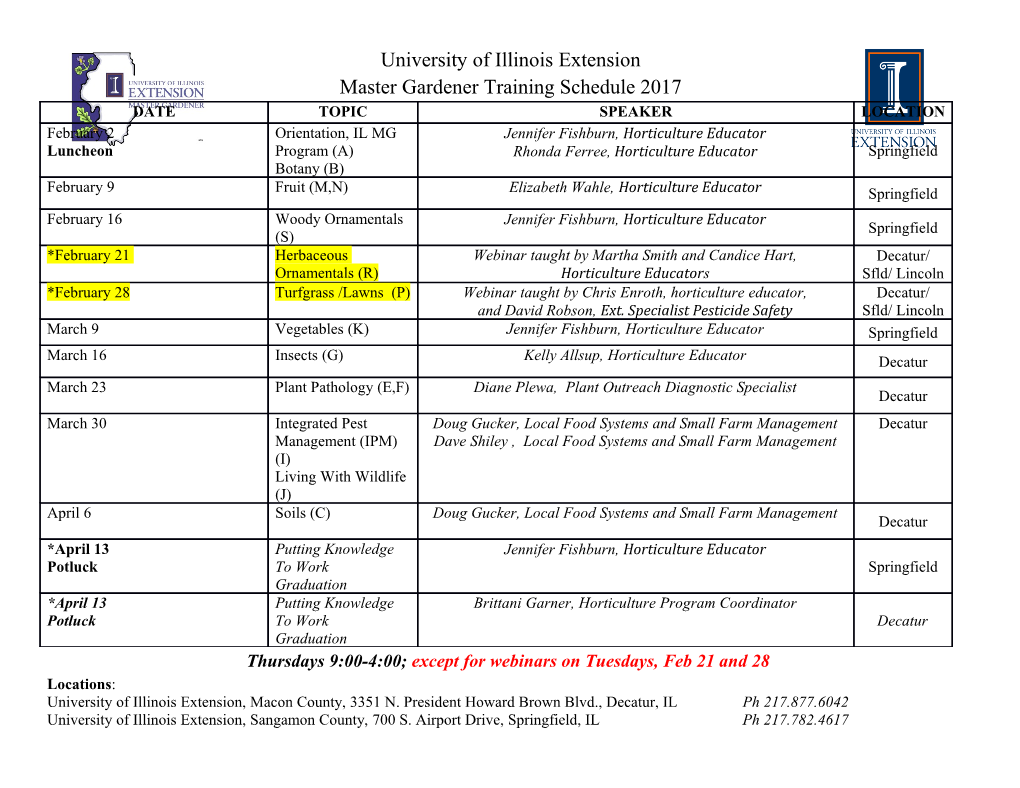
International Journal of Applied Environmental Sciences ISSN 0973-6077 Volume 8, Number 18 (2013), pp. 2295-2307 © Research India Publications http://www.ripublication.com/ijaes.htm Regional Agriculture Drought Risk Assessment based on T-S Fuzzy Neural Network Xu Zhengmina, b, Wang Fuqiangb, Han Yupingb, 1, Zhang Gongjinb and Wang Jingb a College of Water Resources and Architectural Engineering, Northwest A & F University, Yangling 712100, China b College of Water Resources, North China University of Water Resources and Electric Power, Zhengzhou 450045, China Abstract Agricultural drought is a mainly limited factor of the agricultural production in China. It’s very important for agricultural divisions, agricultural scheduling and crop production etc. to set up a suitable index system on the agricultural drought risk and evaluate scientifically the agricultural drought. The index system on the agricultural drought risk assessment based on society, economy and ecology etc. is built in the paper. And the evaluation model on the agricultural drought risk based on T-S fuzzy neural network is made, and is applied in Henan province. The results show that there are a remarkable increase on the agricultural drought risk in the north-east region and the east one in Henan province in recent ten years, while there is no apparent change in the south-west region and the south one. Especially, there are much bigger inter-annual changes on the agricultural drought risk in Xinyang and Shangqiu, while the ones in Zhengzhou, Anyang and Sanmenxia are much more smoothly. And all of the results can make a reference for the agricultural drought disasters fighting. Keywords: agriculture drought, index system, T-S fuzzy neural network, Henan province 1 Corresponding author. Address: North China University of Water Resources and Electric Power, Jinshui District, Beihuan Road 36,Zhengzhou 450045,China. Tel./fax:+86 37186549253. E-mail address: [email protected] (Han Yuping). 2296 Xu Zhengmin et al Introduction Drought is a kind of natural disasters caused by short-term climatic anomaly, and high frequency of occurrence, big scope of influence, long lasting time and great damage are all its characters [1].Drought is regarded as one of the natural disasters influencing directly on social economy, agricultural production and human life among all the extreme hydro meteorology events [2]. The conventional view is that drought is a natural disaster. However, drought is a factor causing drought damage, so regional drought damage is a natural risk. With much more influences of human activity on nature, unsuitable water resources exploration and usage and water pollution also directly affect drought. Risk is caused all together by uncertainty and loss or benefit, and the benefit is not concerned in the drought risk. So the risk can be simply defined as the uncertain of drought loss, which is composed of the uncertainty of drought loss occurring and ones of drought loss magnitude. In fact, the viewpoint of regional disaster systems is that drought is a factor causing drought risk, and drought range in space and time will affect the scale of drought risk. As a matter of fact, it is possible that drought damage will not appear when drought occurs. Loss of drought damage will not happen until much worse drought appears. The evaluation on agricultural drought risk was studied by many scholars at home and abroad in recent years. Because the effect of climate change on drought made wheat yield reducing, the maximal soil moisture was chosen as a index by Richter in 2005, and the risk evaluation on agricultural drought in England and Welsh was studied[3]. The evaluation index system on agricultural drought risk including standardized precipitation index (SPI), crop yield, irrigation area ratio, agricultural population etc. was set up by Sham in 2008[4], and it evaluated the agricultural drought risk in Bangladesh. The APSIM model combined with growing characteristics and water use efficiency of two eucalyptuses was studied by Huth etc. in Australia in 2008, and it also evaluated the drought risk on eucalyptus[5]. Based on the theory on the agricultural drought risk, considering the danger of agricultural drought disaster inducing factor and the vulnerability of hazard bearing bodies, the agricultural drought risk was analyzed by Cao Yongqiang in Dalian in 2011, and the index of agricultural drought risk was also calculated from 2000 to 2007. Then the comprehensive evaluation index system of agricultural drought risk was built on the basis of the results of drought risk index. Furthermore, the index weight was gotten with combination weighting method of deviation maximization, and the agricultural drought risk was comprehensively evaluated with variable fuzzy method in Dalian in 2002 [6]. Some scholars thought that China was a developing country with frequent and severe natural disasters [7]. With