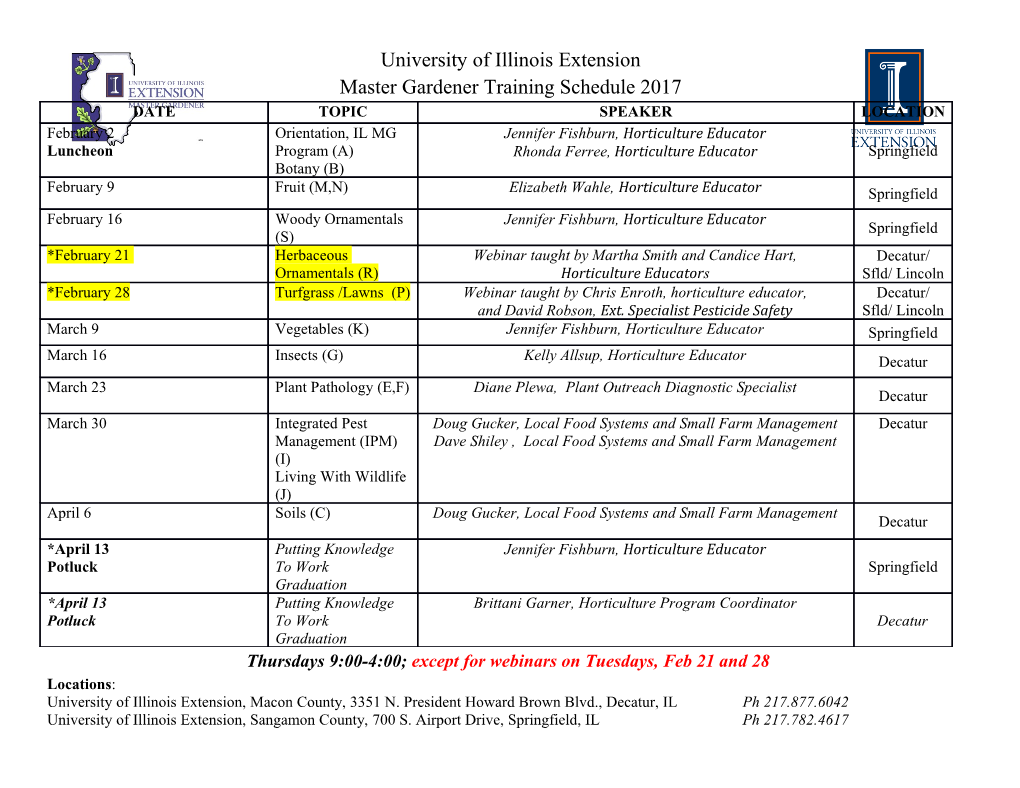
Big data conversion techniques including their main features and characteristics 2017 edition Main title 20 17 edition 17 STATISTICAL WORKING PAPERSBOOKS Big data conversion techniques including their main features and characteristics 2017 edition Manuscript completed in July 2017 Neither the European Commission nor any person acting on behalf of the Commission is responsible for the use that might be made of the following information. Luxembourg: Publications Office of the European Union, 2017 © European Union, 2017 Reuse is authorised provided the source is acknowledged. The reuse policy of European Commission documents is regulated by Decision 2011/833/EU (OJ L 330, 14.12.2011, p. 39). Copyright for photographs: © Shutterstock/Rawpixel.com For any use or reproduction of photos or other material that is not under the EU copyright, permission must be sought directly from the copyright holders. For more information, please consult: http://ec.europa.eu/eurostat/about/policies/copyright The information and views set out in this publication are those of the authors and do not necessarily reflect the official opinion of the European Union. Neither the European Union institutions and bodies nor any person acting on their behalf may be held responsible for the use which may be made of the information contained therein. PDF ISBN 978-92-79-70523-6 ISSN 2315-0807 doi:10.2785/461700 KS-TC-17-003-EN-N Abstract Abstract Big data have high potential for nowcasting and forecasting economic variables. However, they are often unstructured so that there is a need to transform them into a limited number of time series which efficiently summarise the relevant information for nowcasting or short term forecasting the economic indicators of interest. Data structuring and conversion is a difficult task, as the researcher is called to translate the unstructured data and summarise them into a format which is both meaningful and informative for the nowcasting exercise. In this paper we consider techniques to convert unstructured big data to structured time series suitable for nowcasting purposes. We also include several empirical examples which illustrate the potential of big data in economics. Finally, we provide a practical application based on textual data analysis, where we exploit a huge set of about 3 million news articles for the construction of an economic uncertainty indicator. Keywords: Big Data, Unstructured Data, Time Series Conversion, Data Features. Acknowledgement: This work has been carried out by George Kapetanios∗, Massimiliano Marcellino† and Fotis Papailias‡ for Eurostat under a contract with GOPA. The Eurostat project manager was Dario Buono∗∗. ∗ [email protected] † [email protected] ‡ [email protected] ∗∗[email protected] Big Data Conversion Techniques including their Main Features and Characteristics 3 Table of contents Table of contents Abstract ........................................................................................................................................................ 3 1 Introduction ................................................................................................................................. 8 2 Literature review ........................................................................................................................9 2.1 Data mapping ............................................................................................................................... 9 2.2 Recent research papers ...........................................................................................................10 2.2.1 Electronic payments data ...................................................................................................... 10 2.2.2 Mobile phone usage data ......................................................................................................11 2.2.3 Sensor data ........................................................................................................................... 12 2.2.4 Satellite images data ............................................................................................................. 13 2.2.5 Price data .............................................................................................................................. 14 2.2.6 Textual data .......................................................................................................................... 15 3 A general data conversion framework for unstructured numerical Big Data ..................................................................................................................16 3.1 Conceptual setting .....................................................................................................................16 3.2 Aggregation ................................................................................................................................. 