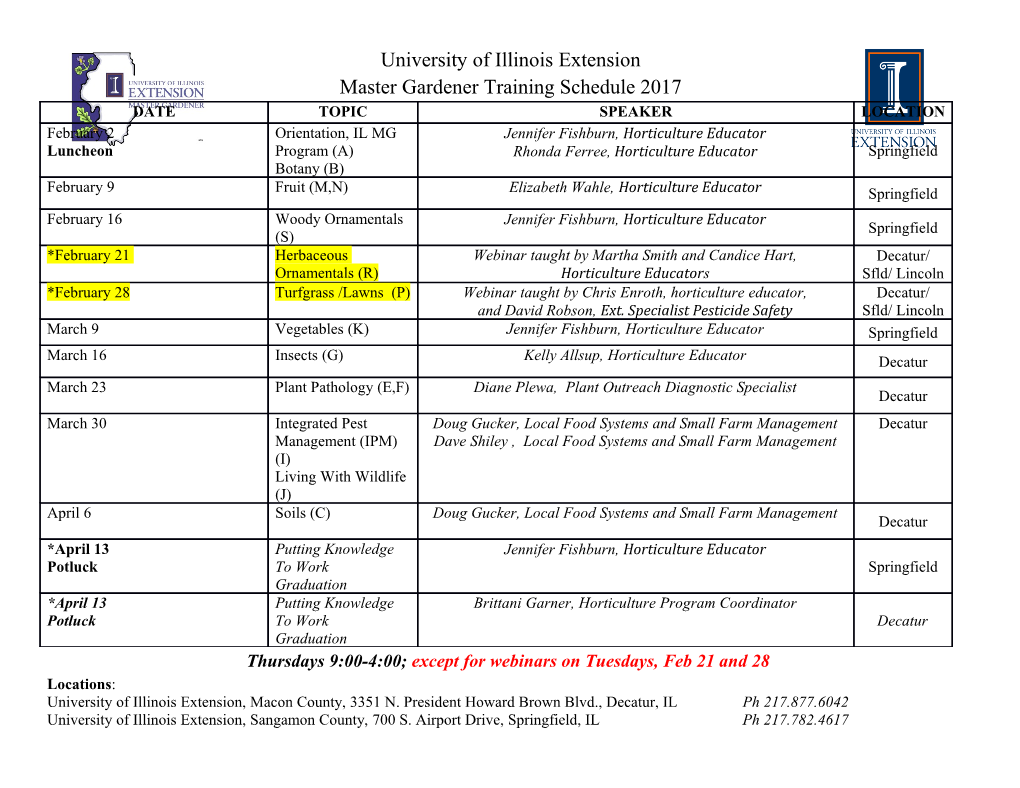
E3S Web of Conferences 251, 03009 (2021) https://doi.org/10.1051/e3sconf/202125103009 TEES 2021 Changes in the spatial and temporal characteristics of inbound tourism flows in Tibet based on geotagged photographs HuaJian Gao1, a, NaiXia Mou1, b* 1 College of Geomatics, Shandong University of Science and Technology, Qingdao 266590, China Abstract: With the further advent of the era of big data, the scale of social media data containing geolocation information is exploding, providing a new source of big data information and perspective for an in-depth study of the changing spatio-temporal and geographical characteristics of the current tourist population. This paper extracts data on popular attractions in the Tibet Autonomous Region using the HDBSCAN algorithm combined with the TF-IDF algorithm based on information on images with geotags shared by users in the Flickr image sharing site from 2005-2018. Social network analysis was used to explore the changes in the spatial and temporal characteristics of inbound tourism flows in Tibet. The results show that: (1) in terms of temporal characteristics, the number of inbound tourists shows obvious off-peak seasons, with relatively high sensitivity to the influence of economic, policy and infrastructure construction factors; (2) in terms of spatial distribution characteristics, the inbound tourism flow in Tibet shows an "axis-scattered" distribution. The core area is centred on Lhasa and extends in three directions: west, north and east along important roads. temporal information such as check-in times and latitude 1 Introduction and longitude, making it an ideal data source for studying inbound tourism flows[7]. Inbound tourism is an important part of China's tourism In this paper, based on geotagged photo information market and an important indicator of the country's tourism [1] from Flickr, we use the HDBSCAN algorithm to extract competitiveness . The flow pattern of inbound tourists tourism AOI and use the TF-IDF algorithm to constrain between tourism hotspots can reflect the dynamic trend of the clustering results according to geotags, solving the the international tourism source market, and is also problem of multiple tourism points in tourism AOI important for the development of tourism markets and [2] extraction. On this basis, social network theory is tourism products . introduced to explore the structure of spatial relationships Research on inbound tourism flows usually revolves [3] and the dynamic evolution process of inbound tourism around traditional data, such as statistical yearbooks and flows. questionnaires[4], which can reflect changes in the number of inbound tourists in a certain region and time, but often fail to accurately and comprehensively reflect the spatial 2 Materials and Methods distribution and flow characteristics of tourists[5]. The sample representativeness of survey questionnaires is poor, 2.1 Study area and dataset and it is also difficult to accurately and comprehensively reflect the behavioural characteristics of inbound Located in the west and south of China's Tibetan Plateau, [6] tourists . With the increasing popularity and rapid with an average altitude of more than four kilometres industrial development of information technology of above sea level and an area of 1,228,400 square kilometres, mobile application terminals such as modern mobile smart Tibet has a resident population of 3,348,200 as of 31 Internet and mobile phone intelligence, it has become December 2018[8]and is the richest and least developed possible and trendy to use social media data containing region in China in terms of tourism resources, with the geographical location information to study tourism least resources being infringed upon and subject to any [7] flows . artificial damage[9]. The geographical isolation has given Flickr, Panoramio, Instagram and a host of other photo Tibet a unique cultural history and tradition that has social applications allow users to share photos to online created a strong tourism attraction for modern travellers, communities and add location information to them using especially from foreign countries. Between 2005 and 2018, tags, hence the name geotagged photos. the Flickr website Tibetan inbound tourists increased from 120,000 to has over 49 million geotagged photos. This social media 470,000, an average annual growth rate of 21.1%, and data contains not only descriptive textual information such foreign exchange earnings from international tours as captions, messages and tags, but also spatial and increased from US$44.43 million to US$247.09 million, [email protected] * Corresponding author: [email protected] © The Authors, published by EDP Sciences. This is an open access article distributed under the terms of the Creative Commons Attribution License 4.0 (http://creativecommons.org/licenses/by/4.0/). E3S Web of Conferences 251, 03009 (2021) https://doi.org/10.1051/e3sconf/202125103009 TEES 2021 an average annual growth rate of 90.8%[10] Flickr (https://www.flickr.com/) is a widely used web- based photo management and sharing site worldwide. officially founded in 2004, the openness of Flickr's