economy developing and population increasing, drought would make much more economic loss and much worse ecological environment, the same to Chinese social life, agricultural production. And drought damage became a severe problem affecting sustainable development of social economy, sustainable exploration and utilization of water resources and water environment protection in China. Furthermore, an index system on regional agricultural drought risk evaluation was set up based on social, economy and ecology Regional Agriculture Drought Risk Assessment 2297 etc. and a model on agricultural drought risk assessment was proposed depending on T-S fuzzy-neural network, and it was applied in Henan province so as to provide scientific proof for making regional agricultural anti-drought and making policy. 1 Agricultural drought risk evaluation model based on T-S fuzzy neural net 1.1 Model principle Takagi-Sugeno fuzzy system (T-S fuzzy system) composed of fuzzy system and artificial neural network is a readily adaptable fuzzy system, and its virtue is that subjection degree of fuzzy subset can constantly auto-update and auto-rectify. Because the input rule of T-S fuzzy neural network model is a linear relationship, T-S model has better mathematical expression input. As a result, the number of model input rules can be decreased farthest when multi-variable system is applied in model. It shows that T-S model has much more superiority than other fuzzy systems and artificial neutral model [8]. T-S fuzzy neural net was composed of the antecedent and subsequent net, and the antecedent net could march the predictor of fuzzy rules. The rule of ‘if-then’ is usually adapted in the input of T-S fuzzy neutral net model. And it is deduced in the iiii i rule of R :Ifx1122 isA , x isA ,..., xkk isA , in which xk 、 Aj are separately linguistic variable and fuzzy set. Subsequent net can make the subsequence of fuzzy rules. And =+ii +⋅⋅⋅+ i i it is deduced from antecedent rules a function, yppxikk011 px, in which R th i = is the i rule, p j (jk 1,2,..., ) is a parameter of fuzzy system, yi is an output from fuzzy rules. Generally, the net antecedence is the input, if, while the net subsequence is the output. Usually, the input is fuzzy, while the output is ensured. And there exists a linear relationship between them. The T-S fuzzy neural net model is composed of the input module, the fuzzy module, the fuzzy rule calculating module and output module [9]. (1)Input values are fuzzed by the subjection function with the fuzzy module, and μ = the fuzzy membership values can be gotten. It is for input quality, x [xx12 , ..., xk ] , the membership value of the input variables x j can be calculated on the basis of the fuzzy rules. And the fuzzy module adopts subjection function μΑiii = − −2 ) = = f jjjjexp( (xc ) / bi ( 1,2,..., kj ; 1,2,..., n ) (1.1) i i Where c j is the center of subjection function, bj the width of subjection function, k is the input parameter of the fuzzy system, n is the number of the fuzzy subset. (2) ω can be gotten with the fuzzy multiple multiplication function, when the module is calculated with the fuzzy rules. Each membership value can adopt the fuzzy calculation, in which the multiplication operator is replaced by the fuzzy operator ω=iiuA( x ) ∗ uA2 ( x ) ∗ ... ∗ uA k ( x ) ( i= 1,2,..., k ) (1.2) jj12 jk (3)The output of fuzzy neutral net can be gotten with functions in the output layer. 2298 Xu Zhengmin et al And the output value yi of fuzzy model can be done with the fuzzy results. nn =ωii + i +⋅⋅⋅+ i ω i yppxpxikk∑∑()/011 (1.3) ii==11 Error calculation: 1 eyy=−()2 (1.4) 2 dc Where yd , yc are the expected output and the actual output of model net, respectively. e is the error between the expected output and the actual output. Coefficient modification ∂e pkii()=−−α pk ( 1) (1.5) jj ∂ i p j ∂e n =−ωω()/y yxii ∂ i dc∑ j p j i=1 i α Where p j is the neutral internet coefficient, the net learning rate, x j the net input parameter, ωi the continued products of the input parameter membership value. Parameter modification: ∂e ckii()=−−β ck ( 1) (1.6) jj ∂ i c j ∂e bkii()=−−β bk ( 1) jj ∂ i bj i i Where c j is the center of the subjection function, bj the width of the subjection function, 1.2 Index system on agricultural drought risk assessment Drought is the risk factor of the drought risk, the drought accident is the drought damage in which the drought magnitude surpasses a certain threshold value, and the loss is the drought loss, provided that risk factor, risk accident and loss are all analyzed. All of them are corresponding separately with the danger of the drought accident, the vulnerability of bearing disaster environment and the draught loss, where the danger could be quantitatively described with the space and time scale, the magnitude and the intensity.
Details
-
File Typepdf
-
Upload Time-
-
Content LanguagesEnglish
-
Upload UserAnonymous/Not logged-in
-
File Pages14 Page
-
File Size-