17 3.3 Features extraction ....................................................................................................................18 3.4 Data mining ................................................................................................................................. 18 3.4.1 Random subsampling ...........................................................................................................21 4 Empirical examples ................................................................................................................26 4.1 Financial markets data .............................................................................................................26 4.2 Mobile phone usage data .........................................................................................................28 4.3 Sensor data ................................................................................................................................. 32 4.4 Online prices data ......................................................................................................................36 4.5 Online search data ....................................................................................................................38 4.6 Social media................................................................................................................................ 41 5 From Reuters News data to uncertainty indexes ......................................................... 44 5.1 Obtaining the data .....................................................................................................................44 5.2 Obtaining a list of links ..............................................................................................................45 5.3 Removing duplicates .................................................................................................................46 5.3.1 Removing broken links .......................................................................................................... 47 5.3.2 Removing duplicate URLs .....................................................................................................48 5.3.3 Removing duplicate headlines ..............................................................................................48 Big Data Conversion Techniques including their Main Features and Characteristics 4 Table of contents 5.4 Scraping new articles ................................................................................................................49 5.5 Article volume over time ...........................................................................................................50 5.6 Constructing the uncertainty index ........................................................................................51 5.6.1 Empirical estimator ................................................................................................................ 51 5.6.2 From word mentions to indexes ............................................................................................52 5.7 Indices .......................................................................................................................................... 52 6 Conclusions .............................................................................................................................. 53 References ................................................................................................................................................. 54 Appendix .................................................................................................................................................... 57 Big Data Conversion Techniques including their Main Features and Characteristics 5 List of tables List of tables Table 1: Comparing statistics of the original sample and the two random subsamples. 22 Table 2: Raw and cleaned data for a hypothetical security with ticker XXX. 27 Table 3: Example of mobile phone usage data. 29 Table 4: Sample data for GPS using mobile phones. 32 Table 5: Sample data of web scraped prices. 37 Table 6: Various correlated keywords to “gbpusd” as returned by Google Correlate. 40 Table 7: Sample Twitter data scraped from Twitter. 41 Table 8: Summary of de-duplication of articles. 46 Table 9: Dictionaries of keywords by index. 52 Big Data Conversion Techniques including their Main Features and Characteristics 6 List of figures List of figures Figure 1: General description of big data conversion ...................................................................
Details
-
File Typepdf
-
Upload Time-
-
Content LanguagesEnglish
-
Upload UserAnonymous/Not logged-in
-
File Pages62 Page
-
File Size-