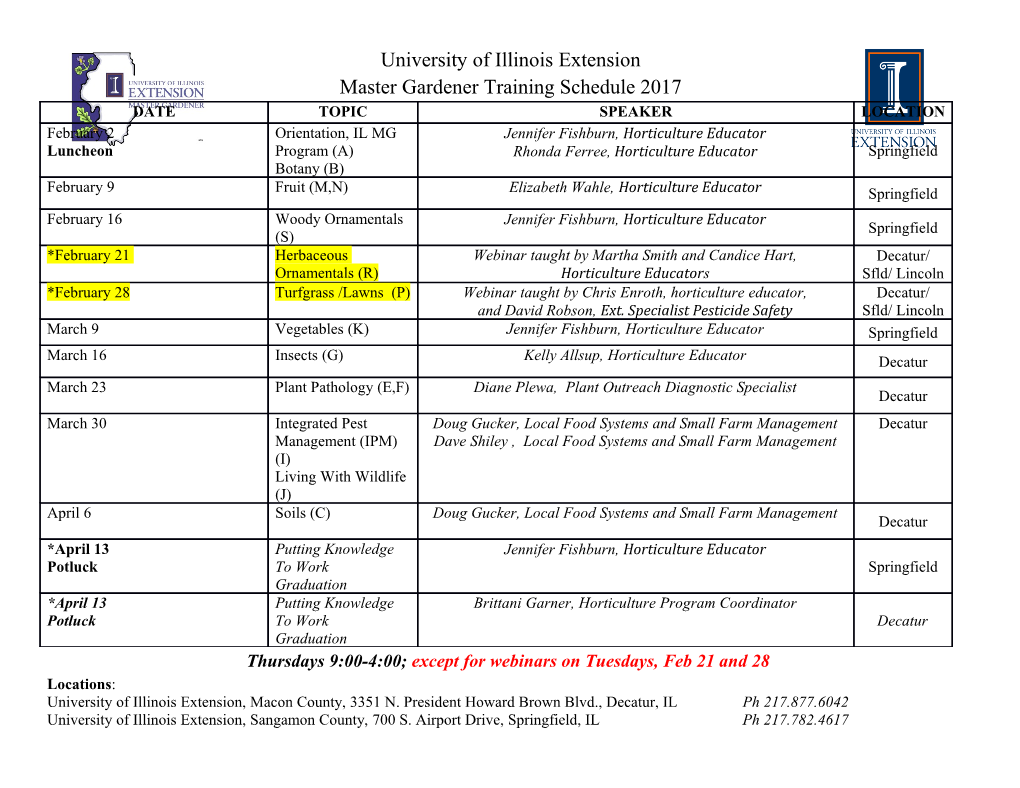
Urban Rail Transit (2019) 5(1):1–16 https://doi.org/10.1007/s40864-018-0100-x http://www.urt.cn/ ORIGINAL RESEARCH PAPERS Open-Source Public Transportation Mobility Simulation Engine DTALite-S: A Discretized Space–Time Network-Based Modeling Framework for Bridging Multi-agent Simulation and Optimization 1,2 3 4 5 5 5,6 Lu Tong • Yuyan Pan • Pan Shang • Jifu Guo • Kai Xian • Xuesong Zhou Received: 24 November 2018 / Revised: 8 December 2018 / Accepted: 17 December 2018 / Published online: 21 January 2019 Ó The Author(s) 2019 Abstract Recently, an open-source light-weight dynamic dynamic representation details in DTALite for future traffic assignment (DTA) package, namely DTALite, has extensions. We hope to offer a unified modeling framework been developed to allow a rapid utilization of advanced with inherently consistent space–time network representa- dynamic traffic analysis capabilities. Aiming to bridge the tions for both optimization formulation and simulation modeling gaps between multi-agent simulation and opti- process. This paper includes three major modeling com- mization in a multimodal environment, we further design ponents: (1) mathematic formulations to describe traffic and develop DTALite-S to simplify the traffic flow and public transportation simulation problem on a space– time network; (2) transportation transition dynamics involving multiple agents in the optimization process; (3) & Yuyan Pan an alternating direction method of multipliers (ADMM)- [email protected] based modeling structure to link different features between Lu Tong multi-agent simulation and optimization used in trans- [email protected] portation. This unified framework can be embedded in a Pan Shang Lagrangian relaxation method and a time-oriented [email protected] sequential simulation procedure to handle many general Jifu Guo applications. We carried out a case study by using this [email protected] unified framework to simulate the passenger traveling Kai Xian process in Beijing subway network which contains 18 [email protected] urban rail transit lines, 343 stations, and 52 transfer sta- Xuesong Zhou tions. Via the ADMM-based solution approach, queue [email protected] lengths at platforms, in-vehicle congestion levels and 1 School of Electronic and Information Engineering, Beihang absolute deviation of travel times are obtained within 1560 University, Beijing, China seconds.The case study indicate that the open-source 2 National Engineering Laboratory for Comprehensive DTALite-S integrates simulation and optimization proce- Transportation Big Data Application Technology, Beijing, China dure for complex dynamic transportation systems and can 3 Beijing University of Technology, Beijing, China efficiently generate comprehensive space-time traveling status. 4 Department of Civil Engineering, Tsinghua University, Beijing, China Keywords Space–time network Á Dynamic traffic 5 Beijing Transport Institute, No. 9 LiuLiQiao South Road, Fengtai District, Beijing, China assignment Á Multi-agent simulation Á Lagrangian relaxation Á Alternating direction method of multipliers 6 School of Sustainable Engineering and the Built Environment, Arizona State University, Tempe, USA Communicated by Lixing Yang. 123 2 Urban Rail Transit (2019) 5(1):1–16 1 Introduction service model and estimate the benefits of sharing vehicles. Focusing on modeling the microscopic behavior in virtual To understand and analyze future emerging mobility sce- reality systems, Yu et al. [9] provided a hierarchical modular narios, planers and engineers need to utilize many different modeling and distributed simulation methodology. A con- simulation tools to generate corresponding modeling cise overview of simulation-based transportation analysis results. The main purpose of transportation simulation is to approaches is offered by Bierlaire [10]. shed more light on the underlying mechanisms or potential Transportation researchers have devoted significant problems that control the behavior of a complex trans- attentions to both traffic and public transportation simula- portation system. tion models. Recently, Bradley et al. [11] conducted pos- Typically, simulating a system involves a probabilistic sible autonomous vehicle (AV) operating scenarios in a input model, a set of dynamic equations or constraints road network system, and further modeled the metro transit between the inputs and outputs, and then finally produces a station as a finite capacity queuing system through a dis- set of outputs under different input instances. Optimization, crete-event simulation (DES) approach, which was also on the other hand, needs to search a solution in the dynamic adopted in the study by Afaq et al. [12]. Liang et al. [13] (possibly complex) system subject to a number of con- provided a mathematical model to consider the door-to- straints. There