive schemes on individual sequences. vector the information dimension is . Hence, he remarked in For example, not only is random coding a proof technique in [11] that “the geometrical (or topological) and information-the- Shannon theory, but also a guiding principle in modern coding oretical concepts of dimension coincide for absolutely contin- theory as well as in compressed sensing. uous probability distributions.” However, the operational role Recently there have been considerably new developments of Rényi information dimension has not been addressed before in using statistical signal models (e.g., mixed distributions) except in the work of Kawabata and Dembo [12], which relates in compressed sensing (e.g., [5]–[8]), where reconstruction it to the rate-distortion function. It is shown in [12] that when performance is evaluated by computing the asymptotic error the single-letter distortion function satisfies certain conditions, probability in the large-blocklength limit. As discussed in the rate-distortion function of a real-valued source scales Section IV-B, the performance of those practical algorithms proportionally to as , with the proportionality con- still lies far from the fundamental limit. stant being the information dimension of the source. This result serves to drop the assumption of continuity in the asymptotic B. Lossless Source Coding for Analog Sources tightness of Shannon’s lower bound in the low distortion regime. Discrete sources have been the sole object in lossless data In this paper we give an operational characterization of Rényi compression theory. The reason is at least twofold. First, information dimension as the fundamental limit of almost loss- nondiscrete sources have infinite entropy, which implies that less data compression for analog sources under various regu- representation with arbitrarily small block error probability larity constraints of the encoder/decoder. Moreover, we con- requires arbitrarily large rate. On the other hand, even if we sider the problem of lossless Minkowski dimension compres- consider encoding analog sources by real numbers, the result is sion, where the Minkowski dimension of a set measures its de- still trivial, as and have the same cardinality. Therefore, gree of fractality. In this setup we study the minimum upper a single real number is capable of representing a real vector Minkowski dimension of high-probability events of source re- losslessly, yielding a universal compression scheme for any alizations. This can be seen as a counterpart of lossless source analog source with zero rate and zero error probability. coding, which seeks the smallest cardinality of high-probability However, it is worth pointing out that the compression events. Rényi information dimension turns out to be the funda- method proposed above is not robust because the bijection be- mental limit for lossless Minkowski dimension compression. tween and is highly irregular. In fact, neither the encoder nor the decoder can be continuous [9, Exercise 6(c), p. 385]. D. Organization of the Paper Therefore, such a compression scheme is useless in the pres- Notations frequently used throughout the
Details
-
File Typepdf
-
Upload Time-
-
Content LanguagesEnglish
-
Upload UserAnonymous/Not logged-in
-
File Pages28 Page
-
File Size-