vast amount of social photo data has also made it one of the more recognised data collections in social photo research today. the Flickr website provides a free API interface for people to Flickr provides a free API for accessing its data. This paper crawls geotagged Flickr images and metadata in the Tibet Autonomous Region (78.3598°E~99.1146E, 27.2744°N~36.4619°N) from 1 January 2005 to 30 December 2018 through the Flickr API interface. The total Fig 1. Tibet Flickr photo distribution number of records is 95,321. As shown in Table 1 Table1. Flickr example data 2.3 Travel AOI Extraction The extraction of tourism AOIs (Area of Interest) from UserID PictureID Lat Lon P_date geotagged social media data is a prerequisite for studying 10003439 2011/10/1 the spatial and temporal variation of tourism flows. An 1000343991 31.868139 88.759407 9@N08 9 9:04 AOI usually consists of multiple POIs (Points of Interest), 10003439 2011/10/1 and unlike administrative divisions with clear boundaries, 1000343992 31.868139 88.759407 [15] 9@N08 9 9:24 the boundaries of tourism AOIs are mostly fuzzy so tourism researchers often use clustering algorithms to 10008272 2016/3/27 [16] 1000827201 29.653363 91.116861 calculate the boundaries of AOIs . Clustering algorithms 0@N07 10:22 are a method of classifying and categorizing data using similarities between objects. Traditional clustering 2.2 Data Cleaning algorithms mostly use density and distance-based methods to extract AOIs, such as the K-means algorithm and K- Flickr photo data may have some errors due to user device modes algorithm for distance-based clustering; the accuracy problems. Therefore, the following rules were DBSCAN algorithm and Mean-Shift algorithm for used to clean the data in this paper: (1) Removing density-based clustering. However, traditional clustering duplicate data. When obtaining Flickr data through the algorithms based on density and distance also have certain API interface, the data was obtained by chunking limitations. The core of these clustering algorithms is according to latitude and longitude, and duplicate crawled Single Linkage Clustering, which is very sensitive to noise, data would inevitably appear at the junction of each chunk and a noisy data point that lies between two class clusters of data, so these duplicate data needed to be cleaned, and may cause the class clusters to stick together and thus be a total of 631 data were removed. (2) Removal of resident classified into one class. However, the distribution of population data, part of the photos may be taken by social media data in geographic space is complex and residents based in Tibet[11, 12], and the length of stay in a often contains a large amount of noisy data. Carlos et al. place can be used as a criterion for judging tourists or use the HDBSCAN (Hierarchical Density-Based Spatial residents. girardin et al. set the threshold value to 30 Clustering of Applications with Noise) algorithm, which days[13], and this criterion has been adopted by most combines the idea of hierarchical clustering with density studies[14], this paper also adopts the threshold value of clustering to solve the problem of traditional clustering thirty days, and the two photos taken more than thirty days algorithms. that solves the class-cluster adhesion and apart users were excluded. A total of 7435 items were multi-density clustering problems that exist in traditional removed. (3) Removal of duplicate data. If the same user clustering algorithms[17]. took multiple photos at the same location and within one hour, the data were excluded as duplicate photos taken by 2.3.1 HDBSCAN algorithm the same user. A total of 12,037 pieces of data were removed. (4) Removal of orphaned data, as the aim of this The HDBSCAN algorithm idea is a combination of the paper is to study inbound tourism flows, so tourists with ideas of hierarchical clustering and density clustering, at least 2 shooting locations were filtered in order to build which proposes the concept of mutual reachable distances a network of inbound tourism flows. A total of 3858 pieces and generates a tree diagram by means of a mutual of data were removed. After cleaning, a total of 71,360 reachable distance matrix, thus achieving the separation of records from 1576 tourists were collected. The distribution clustering results of different densities from sparse noise. is shown in Figure 1. The mutual reachable distance is defined as: ��푎 � (1) Where��푎 � � ��� denotes��� the�푎 radius�� of� 푎clustering �푎� � of in the DBSCAN algorithm subject to the minimum � clustering parameter �� , and denotes the Euclidean distance between a and b. Under this metric, dense points �푎 � 2 E3S Web of Conferences 251, 03009 (2021) https://doi.org/10.1051/e3sconf/202125103009 TEES 2021 remain at the same distance from each other, but sparse 2.3.2 Geotagging-based merging of class clusters points are pushed away from the core distance of other points, effectively separating clusters from sparse noisy In order to solve the problem that different clusters of points.
Details
-
File Typepdf
-
Upload Time-
-
Content LanguagesEnglish
-
Upload UserAnonymous/Not logged-in
-
File Pages7 Page
-
File Size-