are a wide range of studies focusing on door intermodal travel trips and found that the vehicle fleet simulation-based optimization, to name a few, a leading size directly influences the performance of the taxi system. study by Osorio et al. [1] involving stochastic urban traffic Mahmassani [14] integrated varying behavioral mecha- simulators, and another study by Xiong et al. [2] using the nisms for different classes of vehicles into a microsimu- DTALite simulator. Generally, transportation planners and lation framework through a series of experiments under engineers utilize simulation tools to evaluate and further varying market penetration rates of AVs and/or connected optimize a subset of system’s parameters, but there is a vehicles. Qu et al. [15] presented a computationally effi- critical modeling gap between simulation and optimization cient parallel-computing framework for real-life traffic for complex dynamic transportation systems. To bridge such simulation for metropolitan areas. To meet simulation a gap in a multimodal environment, this research focuses on accuracy requirements, Martinez et al. [16] proposed an how to offer a theoretically sound and practically useful agent-based model to simulate individual daily mobility modeling framework with a simplified traffic flow dynamic. while traffic assignment conditions are updated every 5 min. Golubev et al. [17] presented an agent-based traffic 1.1 Literature Review modeling framework allowing users to set a specific model for each supported class. Sun et al. [18] presented an agent- Scheduling vehicles on congested transportation networks based simulation for urban rail transit systems. Based on needs to consider both traffic flows with vehicle-to-road kinematic wave model, Wen et al. [19] implemented a assignment and vehicle routing problem (VRP) with pas- shared autonomous mobility-on-demand (AMoD) model- senger-to-vehicle matching. There are numbers of studies ing platform for simulating individual travelers and vehi- about agent-based traffic assignment and traffic simulation. cles with demand–supply interaction and analyzing the Mahmassani et al. [3] used flow-density relationships to system performance through various metrics of indicators. predict time-dependent traffic flows in the Dynamic Net- Recently, there are many papers focusing on vehicle work Assignment-Simulation Model for Advanced Road- routing optimization models and algorithms used in large- way Telematics (DYNASMART). From a broader multi- scale optimization. Boyd et al. [20] discussed general dis- agent optimization perspective, in the study by Nedic et al. tributed optimization and provided efficient implementa- [4], a distributed computation model is built for optimizing a tion under the non-convex setting. Mahmoudi and Zhou sum of convex objective functions for all types of agents. [21] built the state-space–time network to model the For shared autonomous vehicle (SAV) operating, Fagnant vehicle routing problem with pickup and drop-off and with et al. [5] proposed an agent-based shared autonomous time windows (VRPPDTW). Based on the Lagrangian vehicle relocation model in order to reduce potential users’ decomposition and space–time windows, Tong et al. [22] wait times. Following Newell’s kinematic approach [6], developed a joint optimization approach for customized Zhou and Taylor [7] designed a mesoscopic traffic simula- bus services. Wei et al. [23] developed a set of integer tion approach and developed a time-driven open-source programming and dynamic programming models to opti- traffic assignment package DTALite to simulate large-scale mize simplified multi-vehicle trajectories. Zhou et al. [24] networks with millions of vehicles. Based on the multi- introduced a vehicle routing optimization engine VRPLite source data generated from transportation network compa- on the basis of a hyper space–time–state network repre- nies, Spieser et al. [8] provide an on-demand transportation sentation with an embedded column generation and Lagrangian relaxation framework. Zhao et al. [25] 123 Urban Rail Transit (2019) 5(1):1–16 3 considered an optimization framework for electric vehicles in the context of public transportation network optimiza- in the one-way carsharing system, and they proposed a tion, (b) how to use agent-based simulation tools to eval- Lagrangian relaxation-based solution approach to decom- uate travel schedules with limited road capacity, and pose the primal problem. (c) how to improve system flexibility and accessibility with a consolidation of modes. To meet these challenges, this 1.2 Paper Structure paper considers the vehicle route scheduling optimization in the broader framework of public transportation and The remainder
Details
-
File Typepdf
-
Upload Time-
-
Content LanguagesEnglish
-
Upload UserAnonymous/Not logged-in
-
File Pages16 Page
-
File Size-