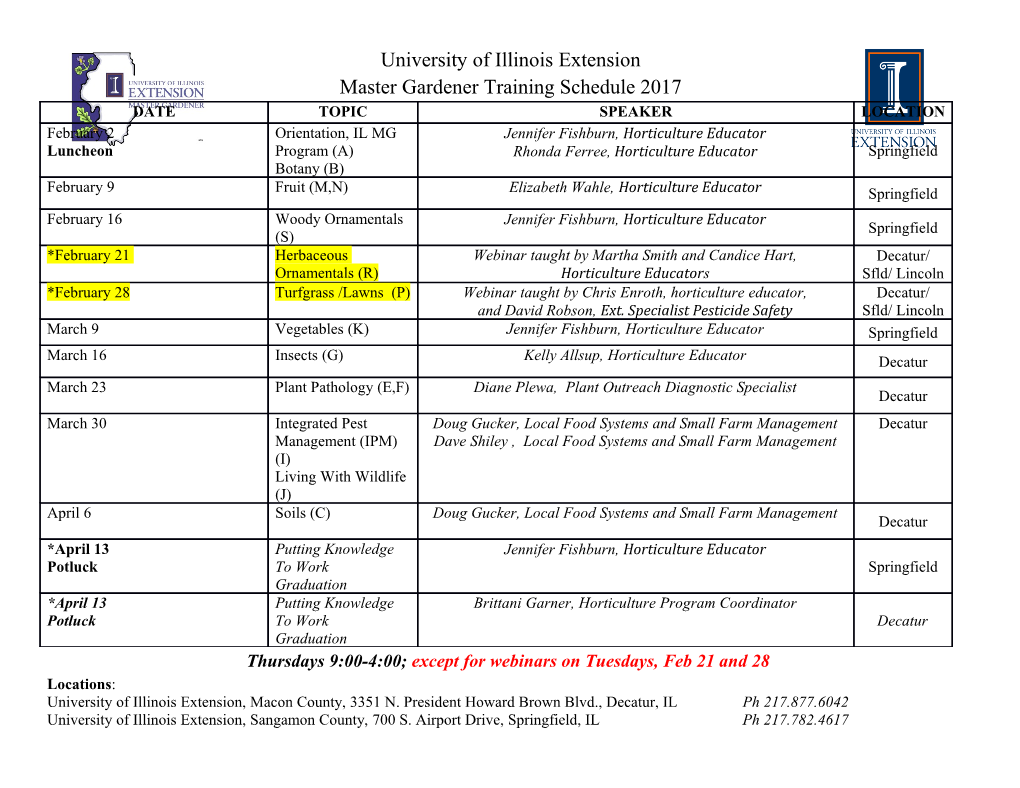
hydrology Article Predicting Runoff Chloride Concentrations in Suburban Watersheds Using an Artificial Neural Network (ANN) Khurshid Jahan and Soni M. Pradhanang * Department of Geosciences, University of Rhode Island, 315 Woodward Hall, 9 E. Alumni Ave, Kingston, RI 02881, USA; [email protected] * Correspondence: [email protected] Received: 26 August 2020; Accepted: 13 October 2020; Published: 21 October 2020 Abstract: Road salts in stormwater runoff, from both urban and suburban areas, are of concern to many. Chloride-based deicers [i.e., sodium chloride (NaCl), magnesium chloride (MgCl2), and calcium chloride (CaCl2)], dissolve in runoff, travel downstream in the aqueous phase, percolate into soils, and leach into groundwater. In this study, data obtained from stormwater runoff events were used to predict chloride concentrations and seasonal impacts at different sites within a suburban watershed. Water quality data for 42 rainfall events (2016–2019) greater than 12.7 mm (0.5 inches) were used. An artificial neural network (ANN) model was developed, using measured rainfall volume, turbidity, total suspended solids (TSS), dissolved organic carbon (DOC), sodium, chloride, and total nitrate concentrations. Water quality data were trained using the Levenberg-Marquardt back-propagation algorithm. The model was then applied to six different sites. The new ANN model proved accurate in predicting values. This study illustrates that road salt and deicers are the prime cause of high chloride concentrations in runoff during winter and spring, threatening the aquatic environment. Keywords: stormwater; artificial neural network (ANN); water quality; road salts; deicers 1. Introduction Urban areas require the construction of buildings, roads, and parking areas, yet such urban development causes hydrologic impacts and pollution as pervious surfaces are made impervious [1]. For safety, given abundant snowfall during the winter season, most communities in New England use salt or deicing on roads and parking areas. Road salts or deicing during the winter season are the primary factors for increasing salinity in surface soils, surface water, groundwater, and runoff. In the USA, an average of 24 million metric tons of road salt is applied each year to roads [2]. It is well-established that the application of road salts leads to the accumulation of sodium and chloride in soils and surface waters [3–5], with adverse impacts on downstream aquatic ecosystems [6]. In fact, when impervious surface areas increase, the areas that need to be deiced also increase. Chloride-based deicers [i.e., sodium chloride (NaCl), magnesium chloride (MgCl2), and calcium chloride (CaCl2)] dissolve in runoff, percolate into soils, and leach into groundwater. Chloride from chloride-based deicers does not efficiently precipitate or biodegrade but is absorbed by mineral/soil surfaces [7]. Although winter road deicing is an essential service for urban areas in USA (especially in the upper Midwest and Northeast), it contributes to a significant increase in chloride concentration [8]. The United States Geological Survey (USGS) (2014) conducted a temporal, seasonal, and environmental analysis of chloride concentrations in urban areas and assessed effects on water quality and the environment, especially on aquatic organisms across the USA [8]. This study concluded that there is an increasing trend of high chloride concentrations in urban areas due to expansion of impervious cover that requires deicing. Hydrology 2020, 7, 80; doi:10.3390/hydrology7040080 www.mdpi.com/journal/hydrology Hydrology 2020, 7, x FOR PEER REVIEW 2 of 17 Hydrology 2020, 7, 80 2 of 17 study concluded that there is an increasing trend of high chloride concentrations in urban areas due to expansion of impervious cover that requires deicing. An increasing trend in chloride concentrations in some US rivers is shown in Figure1 and attributed An increasing trend in chloride concentrations in some US rivers is shown in Figure 1 and to increasedattributed usageto increased of road usage salt; of the road trend salt; is the positive trend inis positive New England in New (USGS, England 2014). (USGS, The 2014). increasing The salinityincreasing not only salinity threatens not only aquatic threatens ecosystems, aquatic ecosystems, but also contributes but also contributes to corrosion to incorrosion water distribution in water systems.distribution Salinity systems. can increase Salinity even can with increase little snowfall even with due little to effi snowfallcient transfer due to of chlorideefficient totransfer wastewater of dischargechloride or to septicwastewater systems discharge [8]. Areas or septic that havesystems no [8]. snow, Areas such that as have Florida, no snow, also showed such as increasingFlorida, salinity.also showed In the caseincreasing