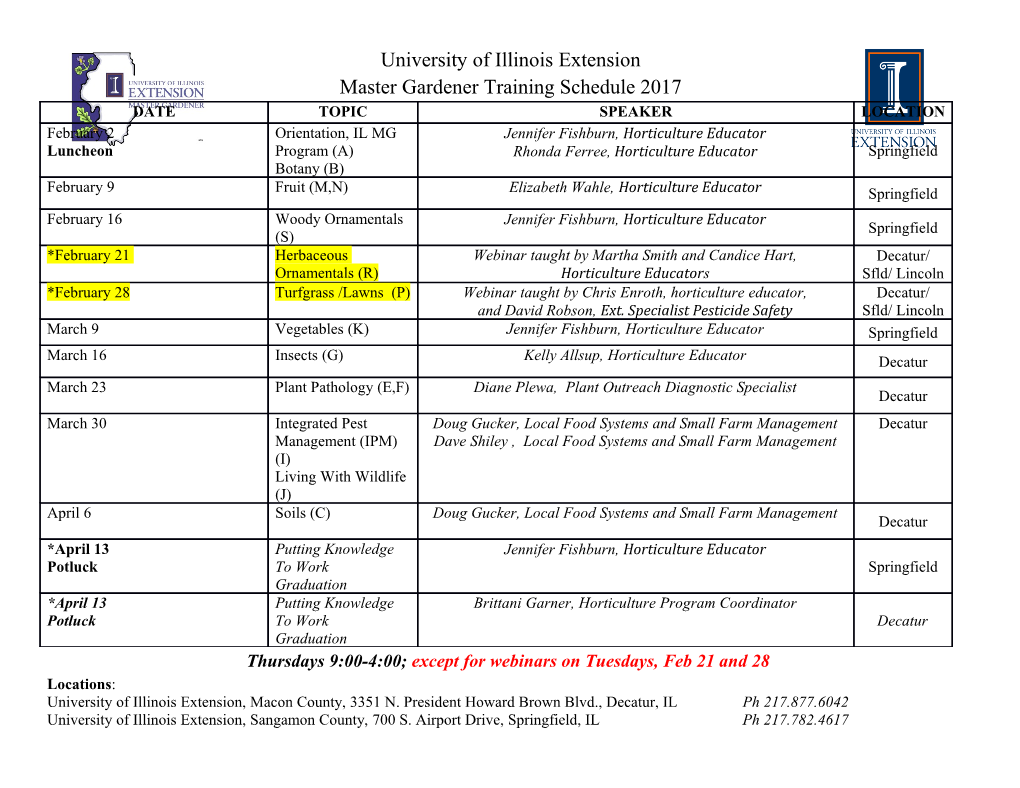
An Extended Semantics for Logic Programs with Annotated Disjunctions and its Efficient Implementation Fabrizio Riguzzi1 and Terrance Swift2 1 ENDIF – University of Ferrara Via Saragat 1, I-44122, Ferrara, Italy [email protected] 2 CENTRIA – Universidade Nova de Lisboa [email protected] Abstract. Logic Programming with Annotated Disjunctions (LPADs) is a for- malism for modeling probabilistic information that has recently received increased attention. The LPAD semantics, while being simple and clear, suffers from the re- quirement of having function free-programs, which is a strong limitation. In this paper we present an extension of the semantics that removes this restriction and allows us to write programs modeling infinite domains, such as Hidden Markov Models. We show that the semantics is well-defined for a large class of programs. Moreover, we present the algorithm “Probabilistic Inference with Tabling and Answer subsumption” (PITA) for computing the probability of queries to pro- grams according to the extended semantics. Tabling and answer subsumption not only ensure the correctness of the algorithm with respect to the semantics but also make it very efficient on programs without function symbols. PITA has been implemented in XSB and tested on six domains: two with func- tion symbols and four without. The execution times are compared with those of ProbLog, cplint and CVE. PITA was almost always able to solve larger prob- lems in a shorter time on both type of domains. 1 Introduction Many real world domains only can be represented effectively if we are able to model uncertainty. Recently, there has been an increased interest in logic languages represent- ing probabilistic information due to their succesful use in Machine Learning. Logic Programs with Annotated Disjunction (LPADs) [21] have attracted the atten- tion of various researchers due to their clarity, simplicity, modeling power and ability to model causation. Their semantics is an instance of the distribution semantics [17]: a theory defines a probability distribution over logic programs and the probability of a query is obtained by summing the probabilities of the programs where the query is true. The semantics of LPADs proposed in [21] requires the programs to be function-free, which is a strong requirement ruling out many interesting programs. Thus, we propose a version of the semantics that allows function symbols, along the lines of [17,12]. The new semantics is based on a program transformation technique that not only allows proving the correctness the semantics but also provides an efficient procedure for computing the probability of queries from LPADs. The algorithm “Probabilistic In- ference with Tabling and Answer subsumption” (PITA) builds explanations for every subgoal encountered during a derivation of the query. The explanations are compactly represented using Binary Decision Diagrams (BDDs) that also allow an efficient com- putation of the probability. Since all the explanations for a subgoal must be found, tabling is very useful for storing such information. Tabling has already been shown use- ful for probabilistic logic programming in [6,14,7]. PITA transforms the input LPAD into a normal logic programs in which the subgoals have an extra argument storing a BDD that represents the explanations for its answers. Moreover, we also exploit answer subsumption to combine explanations coming from different clauses. PITA draws inspiration from [5] that first proposed to use BDDs for computing the probability of queries for the Problog language, a minimalistic probabilistic extension of Prolog, and from [15] that applied BDDs to the more general LPAD syntax. Other approaches for reasoning on LPADs include [14], where SLG resolution is extended by repeatedly branching on disjunctive clauses, and [10], where CVE is presented that transforms an LPAD into an equivalent Bayesian network and then performs inference on the network using the variable elimination algorithm. PITA was tested on a number of datasets, both with and without function symbols, in order to evaluate its efficiency. The execution times of PITA were compared with those of cplint [15], CVE [10] and ProbLog [8]. PITA was able to successfully solve more complex queries than the other algorithms in most cases and it was also almost always faster both on datasets with and without function symbols. The paper is organized as follows. Section 2 illustrates the syntax and semantics of LPADs. Section 3 discusses the semantics of LPADs with function symbols. Section 4 gives an introduction to BDDs. Section 5 defines dynamic stratification for LPADs. Section 6 briefly recalls tabling and answer subsumption. Section 7 presents PITA and shows its correctness. Section 8 describes the experiments and Section 9 concludes the paper and presents directions for future works. 