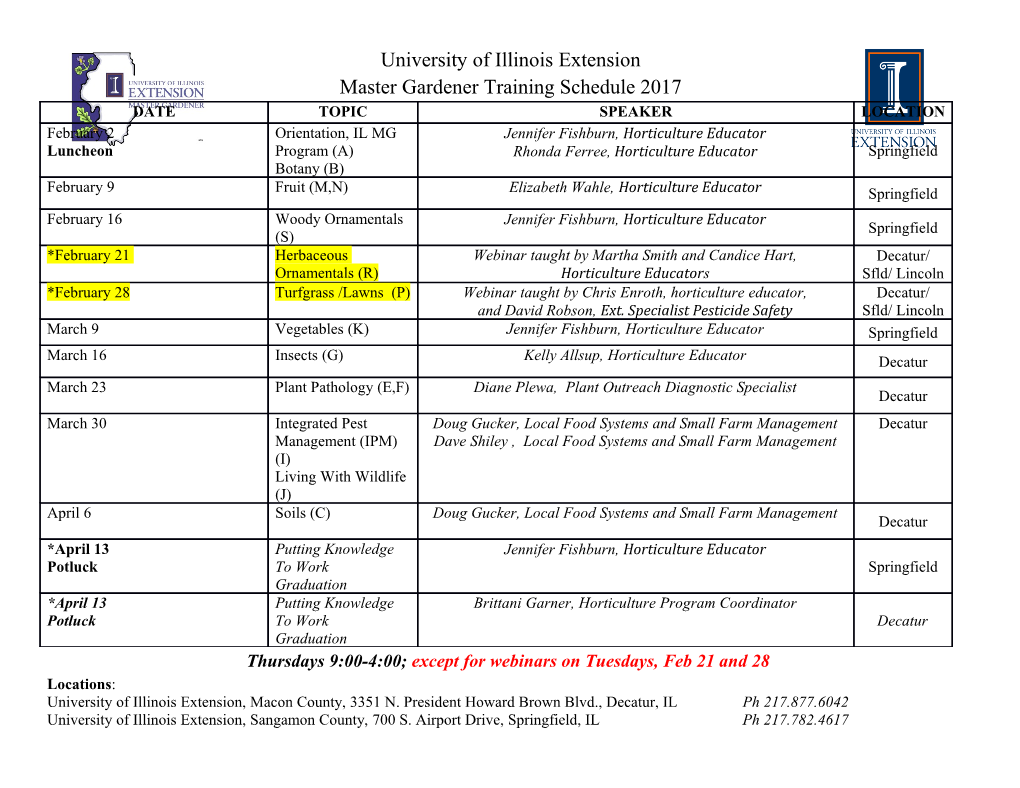
Central Annals of Biometrics & Biostatistics Bringing Excellence in Open Access Editorial *Corresponding author Huaizhen Qin, Department of Biostatistics and Bioinformatics Tulane University School of Public Health Exploit the Neglected and Tropical Medicine, New Orleans, LA 70112, USA, Tel: 504-988-2042; Fax: 504-988-1706; Email: Heteroscedasticity in Genetics Submitted: 05 December 2016 Accepted: 05 December 2016 Data Published: 08 December 2016 Copyright Huaizhen Qin* and Weiwei Ouyang © 2016 Qin et al. Department of Biostatistics and Bioinformatics, Tulane University School of Public Health and Tropical Medicine, USA OPEN ACCESS Recently, there have been emerging investigations on EDITORIAL heteroscedasticity in genetic association studies. Roughly The use of (generalized) linear regression is ubiquitous in speaking, there are two schools about how to deal with potential genetic association studies of complex phenotypes. In this area, homoscedasticity is often assumed, namely, the variances of considered as impediment to information mining, and data modelling errors are assumed to be invariant with respect to transformationheteroscedasticity. is employed In the tofirst eliminate school, data heteroscedasticity heteroscedasticity, is the effects being modelled. Such studies aim to localize causal i.e., log transformation and Box-Cox transformation. Sun et al. loci by exploiting the mean effects of genetic variants at the loci [12], formally demonstrated that biological interpretations that are in LD with the genuine causal loci (Figure 1), i.e., testing based on genotypedriven heteroscedasticity may not be valid when phenotypic means with respect to the three genotype for β1 = 0 under standard linear model Y = β0 + Gβ1+ e, assuming 2 homoscedasticity (σe , the variance of does not depend on G). groups are not equal. They theoretically showed that the variance The homoscedasticity assumption is often violated in real-world can be expressed as a quadratic function of the mean and a genetic association studies, more or less. Heteroscedasticity, aka, transformation can be employed to equalize the three variances variance heterogeneity, is ubiqitous, since LD is ubiquitous [1]. The for an autosomal diallelic SNP. The quadratic transformation is distribution of the modeling error in aforesaid linear regression apparently depends on the genuine causal variants and hence the there are only three possible genotypes. However, it may not observed genotypes at their immediate neighbor markers, i.e., worksufficient well to for eliminate set wise heteroscedasticity tests, e.g., the most for popular a single SKAT SNP, because[12] for 2 σe = h() G for some function h().. The heteroscedasticity problem identifying sequence associations. In general, it is hard to choose in genetic association studies was acknowledged at least as early a transformation to completely eliminate set (e.g., gene, pathway) as in 2000 but was historically neglected for simplicity [2]. In the driven heteroscedasticity and the induced bias in standard error era of deep sequencing, homoscedasticity is still assumed by vast most existent prominent marker wise and set wise association way to calibrate heteroscedasticityother than a way to directly tests [3-10]. As warned by Econometrician White [11], the exploitestimates it. of regression coefficients. Data transformation is one presence of heteroscedasticity can invalidate the statistical tests In the second school, heteroscedasticityis thought to represent that assume homoscedasticity. an important information resource other than an impediment for mining genetics data. Venables et al. [13], pointed out that when difference would often be more important than any difference in theirsamples means. show Variability-controlling a significant difference quantitative in their trait variances, loci (vQTLs) this [14-21] were reported as genetic variants whose allelic states are associated with phenotypic variability. vQTLs show evidence of potential highorder interactions including networks with other genes (gene-gene interactions) or environmental factors (gene- environment interactions), and may also implicate an increased variation over time among subjects in a given genotype group. Gene expression level is considered as a quantitative phenotype. Figure 1 Schematic diagram of association mapping. Genetic loci are Expression variability QTLs (evQTLs) [22] were reported as said to be in LD if some combinations of alleles occur at these loci loci whose allelic states are associated with variances of gene more or less frequently than expected from random formation [2]. A expression. Formation of evQTL would be due to gene-gene correlation between genotyped markers (G) and study trait (Y) would be observed if these markers are in LD with some QTLs (C). Therefore, interactions. The double generalized linear model (DGLM) [23] QTLs can be localized by their immediate . and the hierarchical generalized linear model (HGLM) [24- Cite this article: Qin H, Ouyang W (2016) Exploit the Neglected Heteroscedasticity in Genetics Data. Ann Biom Biostat 3(1): 1026. Qin et al. (2016) Email: Central Bringing Excellence in Open Access 26] have been proposed to incorporate mean and dispersion components of testing variants and adjusting for covariates. The DGLM is an extension of a classical heteroscedastic regression model [27] incorporating heteroscedasticity within dispersion component. The HDGLM is a direct extension of the DGLM to avoid misinterpretation of detected Heteroscedasticity [19,28] Dueallow to both various fixed latent and predictors,random effects. detected Caution heteroscedasticity should be used may to not necessarily indicate strong gene-gene or gene-environment interactions. Figure 2 Schematic diagram of admixture mapping. Within a CAB, all Aforesaid association methods were designed virtually for genetics data from homogeneous populations. Before this its immediate neighbor loci. Local ancestriesA of the block directly editorial, admixture mapping was thought to come of age [29] impactsloci are in the ALD, genotypic and a specific distributions causal locusof all inthe C blockcan also wide be lociin BLD and with the but no publication addressed heteroscedasticity in admixture distribution of global ancestriesQ; and (Q, A) can be associated with mapping regime. We argue that exploiting ancestry driven some of all other causal factors (X), which directly or indirectly impact the distribution of Y. mapping. The genomes of admixed subjects derive from 1 + kheteroscedasticitywill distinct ancestral populations have significant (k relevance to admixture window included 100 SNPs as recommended by the package of segments (aka, admixture blocks) with various ancestries (ethnic origins). For example, genomes ≥ 1) of andAfrican thus Americans are mosaics are formed from recent admixture of West African and European surrogatesdocument. Then,and computed we fitted thethe DGLMcalibrated [23] ofresiduals drinking of symptoms drinking ancestries (k = 1). There are three LD sources in admixed symptoms.on age, gender, Next, atthe each first window, 10 global we dividedPCs as theglobal subjects ancestry into genomes [30] Admixture LD (ALD) occurs between the loci three groups according to their window wise European ancestries within each admixture block due to coherence in local ancestries. and inspected local ancestry driven heteroscedasticity by the Background LD (BLD) is the traditional LD inherited from their Levene’s test [40]. We implemented such a two-stage procedure homogeneous ancestral populations. Mixture LD (MLD) occurs because the existent dglm function [41] did not converge when among loci across the entire mosaic genomes due to variation jointly modeling both local ancestries and aforesaid predictors. in global ancestries. ALD has long been exploited for identifying A couple of clear peaks in –log10 (P ) emerged (Figure 3), where causal admixture blocks (CABs) that harbor causal alleles with the signals of heteroscedasticity appeared much stronger than distinct ancestral frequencies [29,31-33] that of the corresponding mean effects. In this analysis, the two pieces of information track each other consistently. Namely, According to our and others’ genetic studies in admixed the local ancestries at CABs simultaneously impact conditional populations [34-37], we depict the generic causal network phenotypic mean and conditional phenotypic variances for the between trait and its causal factors within and out of a given predictors. By this example, heteroscedastic admixture CAB (Figure 2). For each admixed subject , local ancestries mapping appears to be a novel and useful complementary tool for =,, … are the same for all loci within the CAB, where AAAi() i1 ik localizing genetic causal variants in the era of deep sequencing. Aij ∈{}0,, 1 2 stands for the number of marker wise alleles from the jth ancestral population(,,)j=1 … k ; and global ancestries In a broad sense, agenetic variant that alters arbitrary moments of the distribution of a study phenotype can be called a QQQi=(,,) i1 … ik is the average of local ancestries across the entire genome. C (G) is the set of all causal (neutral) loci within genetic causal variant for that phenotype. Due to the complexity the CAB. X is the set of all the other causal factors, e.g., other of biological mechanisms, the conditional distribution of a study causal loci across the genome, environmental factors, and phenotype for given predictors cannot be completely determined latent pleiotropic
Details
-
File Typepdf
-
Upload Time-
-
Content LanguagesEnglish
-
Upload UserAnonymous/Not logged-in
-
File Pages4 Page
-
File Size-