of Florida, salinity. less In than the case normal of Florida, rainfall less since than 1990 normal combined rainfall with since groundwater 1990 combined level declinewith duegroundwater to over pumping level decline explain due to the over observed pumping increasing explain the salinity observed [9–11 increasing]. The characteristics salinity [9–11]. and degradationThe characteristics of urban and runo degradationff quality and of itsurban impact runoff on thequality environment and its impact largely on depend the environment on the urban land-uselargely practices, depend on site the geology, urban land-use and hydrogeology. practices, site The geology, large quantity and hydrogeology. of road salt The that large is applied quantity every yearof forroad snow salt removalthat is applied in the every USA year is one for of snow the major removal contributors in the USA to is decliningone of the stormwater major contributors quality. to declining stormwater quality. FigureFigure 1. 1.Chloride Chloride concentration concentration trendstrends from 1992 to to 2012 2012 in in the the USA USA show show regional regional differences differences (modified(modified from from United United States States Geological Geological Survey Survey Report,Report, 2014).2014) Recently,Recently, several several studies studies have have applied applied artificial artificial neural neural network network (ANN) (ANN) methods methods to predict to predict resulting waterresulting quality water based quality upon based input upon variables input variables [12]. Since [12]. 1990, Since ANN 1990, hasANN been has appliedbeen applied in many in many fields, includingfields, including environmental environmental sciences, sciences, ecological ecological sciences, sciences, and water and engineering water engineering [13]. According [13]. According to Haykin (1999)to Haykin [14], ANN (1999) is [14], highly ANN capable is highly in modeling capable innonlinear modeling systemnonlinear estimation system estimation and is highly and is adaptable. highly ANNadaptable. allows preciseANN allows predictions precise of predictions the target parameterof the target for parameter specific materialsfor specific or materials stages [14 or,15 stages]. [14,15].In this study, an ANN model is developed with a back-propagation algorithm. The back-propagation algorithmIn incorporatesthis study, highlyan ANN nonlinear model relationshipsis developed [ 15with]. The a ANNback-propagation model was developed algorithm. for The rapid calculationback-propagation and prediction algorithm of selectedincorporates water highly quality nonlinear variables relationships at any location [15]. The of interest.ANN model Within was the model,developed unknown for rapid parameter calculation weights and areprediction adjusted of toselected obtain water the best quality correlation variables between at any location appropriate of interest. Within the model, unknown parameter weights are adjusted to obtain the best correlation input parameters or a historical set of model inputs and the corresponding outputs [16]. This study between appropriate input parameters or a historical set of model inputs and the corresponding provides the ANN modeling method needed to simulate and forecast chloride concentrations in runoff. outputs [16]. This study provides the ANN modeling method needed to simulate and forecast The aim of the study is to (i) develop an ANN model of the system trained using a small data set, chloride concentrations in runoff. The aim of the study is to (i) develop an ANN model of the system (ii)trained obtain theusing best-fit a small models data set, for predicting(ii) obtain the chloride best-fit concentrations models for predicting using data chloride from concentrations monitoring sites, (iii)using evaluate data the from ANN monitoring model performance sites, (iii) evaluate using 3the years ANN (2016–2019) model performance of observed using data 3 versus years predicted(2016– data2019) from of theobserved model, data and versus (iv) determine predicted data the accuracyfrom the model, of the and ANN (iv) model determine performance. the accuracy The of modelthe also assesses the impact of road salt applications through assessment of a spatial density distribution focused on probable high chloride concentration in an area. Hydrology 2020, 7, x FOR PEER REVIEW 3 of 17 Hydrology 2020, 7, x FOR PEER REVIEW 3 of 17 ANN model performance. The model also assesses the impact of road salt applications through Hydrology 2020, 7, 80 3 of 17 assessmentANN model of performance.a spatial density The distributionmodel also focusedassesses onthe probable impact ofhigh road chloride salt applications concentration through in an area.assessment
Details
-
File Typepdf
-
Upload Time-
-
Content LanguagesEnglish
-
Upload UserAnonymous/Not logged-in
-
File Pages17 Page
-
File Size-