2 Logic Programs with Annotated Disjunctions A Logic Program with Annotated Disjunctions [21] consists of a finite set of annotated disjunctive clauses of the form h1 : α1 ∨ ... ∨ hn : αn ← b1,...,bm. In such a clause h1,...hn are logical atoms, b1,...,bm logical literals, and {α1,...,αn} real numbers n in the interval [0, 1] such that Pj=1 αj ≤ 1. h1 : α1 ∨ ... ∨ hn : αn is called the head and b1,...,bm is called the body. Note that if n = 1 and α1 = 1 a clause corresponds n to a normal program clause, sometimes called a non-disjunctive clause. If Pj=1 αj < 1, the head of the annotated disjunctive clause implicitly contains an extra atom null n that does not appear in the body of any clause and whose annotation is 1 − Pj=1 αj. For a clause C of the form above, we define head(C) as {(hi : αi)|1 ≤ i ≤ n} if n n Pi=1 αi = 1 and as {(hi : αi)|1 ≤ i ≤ n} ∪ {(null : 1 − Pi=1 αi)} otherwise. Moreover, we define body(C) as {bi|1 ≤ i ≤ m}, hi(C) as hi and αi(C) as αi. If the LPAD is ground, a clause represents a probabilistic choice between the non- disjunctive clauses obtained by selecting only one atom in the head. As usual, if the LPAD T is not ground, T can be assigned a meaning by computing its grounding, ground(T ). The semantics of LPADs, given in [21], requires the ground program to be finite, so the program must not contain function symbols if it contains variables. 2 By choosing a head atom for each ground clause of an LPAD we get a normal logic program called a possible world of the LPAD (called an instance of the LPAD in [21]). A probability distribution is defined over the space of possible worlds by assuming independence between the choices made for each clause. More specifically, an atomic choice is a triple (C,θ,i) where C ∈ T , θ is a substi- tution that grounds C and i ∈ {1,..., |head(C)|}. (C,θ,i) means that, for ground clause Cθ, the head hi(C) was chosen. A set of atomic choices κ is consistent if (C,θ,i) ∈ κ, (C,θ,j) ∈ κ ⇒ i = j, i.e., only one head is selected for a ground clause. A composite choice κ is a consistent set of atomic choices. The probability P (κ) of a composite choice κ is the product of the probabilities of the individual atomic choices, i.e. P (κ)= Q(C,θ,i)∈κ αi(C). A selection σ is a composite choice that, for each clause Cθ in ground(T ), con- tains an atomic choice (C,θ,i) in σ. We denote the set of all selections σ of a pro- gram T by ST . A selection σ identifies a normal logic program wσ defined as follows wσ = {(hi(C)θ ← body(C))θ|(C,θ,i) ∈ σ}. wσ is called a possible world (or simply world) of T . WT denotes the set of all the possible worlds of T . Since selections are composite choices, we can assign a probability to possible worlds: P (wσ) = P (σ) = Q(C,θ,i)∈σ αi(C). We consider only sound LPADs, in which every possible world has a total model according to the Well-Founded Semantics (WFS) [20]. In this way, the uncertainty is modeled only by means of the disjunctions in the head and not by the features of the semantics. In the following, wσ |= φ means that the atom φ is true in the well-founded model of the program wσ. The probability of a ground atom φ according to an LPAD T is given by the sum of the probabilities of the possible worlds where the atom is true under the WFS: P (φ)= P P (σ). It is easy to see that P satisfies the axioms of probability. σ∈ST ,wσ |=φ Example 1. Consider the dependency of sneezing on having the flu or hay fever: C1 = strong sneezing(X):0.3 ∨ moderate sneezing(X):0.5 ← flu(X). C2 = strong sneezing(X):0.2 ∨ moderate sneezing(X):0.6 ← hay fever(X). C3 = flu(david). C4 = hay fever(david). This program models the fact that sneezing can be caused by flu or hay fever. The query strong sneezing(david) is true in 5 of the 9 instances of the program and its probability is PT (strong sneezing(david))=0.3·0.2+0.3·0.6+0.3·0.2+0.5·0.2+0.2·0.2 = 0.44 Even if we assumed independence between the choices for individual ground clauses, this does not represents a restriction, in the sense that this still allow to represent all the joint distributions of atoms of the Herbrand base that are representable with a Bayesian network over those variables. Details of the proof are omitted for lack of space. LPADs can be written by the user or learned from data. When written by the user, the best approach is to write each clause so that it models a causal mechanism of the domain and to choose the parameters on the basis of his knowledge of the mechanism.
Details
-
File Typepdf
-
Upload Time-
-
Content LanguagesEnglish
-
Upload UserAnonymous/Not logged-in
-
File Pages15 Page
-
File Size-